Glimpsing speech interrupted by speech-modulated noise
- PMID: 29857765
- PMCID: PMC5966307
- DOI: 10.1121/1.5038273
Glimpsing speech interrupted by speech-modulated noise
Abstract
Everyday environments frequently present speech in modulated noise backgrounds, such as from a competing talker. Under such conditions, temporal glimpses of speech may be preserved at favorable signal-to-noise ratios during the amplitude dips of the masker. Speech recognition is determined, in part, by these speech glimpses. However, properties of the noise when it dominates the speech may also be important. This study interrupted speech to provide either high-intensity or low-intensity speech glimpses derived from measurements of speech-on-speech masking. These interrupted intervals were deleted and subsequently filled by steady-state noise or one of four different types of noise amplitude modulated by the same or different sentence. Noise was presented at two different levels. Interruption by silence was also examined. Speech recognition was best with high-intensity glimpses and improved when the noise was modulated by missing high-intensity segments. Additional noise conditions detailed significant interactions between the noise level and glimpsed speech level. Overall, high-intensity speech segments, and the amplitude modulation (AM) of the segments, are crucial for speech recognition. Speech recognition is further influenced by the properties of the competing noise (i.e., level and AM) which interact with the glimpsed speech level. Acoustic properties of both speech-dominated and noise-dominated intervals of speech-noise mixtures determine speech recognition.
Figures
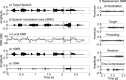
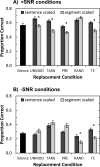
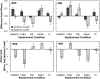
Similar articles
-
Modulation masking and glimpsing of natural and vocoded speech during single-talker modulated noise: Effect of the modulation spectrum.J Acoust Soc Am. 2016 Sep;140(3):1800. doi: 10.1121/1.4962494. J Acoust Soc Am. 2016. PMID: 27914381 Free PMC article.
-
Glimpsing speech in temporally and spectro-temporally modulated noise.J Acoust Soc Am. 2018 May;143(5):3047. doi: 10.1121/1.5038266. J Acoust Soc Am. 2018. PMID: 29857753 Free PMC article.
-
Age effects on perceptual organization of speech: Contributions of glimpsing, phonemic restoration, and speech segregation.J Acoust Soc Am. 2018 Jul;144(1):267. doi: 10.1121/1.5044397. J Acoust Soc Am. 2018. PMID: 30075693 Free PMC article.
-
Glimpsing Speech in the Presence of Nonsimultaneous Amplitude Modulations From a Competing Talker: Effect of Modulation Rate, Age, and Hearing Loss.J Speech Lang Hear Res. 2016 Oct 1;59(5):1198-1207. doi: 10.1044/2016_JSLHR-H-15-0259. J Speech Lang Hear Res. 2016. PMID: 27603264 Free PMC article.
-
Spectro-temporal modulation glimpsing for speech intelligibility prediction.Hear Res. 2022 Dec;426:108620. doi: 10.1016/j.heares.2022.108620. Epub 2022 Sep 21. Hear Res. 2022. PMID: 36175300 Free PMC article. Review.
Cited by
-
Speech Intelligibility Prediction using Spectro-Temporal Modulation Analysis.IEEE/ACM Trans Audio Speech Lang Process. 2021;29:210-225. doi: 10.1109/taslp.2020.3039929. Epub 2020 Nov 24. IEEE/ACM Trans Audio Speech Lang Process. 2021. PMID: 33748329 Free PMC article.
-
Access to semantic cues does not lead to perceptual restoration of interrupted speech in cochlear-implant users.J Acoust Soc Am. 2021 Mar;149(3):1488. doi: 10.1121/10.0003573. J Acoust Soc Am. 2021. PMID: 33765790 Free PMC article.
-
The Perceptual Contribution of Consonants and Vowels to Sentence Recognition: Effect of Dialect Variation in American English.Proc Int Congr Phon Sci. 2019 Aug;2019:3240-3244. Proc Int Congr Phon Sci. 2019. PMID: 37141140 Free PMC article.
-
[Audiometric test battery for presbycusis].HNO. 2020 Mar;68(3):164-170. doi: 10.1007/s00106-019-00746-7. HNO. 2020. PMID: 31549193 Review. German.
References
-
- Apoux, F. , and Healy, E. W. (2013). “ A glimpsing account of the role of temporal fine structure information in speech recognition,” in ( Springer, New York), pp. 119–126. - PubMed
Publication types
MeSH terms
Grants and funding
LinkOut - more resources
Full Text Sources
Other Literature Sources
Medical