Smartphone app for non-invasive detection of anemia using only patient-sourced photos
- PMID: 30514831
- PMCID: PMC6279826
- DOI: 10.1038/s41467-018-07262-2
Smartphone app for non-invasive detection of anemia using only patient-sourced photos
Abstract
We introduce a paradigm of completely non-invasive, on-demand diagnostics that may replace common blood-based laboratory tests using only a smartphone app and photos. We initially targeted anemia, a blood condition characterized by low blood hemoglobin levels that afflicts >2 billion people. Our app estimates hemoglobin levels by analyzing color and metadata of fingernail bed smartphone photos and detects anemia (hemoglobin levels <12.5 g dL-1) with an accuracy of ±2.4 g dL-1 and a sensitivity of 97% (95% CI, 89-100%) when compared with CBC hemoglobin levels (n = 100 subjects), indicating its viability to serve as a non-invasive anemia screening tool. Moreover, with personalized calibration, this system achieves an accuracy of ±0.92 g dL-1 of CBC hemoglobin levels (n = 16), empowering chronic anemia patients to serially monitor their hemoglobin levels instantaneously and remotely. Our on-demand system enables anyone with a smartphone to download an app and immediately detect anemia anywhere and anytime.
Conflict of interest statement
The authors declare no competing interests.
Figures
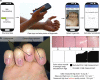
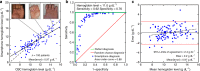
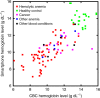
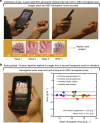
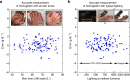
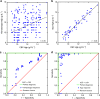
Similar articles
-
Smartphone-based point-of-care anemia screening in rural Bihar in India.Commun Med (Lond). 2023 Mar 22;3(1):38. doi: 10.1038/s43856-023-00267-z. Commun Med (Lond). 2023. PMID: 36949164 Free PMC article.
-
Prediction of anemia in real-time using a smartphone camera processing conjunctival images.PLoS One. 2024 May 13;19(5):e0302883. doi: 10.1371/journal.pone.0302883. eCollection 2024. PLoS One. 2024. PMID: 38739605 Free PMC article.
-
Prediction of anemia and estimation of hemoglobin concentration using a smartphone camera.PLoS One. 2021 Jul 14;16(7):e0253495. doi: 10.1371/journal.pone.0253495. eCollection 2021. PLoS One. 2021. PMID: 34260592 Free PMC article.
-
[Review by expert group in the diagnosis and treatment of anemia in pregnant women. Federación Mexicana de Colegios de Obstetricia y Ginecología].Ginecol Obstet Mex. 2012 Sep;80(9):563-80. Ginecol Obstet Mex. 2012. PMID: 23243836 Spanish.
-
Characteristics of Men Who Have Sex With Men Who Use Smartphone Geosocial Networking Applications and Implications for HIV Interventions: A Systematic Review and Meta-Analysis.Arch Sex Behav. 2017 May;46(4):885-894. doi: 10.1007/s10508-016-0709-3. Epub 2016 Apr 4. Arch Sex Behav. 2017. PMID: 27043837 Review.
Cited by
-
Anemia detection through non-invasive analysis of lip mucosa images.Front Big Data. 2023 Oct 19;6:1241899. doi: 10.3389/fdata.2023.1241899. eCollection 2023. Front Big Data. 2023. PMID: 37928177 Free PMC article.
-
Personalized anesthesia and precision medicine: a comprehensive review of genetic factors, artificial intelligence, and patient-specific factors.Front Med (Lausanne). 2024 May 9;11:1365524. doi: 10.3389/fmed.2024.1365524. eCollection 2024. Front Med (Lausanne). 2024. PMID: 38784235 Free PMC article. Review.
-
Non-invasive detection of anemia using lip mucosa images transfer learning convolutional neural networks.Front Big Data. 2023 Nov 3;6:1291329. doi: 10.3389/fdata.2023.1291329. eCollection 2023. Front Big Data. 2023. PMID: 38025943 Free PMC article.
-
Relationship between Selected Functional Performance Parameters and the Occurrence of Anaemia in Hospitalized Females and Males Aged 80 and More.Int J Environ Res Public Health. 2022 Oct 13;19(20):13179. doi: 10.3390/ijerph192013179. Int J Environ Res Public Health. 2022. PMID: 36293764 Free PMC article.
-
Diagnostic Validation and Feasibility of a Non-invasive Haemoglobin Screening Device (EzeCheck) for 'Anaemia Mukt Bharat' in India.Cureus. 2024 Jan 24;16(1):e52877. doi: 10.7759/cureus.52877. eCollection 2024 Jan. Cureus. 2024. PMID: 38406104 Free PMC article.
References
-
- Rappaport Kathryn M., McCracken Courtney C., Beniflah Jacob, Little Wendalyn K., Fletcher Daniel A., Lam Wilbur A., Shane Andi L. Assessment of a Smartphone Otoscope Device for the Diagnosis and Management of Otitis Media. Clinical Pediatrics. 2016;55(9):800–810. doi: 10.1177/0009922815593909. - DOI - PubMed
Publication types
MeSH terms
Substances
Grants and funding
LinkOut - more resources
Full Text Sources
Other Literature Sources
Medical