Synaptic E-I Balance Underlies Efficient Neural Coding
- PMID: 29456491
- PMCID: PMC5801300
- DOI: 10.3389/fnins.2018.00046
Synaptic E-I Balance Underlies Efficient Neural Coding
Abstract
Both theoretical and experimental evidence indicate that synaptic excitation and inhibition in the cerebral cortex are well-balanced during the resting state and sensory processing. Here, we briefly summarize the evidence for how neural circuits are adjusted to achieve this balance. Then, we discuss how such excitatory and inhibitory balance shapes stimulus representation and information propagation, two basic functions of neural coding. We also point out the benefit of adopting such a balance during neural coding. We conclude that excitatory and inhibitory balance may be a fundamental mechanism underlying efficient coding.
Keywords: energy efficiency; excitatory-inhibitory balance; information propagation; sparse coding; stimulus representation.
Figures
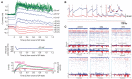
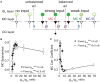
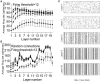
Similar articles
-
Synaptic Excitatory-Inhibitory Balance Underlying Efficient Neural Coding.Adv Neurobiol. 2018;21:85-100. doi: 10.1007/978-3-319-94593-4_5. Adv Neurobiol. 2018. PMID: 30334221
-
Enhanced Thalamocortical Synaptic Transmission and Dysregulation of the Excitatory-Inhibitory Balance at the Thalamocortical Feedforward Inhibitory Microcircuit in a Genetic Mouse Model of Migraine.J Neurosci. 2019 Dec 4;39(49):9841-9851. doi: 10.1523/JNEUROSCI.1840-19.2019. Epub 2019 Oct 23. J Neurosci. 2019. PMID: 31645463 Free PMC article.
-
Efficient Coding and Energy Efficiency Are Promoted by Balanced Excitatory and Inhibitory Synaptic Currents in Neuronal Network.Front Cell Neurosci. 2018 May 3;12:123. doi: 10.3389/fncel.2018.00123. eCollection 2018. Front Cell Neurosci. 2018. PMID: 29773979 Free PMC article.
-
Energy-efficient neural information processing in individual neurons and neuronal networks.J Neurosci Res. 2017 Nov;95(11):2253-2266. doi: 10.1002/jnr.24131. Epub 2017 Aug 22. J Neurosci Res. 2017. PMID: 28833444 Review.
-
Efficient codes and balanced networks.Nat Neurosci. 2016 Mar;19(3):375-82. doi: 10.1038/nn.4243. Nat Neurosci. 2016. PMID: 26906504 Review.
Cited by
-
Inhibitory cell populations depend on age, sex, and prior experience across a neural network for Critical Period learning.Sci Rep. 2019 Dec 27;9(1):19867. doi: 10.1038/s41598-019-56293-2. Sci Rep. 2019. PMID: 31882750 Free PMC article.
-
Perturbation of Cortical Excitability in a Conditional Model of PCDH19 Disorder.Cells. 2022 Jun 16;11(12):1939. doi: 10.3390/cells11121939. Cells. 2022. PMID: 35741068 Free PMC article.
-
Increased excitatory to inhibitory synaptic ratio in parietal cortex samples from individuals with Alzheimer's disease.Nat Commun. 2021 May 10;12(1):2603. doi: 10.1038/s41467-021-22742-8. Nat Commun. 2021. PMID: 33972518 Free PMC article.
-
DNA Methylation in Autism Spectrum Disorders: Biomarker or Pharmacological Target?Brain Sci. 2024 Jul 23;14(8):737. doi: 10.3390/brainsci14080737. Brain Sci. 2024. PMID: 39199432 Free PMC article. Review.
-
Role of Lateral Inhibition on Visual Number Sense.Front Comput Neurosci. 2022 Jun 20;16:810448. doi: 10.3389/fncom.2022.810448. eCollection 2022. Front Comput Neurosci. 2022. PMID: 35795083 Free PMC article.
References
Publication types
LinkOut - more resources
Full Text Sources
Other Literature Sources