Fully Automated Echocardiogram Interpretation in Clinical Practice
- PMID: 30354459
- PMCID: PMC6200386
- DOI: 10.1161/CIRCULATIONAHA.118.034338
Fully Automated Echocardiogram Interpretation in Clinical Practice
Abstract
Background: Automated cardiac image interpretation has the potential to transform clinical practice in multiple ways, including enabling serial assessment of cardiac function by nonexperts in primary care and rural settings. We hypothesized that advances in computer vision could enable building a fully automated, scalable analysis pipeline for echocardiogram interpretation, including (1) view identification, (2) image segmentation, (3) quantification of structure and function, and (4) disease detection.
Methods: Using 14 035 echocardiograms spanning a 10-year period, we trained and evaluated convolutional neural network models for multiple tasks, including automated identification of 23 viewpoints and segmentation of cardiac chambers across 5 common views. The segmentation output was used to quantify chamber volumes and left ventricular mass, determine ejection fraction, and facilitate automated determination of longitudinal strain through speckle tracking. Results were evaluated through comparison to manual segmentation and measurements from 8666 echocardiograms obtained during the routine clinical workflow. Finally, we developed models to detect 3 diseases: hypertrophic cardiomyopathy, cardiac amyloid, and pulmonary arterial hypertension.
Results: Convolutional neural networks accurately identified views (eg, 96% for parasternal long axis), including flagging partially obscured cardiac chambers, and enabled the segmentation of individual cardiac chambers. The resulting cardiac structure measurements agreed with study report values (eg, median absolute deviations of 15% to 17% of observed values for left ventricular mass, left ventricular diastolic volume, and left atrial volume). In terms of function, we computed automated ejection fraction and longitudinal strain measurements (within 2 cohorts), which agreed with commercial software-derived values (for ejection fraction, median absolute deviation=9.7% of observed, N=6407 studies; for strain, median absolute deviation=7.5%, n=419, and 9.0%, n=110) and demonstrated applicability to serial monitoring of patients with breast cancer for trastuzumab cardiotoxicity. Overall, we found automated measurements to be comparable or superior to manual measurements across 11 internal consistency metrics (eg, the correlation of left atrial and ventricular volumes). Finally, we trained convolutional neural networks to detect hypertrophic cardiomyopathy, cardiac amyloidosis, and pulmonary arterial hypertension with C statistics of 0.93, 0.87, and 0.85, respectively.
Conclusions: Our pipeline lays the groundwork for using automated interpretation to support serial patient tracking and scalable analysis of millions of echocardiograms archived within healthcare systems.
Keywords: diagnosis; echocardiography; machine learning.
Figures
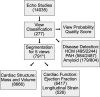
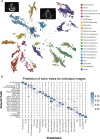
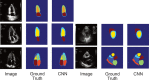
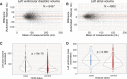
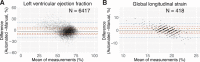
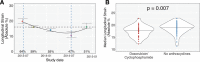
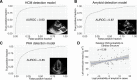
Comment in
-
Learning About Machine Learning to Create a Self-Driving Echocardiographic Laboratory.Circulation. 2018 Oct 16;138(16):1636-1638. doi: 10.1161/CIRCULATIONAHA.118.037094. Circulation. 2018. PMID: 30354471 No abstract available.
-
Will Artificial Intelligence Replace the Human Echocardiographer?Circulation. 2018 Oct 16;138(16):1639-1642. doi: 10.1161/CIRCULATIONAHA.118.037095. Circulation. 2018. PMID: 30354473 Free PMC article. No abstract available.
-
Response by Zhang and Deo to Letter Regarding Article, "Fully Automated Echocardiogram Interpretation in Clinical Practice: Feasibility and Diagnostic Accuracy".Circulation. 2019 Mar 26;139(13):1648-1649. doi: 10.1161/CIRCULATIONAHA.119.039291. Circulation. 2019. PMID: 30908098 No abstract available.
-
Letter by Goto and Goto Regarding Article, "Fully Automated Echocardiogram Interpretation in Clinical Practice: Feasibility and Diagnostic Accuracy".Circulation. 2019 Mar 26;139(13):1646-1647. doi: 10.1161/CIRCULATIONAHA.118.038451. Circulation. 2019. PMID: 30908106 No abstract available.
Similar articles
-
Automated cardiovascular magnetic resonance image analysis with fully convolutional networks.J Cardiovasc Magn Reson. 2018 Sep 14;20(1):65. doi: 10.1186/s12968-018-0471-x. J Cardiovasc Magn Reson. 2018. PMID: 30217194 Free PMC article.
