In vitro neural networks minimise variational free energy
- PMID: 30446766
- PMCID: PMC6240088
- DOI: 10.1038/s41598-018-35221-w
In vitro neural networks minimise variational free energy
Abstract
In this work, we address the neuronal encoding problem from a Bayesian perspective. Specifically, we ask whether neuronal responses in an in vitro neuronal network are consistent with ideal Bayesian observer responses under the free energy principle. In brief, we stimulated an in vitro cortical cell culture with stimulus trains that had a known statistical structure. We then asked whether recorded neuronal responses were consistent with variational message passing based upon free energy minimisation (i.e., evidence maximisation). Effectively, this required us to solve two problems: first, we had to formulate the Bayes-optimal encoding of the causes or sources of sensory stimulation, and then show that these idealised responses could account for observed electrophysiological responses. We describe a simulation of an optimal neural network (i.e., the ideal Bayesian neural code) and then consider the mapping from idealised in silico responses to recorded in vitro responses. Our objective was to find evidence for functional specialisation and segregation in the in vitro neural network that reproduced in silico learning via free energy minimisation. Finally, we combined the in vitro and in silico results to characterise learning in terms of trajectories in a variational information plane of accuracy and complexity.
Conflict of interest statement
The authors declare no competing interests.
Figures
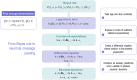
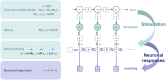
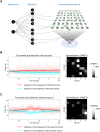
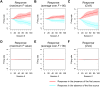
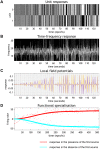
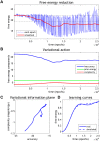
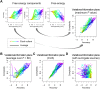
Similar articles
-
Canonical neural networks perform active inference.Commun Biol. 2022 Jan 14;5(1):55. doi: 10.1038/s42003-021-02994-2. Commun Biol. 2022. PMID: 35031656 Free PMC article.
-
Comparing variational Bayes with Markov chain Monte Carlo for Bayesian computation in neuroimaging.Stat Methods Med Res. 2013 Aug;22(4):398-423. doi: 10.1177/0962280212448973. Epub 2012 May 28. Stat Methods Med Res. 2013. PMID: 22642986
-
Stochastic complexities of general mixture models in variational Bayesian learning.Neural Netw. 2007 Mar;20(2):210-9. doi: 10.1016/j.neunet.2006.05.030. Epub 2006 Aug 10. Neural Netw. 2007. PMID: 16904288
-
Active inference leads to Bayesian neurophysiology.Neurosci Res. 2022 Feb;175:38-45. doi: 10.1016/j.neures.2021.12.003. Epub 2021 Dec 27. Neurosci Res. 2022. PMID: 34968557 Review.
-
How do high-level specifications of the brain relate to variational approaches?J Physiol Paris. 2007 Jan-May;101(1-3):118-35. doi: 10.1016/j.jphysparis.2007.10.010. Epub 2007 Oct 26. J Physiol Paris. 2007. PMID: 18035526 Review.
Cited by
-
Nanomagnetic Guidance Shapes the Structure-Function Relationship of Developing Cortical Networks.Nano Lett. 2024 Oct 30;24(43):13564-13573. doi: 10.1021/acs.nanolett.4c03156. Epub 2024 Oct 21. Nano Lett. 2024. PMID: 39432086
-
Message Passing and Metabolism.Entropy (Basel). 2021 May 14;23(5):606. doi: 10.3390/e23050606. Entropy (Basel). 2021. PMID: 34068913 Free PMC article.
-
Bayesian mechanics of perceptual inference and motor control in the brain.Biol Cybern. 2021 Feb;115(1):87-102. doi: 10.1007/s00422-021-00859-9. Epub 2021 Jan 20. Biol Cybern. 2021. PMID: 33471182 Free PMC article.
-
The emergence of synchrony in networks of mutually inferring neurons.Sci Rep. 2019 Apr 30;9(1):6412. doi: 10.1038/s41598-019-42821-7. Sci Rep. 2019. PMID: 31040386 Free PMC article.
-
Canonical neural networks perform active inference.Commun Biol. 2022 Jan 14;5(1):55. doi: 10.1038/s42003-021-02994-2. Commun Biol. 2022. PMID: 35031656 Free PMC article.
References
-
- von Helmholtz, H. Treatise on physiological optics (Vol. 3) (The Optical Society of America, 1925).
Publication types
MeSH terms
Grants and funding
LinkOut - more resources
Full Text Sources