Learning to make external sensory stimulus predictions using internal correlations in populations of neurons
- PMID: 29348208
- PMCID: PMC5798322
- DOI: 10.1073/pnas.1710779115
Learning to make external sensory stimulus predictions using internal correlations in populations of neurons
Abstract
To compensate for sensory processing delays, the visual system must make predictions to ensure timely and appropriate behaviors. Recent work has found predictive information about the stimulus in neural populations early in vision processing, starting in the retina. However, to utilize this information, cells downstream must be able to read out the predictive information from the spiking activity of retinal ganglion cells. Here we investigate whether a downstream cell could learn efficient encoding of predictive information in its inputs from the correlations in the inputs themselves, in the absence of other instructive signals. We simulate learning driven by spiking activity recorded in salamander retina. We model a downstream cell as a binary neuron receiving a small group of weighted inputs and quantify the predictive information between activity in the binary neuron and future input. Input weights change according to spike timing-dependent learning rules during a training period. We characterize the readouts learned under spike timing-dependent synaptic update rules, finding that although the fixed points of learning dynamics are not associated with absolute optimal readouts they convey nearly all of the information conveyed by the optimal readout. Moreover, we find that learned perceptrons transmit position and velocity information of a moving-bar stimulus nearly as efficiently as optimal perceptrons. We conclude that predictive information is, in principle, readable from the perspective of downstream neurons in the absence of other inputs. This suggests an important role for feedforward prediction in sensory encoding.
Keywords: information theory; learning; plasticity; prediction; retina.
Conflict of interest statement
The authors declare no conflict of interest.
Figures
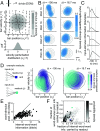
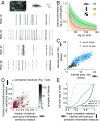
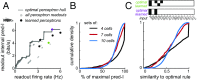
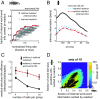
Similar articles
-
Computing complex visual features with retinal spike times.PLoS One. 2013;8(1):e53063. doi: 10.1371/journal.pone.0053063. Epub 2013 Jan 2. PLoS One. 2013. PMID: 23301021 Free PMC article.
-
How neural interactions form neural responses in the salamander retina.J Comput Neurosci. 1997 Jan;4(1):5-27. doi: 10.1023/a:1008840709467. J Comput Neurosci. 1997. PMID: 9046449
-
Decoding visual information from a population of retinal ganglion cells.J Neurophysiol. 1997 Nov;78(5):2336-50. doi: 10.1152/jn.1997.78.5.2336. J Neurophysiol. 1997. PMID: 9356386
-
Information processing in the primate retina: circuitry and coding.Annu Rev Neurosci. 2007;30:1-30. doi: 10.1146/annurev.neuro.30.051606.094252. Annu Rev Neurosci. 2007. PMID: 17335403 Review.
-
Neuromodulated Spike-Timing-Dependent Plasticity, and Theory of Three-Factor Learning Rules.Front Neural Circuits. 2016 Jan 19;9:85. doi: 10.3389/fncir.2015.00085. eCollection 2015. Front Neural Circuits. 2016. PMID: 26834568 Free PMC article. Review.
Cited by
-
Sources of predictive information in dynamical neural networks.Sci Rep. 2020 Oct 9;10(1):16901. doi: 10.1038/s41598-020-73380-x. Sci Rep. 2020. PMID: 33037274 Free PMC article.
-
Spiking time-dependent plasticity leads to efficient coding of predictions.Biol Cybern. 2020 Feb;114(1):43-61. doi: 10.1007/s00422-019-00813-w. Epub 2019 Dec 24. Biol Cybern. 2020. PMID: 31873797 Free PMC article.
-
Maximally efficient prediction in the early fly visual system may support evasive flight maneuvers.PLoS Comput Biol. 2021 May 20;17(5):e1008965. doi: 10.1371/journal.pcbi.1008965. eCollection 2021 May. PLoS Comput Biol. 2021. PMID: 34014926 Free PMC article.
-
Efficient coding, channel capacity, and the emergence of retinal mosaics.Adv Neural Inf Process Syst. 2022 Dec;35:32311-32324. Adv Neural Inf Process Syst. 2022. PMID: 37168261 Free PMC article.
-
Optimal prediction with resource constraints using the information bottleneck.PLoS Comput Biol. 2021 Mar 8;17(3):e1008743. doi: 10.1371/journal.pcbi.1008743. eCollection 2021 Mar. PLoS Comput Biol. 2021. PMID: 33684112 Free PMC article.
References
-
- Segev R, Puchalla J, Berry MJ., 2nd Functional organization of ganglion cells in the salamander retina. J Neurophysiol. 2006;95:2277–2292. - PubMed
-
- Srinivasan MV, Laughlin SB, Dubs A. Predictive coding: A fresh view of inhibition in the retina. Proc R Soc Lond B Biol Sci. 1982;216:427–459. - PubMed
-
- Hosoya T, Baccus SA, Meister M. Dynamic predictive coding by the retina. Nature. 2005;436:71–77. - PubMed
Publication types
MeSH terms
LinkOut - more resources
Full Text Sources
Other Literature Sources