Efficient and self-adaptive in-situ learning in multilayer memristor neural networks
- PMID: 29921923
- PMCID: PMC6008303
- DOI: 10.1038/s41467-018-04484-2
Efficient and self-adaptive in-situ learning in multilayer memristor neural networks
Abstract
Memristors with tunable resistance states are emerging building blocks of artificial neural networks. However, in situ learning on a large-scale multiple-layer memristor network has yet to be demonstrated because of challenges in device property engineering and circuit integration. Here we monolithically integrate hafnium oxide-based memristors with a foundry-made transistor array into a multiple-layer neural network. We experimentally demonstrate in situ learning capability and achieve competitive classification accuracy on a standard machine learning dataset, which further confirms that the training algorithm allows the network to adapt to hardware imperfections. Our simulation using the experimental parameters suggests that a larger network would further increase the classification accuracy. The memristor neural network is a promising hardware platform for artificial intelligence with high speed-energy efficiency.
Conflict of interest statement
The authors declare no competing interests.
Figures
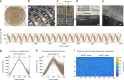
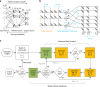
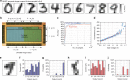
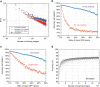
Similar articles
-
Thousands of conductance levels in memristors integrated on CMOS.Nature. 2023 Mar;615(7954):823-829. doi: 10.1038/s41586-023-05759-5. Epub 2023 Mar 29. Nature. 2023. PMID: 36991190
-
A Learning-Rate Modulable and Reliable TiOx Memristor Array for Robust, Fast, and Accurate Neuromorphic Computing.Adv Sci (Weinh). 2022 Aug;9(22):e2201117. doi: 10.1002/advs.202201117. Epub 2022 Jun 5. Adv Sci (Weinh). 2022. PMID: 35666073 Free PMC article.
-
Training memristor-based multilayer neuromorphic networks with SGD, momentum and adaptive learning rates.Neural Netw. 2020 Aug;128:142-149. doi: 10.1016/j.neunet.2020.04.025. Epub 2020 May 7. Neural Netw. 2020. PMID: 32446191
-
Memristor-based neural networks: a bridge from device to artificial intelligence.Nanoscale Horiz. 2023 May 30;8(6):716-745. doi: 10.1039/d2nh00536k. Nanoscale Horiz. 2023. PMID: 36946082 Review.
-
Synapse-Mimetic Hardware-Implemented Resistive Random-Access Memory for Artificial Neural Network.Sensors (Basel). 2023 Mar 14;23(6):3118. doi: 10.3390/s23063118. Sensors (Basel). 2023. PMID: 36991829 Free PMC article. Review.
Cited by
-
An in-memory computing architecture based on two-dimensional semiconductors for multiply-accumulate operations.Nat Commun. 2021 Jun 7;12(1):3347. doi: 10.1038/s41467-021-23719-3. Nat Commun. 2021. PMID: 34099710 Free PMC article.
-
Implementation of Dropout Neuronal Units Based on Stochastic Memristive Devices in Neural Networks with High Classification Accuracy.Adv Sci (Weinh). 2020 Jul 26;7(18):2001842. doi: 10.1002/advs.202001842. eCollection 2020 Sep. Adv Sci (Weinh). 2020. PMID: 32999852 Free PMC article.
-
Proposition of Adaptive Read Bias: A Solution to Overcome Power and Scaling Limitations in Ferroelectric-Based Neuromorphic System.Adv Sci (Weinh). 2024 Feb;11(5):e2303735. doi: 10.1002/advs.202303735. Epub 2023 Dec 1. Adv Sci (Weinh). 2024. PMID: 38039488 Free PMC article.
-
Versatile stochastic dot product circuits based on nonvolatile memories for high performance neurocomputing and neurooptimization.Nat Commun. 2019 Nov 8;10(1):5113. doi: 10.1038/s41467-019-13103-7. Nat Commun. 2019. PMID: 31704925 Free PMC article.
-
Metal-Organic Frameworks-Based Memristors: Materials, Devices, and Applications.Molecules. 2022 Dec 14;27(24):8888. doi: 10.3390/molecules27248888. Molecules. 2022. PMID: 36558025 Free PMC article. Review.
References
-
- Jouppi, N. P. et al. In-datacenter performance analysis of a tensor processing unit. In 44th International Symposium on Computer Architecture (ISCA) 1–12 (ACM, Toronto, ON, Canada, 2017).
-
- Chen, Y. et al. DaDianNao: A machine-learning supercomputer. In 47th Annual IEEE/ACM International Symposium on Microarchitecture 609–622 (IEEE, Cambridge, UK, 2014).
Publication types
Grants and funding
LinkOut - more resources
Full Text Sources
Other Literature Sources