Theoretical Models of Neural Development
- PMID: 30321813
- PMCID: PMC6197653
- DOI: 10.1016/j.isci.2018.09.017
Theoretical Models of Neural Development
Abstract
Constructing a functioning nervous system requires the precise orchestration of a vast array of mechanical, molecular, and neural-activity-dependent cues. Theoretical models can play a vital role in helping to frame quantitative issues, reveal mathematical commonalities between apparently diverse systems, identify what is and what is not possible in principle, and test the abilities of specific mechanisms to explain the data. This review focuses on the progress that has been made over the last decade in our theoretical understanding of neural development.
Keywords: Biological Sciences; Developmental Neuroscience; In Silico Biology; Neuroscience; Theoretical Neuroscience.
Copyright © 2018 The Author(s). Published by Elsevier Inc. All rights reserved.
Figures
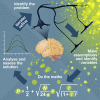
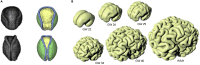
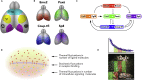
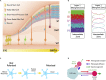
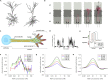
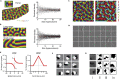
Similar articles
-
Behavioral Neuroscience of Zebrafish.In: Buccafusco JJ, editor. Methods of Behavior Analysis in Neuroscience. 2nd edition. Boca Raton (FL): CRC Press/Taylor & Francis; 2009. Chapter 15. In: Buccafusco JJ, editor. Methods of Behavior Analysis in Neuroscience. 2nd edition. Boca Raton (FL): CRC Press/Taylor & Francis; 2009. Chapter 15. PMID: 21204325 Free Books & Documents. Review.
-
SysBioMed report: advancing systems biology for medical applications.IET Syst Biol. 2009 May;3(3):131-6. doi: 10.1049/iet-syb.2009.0005. IET Syst Biol. 2009. PMID: 19449974
-
Macromolecular crowding: chemistry and physics meet biology (Ascona, Switzerland, 10-14 June 2012).Phys Biol. 2013 Aug;10(4):040301. doi: 10.1088/1478-3975/10/4/040301. Epub 2013 Aug 2. Phys Biol. 2013. PMID: 23912807
-
Enrichment Effects on Adult Cognitive Development: Can the Functional Capacity of Older Adults Be Preserved and Enhanced?Psychol Sci Public Interest. 2008 Oct;9(1):1-65. doi: 10.1111/j.1539-6053.2009.01034.x. Epub 2008 Oct 1. Psychol Sci Public Interest. 2008. PMID: 26162004
-
Toward a computational framework for cognitive biology: unifying approaches from cognitive neuroscience and comparative cognition.Phys Life Rev. 2014 Sep;11(3):329-64. doi: 10.1016/j.plrev.2014.04.005. Epub 2014 May 13. Phys Life Rev. 2014. PMID: 24969660 Review.
Cited by
-
Technique Advances in Enteroatmospheric Fistula Isolation After Open Abdomen: A Review and Outlook.Front Surg. 2021 Jan 20;7:559443. doi: 10.3389/fsurg.2020.559443. eCollection 2020. Front Surg. 2021. PMID: 33553237 Free PMC article. Review.
-
On the Role of LGN/V1 Spontaneous Activity as an Innate Learning Pattern for Visual Development.Front Physiol. 2021 Oct 27;12:695431. doi: 10.3389/fphys.2021.695431. eCollection 2021. Front Physiol. 2021. PMID: 34776991 Free PMC article.
-
Mathematical models of neuronal growth.Biomech Model Mechanobiol. 2022 Feb;21(1):89-118. doi: 10.1007/s10237-021-01539-0. Epub 2022 Jan 7. Biomech Model Mechanobiol. 2022. PMID: 34994872 Free PMC article. Review.
-
Deciphering the topological landscape of glioma using a network theory framework.Sci Rep. 2024 Nov 5;14(1):26724. doi: 10.1038/s41598-024-77856-y. Sci Rep. 2024. PMID: 39496747 Free PMC article.
-
Achieving functional neuronal dendrite structure through sequential stochastic growth and retraction.Elife. 2020 Nov 26;9:e60920. doi: 10.7554/eLife.60920. Elife. 2020. PMID: 33241995 Free PMC article.
References
Publication types
LinkOut - more resources
Full Text Sources