The Computational Anatomy of Visual Neglect
- PMID: 29190328
- PMCID: PMC6005118
- DOI: 10.1093/cercor/bhx316
The Computational Anatomy of Visual Neglect
Abstract
Visual neglect is a debilitating neuropsychological phenomenon that has many clinical implications and-in cognitive neuroscience-offers an important lesion deficit model. In this article, we describe a computational model of visual neglect based upon active inference. Our objective is to establish a computational and neurophysiological process theory that can be used to disambiguate among the various causes of this important syndrome; namely, a computational neuropsychology of visual neglect. We introduce a Bayes optimal model based upon Markov decision processes that reproduces the visual searches induced by the line cancellation task (used to characterize visual neglect at the bedside). We then consider 3 distinct ways in which the model could be lesioned to reproduce neuropsychological (visual search) deficits. Crucially, these 3 levels of pathology map nicely onto the neuroanatomy of saccadic eye movements and the systems implicated in visual neglect.
Keywords: Bayesian; active inference; neuropsychology; saccades; visual neglect.
© The Author 2017. Published by Oxford University Press.
Figures
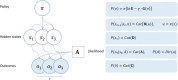
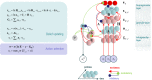
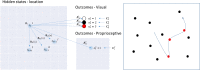
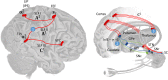
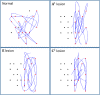
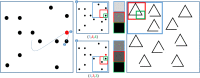
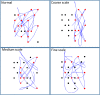
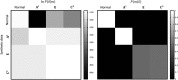
Similar articles
-
Task-related modulation of visual neglect in cancellation tasks.Neuropsychologia. 2009 Jan;47(1):91-103. doi: 10.1016/j.neuropsychologia.2008.08.020. Epub 2008 Aug 26. Neuropsychologia. 2009. PMID: 18790703 Free PMC article.
-
The imbalance of oculomotor capture in unilateral visual neglect.Conscious Cogn. 2010 Mar;19(1):186-97. doi: 10.1016/j.concog.2009.11.003. Epub 2009 Dec 8. Conscious Cogn. 2010. PMID: 20004118
-
Left ipsilesional neglect for visual imagery: a mental image generation impairment?Neurocase. 2006 Jun;12(3):197-206. doi: 10.1080/13554790600665516. Neurocase. 2006. PMID: 16801155
-
Time and neglect: abnormal temporal dynamics in unilateral spatial neglect.Neuropsychologia. 2006;44(14):2775-82. doi: 10.1016/j.neuropsychologia.2006.06.011. Epub 2006 Jul 31. Neuropsychologia. 2006. PMID: 16876831 Review.
-
Spatial neglect and attention networks.Annu Rev Neurosci. 2011;34:569-99. doi: 10.1146/annurev-neuro-061010-113731. Annu Rev Neurosci. 2011. PMID: 21692662 Free PMC article. Review.
Cited by
-
Simulating the computational mechanisms of cognitive and behavioral psychotherapeutic interventions: insights from active inference.Sci Rep. 2021 May 12;11(1):10128. doi: 10.1038/s41598-021-89047-0. Sci Rep. 2021. PMID: 33980875 Free PMC article.
-
A Bayesian Account of Generalist and Specialist Formation Under the Active Inference Framework.Front Artif Intell. 2020 Sep 3;3:69. doi: 10.3389/frai.2020.00069. eCollection 2020. Front Artif Intell. 2020. PMID: 33733186 Free PMC article.
-
Precision and False Perceptual Inference.Front Integr Neurosci. 2018 Sep 20;12:39. doi: 10.3389/fnint.2018.00039. eCollection 2018. Front Integr Neurosci. 2018. PMID: 30294264 Free PMC article.
-
Disconnectomics to unravel the network underlying deficits of spatial exploration and attention.Sci Rep. 2022 Dec 24;12(1):22315. doi: 10.1038/s41598-022-26491-6. Sci Rep. 2022. PMID: 36566307 Free PMC article.
-
Prefrontal Computation as Active Inference.Cereb Cortex. 2020 Mar 21;30(2):682-695. doi: 10.1093/cercor/bhz118. Cereb Cortex. 2020. PMID: 31298270 Free PMC article.
References
-
- Albert ML. 1973. A simple test of visual neglect. Neurology. 23:658. - PubMed
-
- Andersen RA, Essick GK, Siegel RM. 1985. Encoding of spatial location by posterior parietal neurons. Science. 230:456. - PubMed
-
- Auclair L, Siéroff E, Kocer S. 2008. A case of spatial neglect dysgraphia in Wilson’s Disease. Arch Clin Neuropsychol. 23:47–62. - PubMed
Publication types
MeSH terms
Grants and funding
LinkOut - more resources
Full Text Sources
Other Literature Sources