Free-energy minimization in joint agent-environment systems: A niche construction perspective
- PMID: 30012517
- PMCID: PMC6117456
- DOI: 10.1016/j.jtbi.2018.07.002
Free-energy minimization in joint agent-environment systems: A niche construction perspective
Abstract
The free-energy principle is an attempt to explain the structure of the agent and its brain, starting from the fact that an agent exists (Friston and Stephan, 2007; Friston et al., 2010). More specifically, it can be regarded as a systematic attempt to understand the 'fit' between an embodied agent and its niche, where the quantity of free-energy is a measure for the 'misfit' or disattunement (Bruineberg and Rietveld, 2014) between agent and environment. This paper offers a proof-of-principle simulation of niche construction under the free-energy principle. Agent-centered treatments have so far failed to address situations where environments change alongside agents, often due to the action of agents themselves. The key point of this paper is that the minimum of free-energy is not at a point in which the agent is maximally adapted to the statistics of a static environment, but can better be conceptualized an attracting manifold within the joint agent-environment state-space as a whole, which the system tends toward through mutual interaction. We will provide a general introduction to active inference and the free-energy principle. Using Markov Decision Processes (MDPs), we then describe a canonical generative model and the ensuing update equations that minimize free-energy. We then apply these equations to simulations of foraging in an environment; in which an agent learns the most efficient path to a pre-specified location. In some of those simulations, unbeknownst to the agent, the 'desire paths' emerge as a function of the activity of the agent (i.e. niche construction occurs). We will show how, depending on the relative inertia of the environment and agent, the joint agent-environment system moves to different attracting sets of jointly minimized free-energy.
Keywords: Active inference; Adaptive environments; Agent-environment complementarity; Desire paths; Free energy principle; Markov decision processes; Niche construction.
Copyright © 2018 The Author(s). Published by Elsevier Ltd.. All rights reserved.
Figures
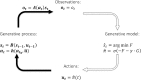
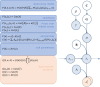
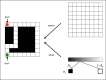
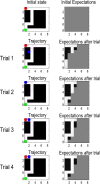
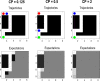
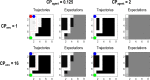
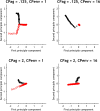
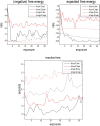
Similar articles
-
Action and behavior: a free-energy formulation.Biol Cybern. 2010 Mar;102(3):227-60. doi: 10.1007/s00422-010-0364-z. Epub 2010 Feb 11. Biol Cybern. 2010. PMID: 20148260
-
Toward an Embodied, Embedded Predictive Processing Account.Front Psychol. 2021 Jan 29;12:543076. doi: 10.3389/fpsyg.2021.543076. eCollection 2021. Front Psychol. 2021. PMID: 33584461 Free PMC article.
-
A free energy principle for the brain.J Physiol Paris. 2006 Jul-Sep;100(1-3):70-87. doi: 10.1016/j.jphysparis.2006.10.001. Epub 2006 Nov 13. J Physiol Paris. 2006. PMID: 17097864 Review.
-
Bayesian mechanics of perceptual inference and motor control in the brain.Biol Cybern. 2021 Feb;115(1):87-102. doi: 10.1007/s00422-021-00859-9. Epub 2021 Jan 20. Biol Cybern. 2021. PMID: 33471182 Free PMC article.
-
Active inference leads to Bayesian neurophysiology.Neurosci Res. 2022 Feb;175:38-45. doi: 10.1016/j.neures.2021.12.003. Epub 2021 Dec 27. Neurosci Res. 2022. PMID: 34968557 Review.
Cited by
-
Variational ecology and the physics of sentient systems.Phys Life Rev. 2019 Dec;31:188-205. doi: 10.1016/j.plrev.2018.12.002. Epub 2019 Jan 7. Phys Life Rev. 2019. PMID: 30655223 Free PMC article. Review.
-
Prefrontal Computation as Active Inference.Cereb Cortex. 2020 Mar 21;30(2):682-695. doi: 10.1093/cercor/bhz118. Cereb Cortex. 2020. PMID: 31298270 Free PMC article.
-
Federated inference and belief sharing.Neurosci Biobehav Rev. 2024 Jan;156:105500. doi: 10.1016/j.neubiorev.2023.105500. Epub 2023 Dec 5. Neurosci Biobehav Rev. 2024. PMID: 38056542 Free PMC article. Review.
-
Regimes of Expectations: An Active Inference Model of Social Conformity and Human Decision Making.Front Psychol. 2019 Mar 29;10:679. doi: 10.3389/fpsyg.2019.00679. eCollection 2019. Front Psychol. 2019. PMID: 30988668 Free PMC article.
-
A World Unto Itself: Human Communication as Active Inference.Front Psychol. 2020 Mar 25;11:417. doi: 10.3389/fpsyg.2020.00417. eCollection 2020. Front Psychol. 2020. PMID: 32269536 Free PMC article.
References
-
- Ball K., Sekuler R. A specific and enduring improvement in visual motion discrimination. Science. 1982;218:697–698. - PubMed
-
- Baltieri M., Buckley C.L. Proc. Eur. Conf. on Artificial Life. 2017. An active inference implementation of phototaxis; pp. 36–43.
-
- Beal M.J. Doctoral Dissertation. University College; London: 2003. Variational algorithms for approximate Bayesian inference.
Publication types
MeSH terms
Grants and funding
LinkOut - more resources
Full Text Sources
Other Literature Sources