-
Transthoracic 3D Echocardiographic Left Heart Chamber Quantification Using an Automated Adaptive Analytics Algorithm.JACC Cardiovasc Imaging. 2016 Jul;9(7):769-782. doi: 10.1016/j.jcmg.2015.12.020. Epub 2016 Jun 15. JACC Cardiovasc Imaging. 2016. PMID: 27318718
-
Fully Automated, Quality-Controlled Cardiac Analysis From CMR: Validation and Large-Scale Application to Characterize Cardiac Function.JACC Cardiovasc Imaging. 2020 Mar;13(3):684-695. doi: 10.1016/j.jcmg.2019.05.030. Epub 2019 Jul 17. JACC Cardiovasc Imaging. 2020. PMID: 31326477 Free PMC article.
-
Automated Quantification in Echocardiography.JACC Cardiovasc Imaging. 2019 Jun;12(6):1073-1092. doi: 10.1016/j.jcmg.2018.11.038. JACC Cardiovasc Imaging. 2019. PMID: 31171260 Review.
-
Deep learning supported echocardiogram analysis: A comprehensive review.Artif Intell Med. 2024 May;151:102866. doi: 10.1016/j.artmed.2024.102866. Epub 2024 Apr 4. Artif Intell Med. 2024. PMID: 38593684 Review.
Cited by
-
Physicians and Machine-Learning Algorithm Performance in Predicting Left-Ventricular Systolic Dysfunction from a Standard 12-Lead-Electrocardiogram.J Clin Med. 2022 Nov 15;11(22):6767. doi: 10.3390/jcm11226767. J Clin Med. 2022. PMID: 36431244 Free PMC article.
-
World Heart Federation Roadmap for Digital Health in Cardiology.Glob Heart. 2022 Aug 26;17(1):61. doi: 10.5334/gh.1141. eCollection 2022. Glob Heart. 2022. PMID: 36051317 Free PMC article.
-
Artificial Intelligence in the Screening, Diagnosis, and Management of Aortic Stenosis.Rev Cardiovasc Med. 2024 Jan 17;25(1):31. doi: 10.31083/j.rcm2501031. eCollection 2024 Jan. Rev Cardiovasc Med. 2024. PMID: 39077660 Free PMC article. Review.
-
Echocardiography-based AI detection of regional wall motion abnormalities and quantification of cardiac function in myocardial infarction.Front Cardiovasc Med. 2022 Aug 22;9:903660. doi: 10.3389/fcvm.2022.903660. eCollection 2022. Front Cardiovasc Med. 2022. PMID: 36072864 Free PMC article.
-
Standard Echocardiographic View Recognition in Diagnosis of Congenital Heart Defects in Children Using Deep Learning Based on Knowledge Distillation.Front Pediatr. 2022 Jan 18;9:770182. doi: 10.3389/fped.2021.770182. eCollection 2021. Front Pediatr. 2022. PMID: 35118028 Free PMC article.
References
-
- Hill JA, Olson EN. Cardiac plasticity. N Engl J Med. 2008;358:1370–1380. doi: 10.1056/NEJMra072139. - PubMed
-
- Ishizu T, Seo Y, Kameda Y, Kawamura R, Kimura T, Shimojo N, Xu D, Murakoshi N, Aonuma K. Left ventricular strain and transmural distribution of structural remodeling in hypertensive heart disease. Hypertension. 2014;63:500–506. doi: 10.1161/HYPERTENSIONAHA.113.02149. - PubMed
-
- Neskovic AN, Edvardsen T, Galderisi M, Garbi M, Gullace G, Jurcut R, Dalen H, Hagendorff A, Lancellotti P, Popescu BA, Sicari R, Stefanidis A European Association of Cardiovascular Imaging Document Reviewers: Focus cardiac ultrasound: the European Association of Cardiovascular Imaging viewpoint. Eur Heart J Cardiovasc Imaging. 2014;15:956–960. doi: 10.1093/ehjci/jeu081. - PubMed
-
- Andrus BW, Welch HG. Medicare services provided by cardiologists in the United States: 1999-2008. Circ Cardiovasc Qual Outcomes. 2012;5:31–36. doi: 10.1161/CIRCOUTCOMES.111.961813. - PubMed
-
- Szeliski R. Computer Vision: Algorithms and Applications. London: Springer-Verlag;; 2011.
Publication types
MeSH terms
Grants and funding
LinkOut - more resources
Full Text Sources
Other Literature Sources
Medical