Computational Principles of Supervised Learning in the Cerebellum
- PMID: 29986160
- PMCID: PMC6056176
- DOI: 10.1146/annurev-neuro-080317-061948
Computational Principles of Supervised Learning in the Cerebellum
Abstract
Supervised learning plays a key role in the operation of many biological and artificial neural networks. Analysis of the computations underlying supervised learning is facilitated by the relatively simple and uniform architecture of the cerebellum, a brain area that supports numerous motor, sensory, and cognitive functions. We highlight recent discoveries indicating that the cerebellum implements supervised learning using the following organizational principles: ( a) extensive preprocessing of input representations (i.e., feature engineering), ( b) massively recurrent circuit architecture, ( c) linear input-output computations, ( d) sophisticated instructive signals that can be regulated and are predictive, ( e) adaptive mechanisms of plasticity with multiple timescales, and ( f) task-specific hardware specializations. The principles emerging from studies of the cerebellum have striking parallels with those in other brain areas and in artificial neural networks, as well as some notable differences, which can inform future research on supervised learning and inspire next-generation machine-based algorithms.
Keywords: Purkinje cell; climbing fiber; consolidation; decorrelation; machine learning; plasticity.
Figures
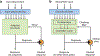
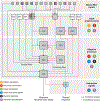
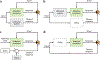
Similar articles
-
Synaptic mechanisms of sensorimotor learning in the cerebellum.Curr Opin Neurobiol. 2011 Aug;21(4):609-15. doi: 10.1016/j.conb.2011.06.011. Epub 2011 Jul 20. Curr Opin Neurobiol. 2011. PMID: 21767944 Review.
-
Adaptive-filter models of the cerebellum: computational analysis.Cerebellum. 2008;7(4):567-71. doi: 10.1007/s12311-008-0067-3. Cerebellum. 2008. PMID: 18972182
-
Decorrelation learning in the cerebellum: computational analysis and experimental questions.Prog Brain Res. 2014;210:157-92. doi: 10.1016/B978-0-444-63356-9.00007-8. Prog Brain Res. 2014. PMID: 24916293 Review.
-
Principles of operation of a cerebellar learning circuit.Elife. 2020 Apr 30;9:e55217. doi: 10.7554/eLife.55217. Elife. 2020. PMID: 32352914 Free PMC article.
-
Embarrassed, but not depressed: eye opening lessons for cerebellar learning.Neuron. 2002 Jul 18;35(2):223-6. doi: 10.1016/s0896-6273(02)00771-7. Neuron. 2002. PMID: 12160741 Review.
Cited by
-
Cerebellar Contributions to the Basal Ganglia Influence Motor Coordination, Reward Processing, and Movement Vigor.J Neurosci. 2022 Nov 9;42(45):8406-8415. doi: 10.1523/JNEUROSCI.1535-22.2022. J Neurosci. 2022. PMID: 36351826 Free PMC article. Review.
-
Systemic pharmacological suppression of neural activity reverses learning impairment in a mouse model of Fragile X syndrome.Elife. 2024 Jul 2;12:RP92543. doi: 10.7554/eLife.92543. Elife. 2024. PMID: 38953282 Free PMC article.
-
Eye Movement Disorders and the Cerebellum.J Clin Neurophysiol. 2019 Nov;36(6):405-414. doi: 10.1097/WNP.0000000000000579. J Clin Neurophysiol. 2019. PMID: 31688323 Free PMC article. Review.
-
Gating by Memory: a Theory of Learning in the Cerebellum.Cerebellum. 2022 Dec;21(6):926-943. doi: 10.1007/s12311-021-01325-9. Epub 2021 Nov 10. Cerebellum. 2022. PMID: 34757585 Free PMC article.
-
Application of Artificial Intelligence and Machine Learning in Drug Discovery.Methods Mol Biol. 2022;2390:113-124. doi: 10.1007/978-1-0716-1787-8_4. Methods Mol Biol. 2022. PMID: 34731466
References
-
- Albus JS. 1971. A theory of cerebellar function. Math. Biosci 10:25–61
-
- Alpaydin E 2014. Introduction to Machine Learning Cambridge, MA: MIT Press
-
- Apps R 2000. Gating of climbing fibre input to cerebellar cortical zones. Prog. Brain Res 124:201–11 - PubMed
Publication types
MeSH terms
Grants and funding
LinkOut - more resources
Full Text Sources
Other Literature Sources