Residual Convolutional Neural Network for the Determination of IDH Status in Low- and High-Grade Gliomas from MR Imaging
- PMID: 29167275
- PMCID: PMC6051535
- DOI: 10.1158/1078-0432.CCR-17-2236
Residual Convolutional Neural Network for the Determination of IDH Status in Low- and High-Grade Gliomas from MR Imaging
Abstract
Purpose: Isocitrate dehydrogenase (IDH) mutations in glioma patients confer longer survival and may guide treatment decision making. We aimed to predict the IDH status of gliomas from MR imaging by applying a residual convolutional neural network to preoperative radiographic data.Experimental Design: Preoperative imaging was acquired for 201 patients from the Hospital of University of Pennsylvania (HUP), 157 patients from Brigham and Women's Hospital (BWH), and 138 patients from The Cancer Imaging Archive (TCIA) and divided into training, validation, and testing sets. We trained a residual convolutional neural network for each MR sequence (FLAIR, T2, T1 precontrast, and T1 postcontrast) and built a predictive model from the outputs. To increase the size of the training set and prevent overfitting, we augmented the training set images by introducing random rotations, translations, flips, shearing, and zooming.Results: With our neural network model, we achieved IDH prediction accuracies of 82.8% (AUC = 0.90), 83.0% (AUC = 0.93), and 85.7% (AUC = 0.94) within training, validation, and testing sets, respectively. When age at diagnosis was incorporated into the model, the training, validation, and testing accuracies increased to 87.3% (AUC = 0.93), 87.6% (AUC = 0.95), and 89.1% (AUC = 0.95), respectively.Conclusions: We developed a deep learning technique to noninvasively predict IDH genotype in grade II-IV glioma using conventional MR imaging using a multi-institutional data set. Clin Cancer Res; 24(5); 1073-81. ©2017 AACR.
©2017 American Association for Cancer Research.
Conflict of interest statement
The authors declare no potential conflicts of interest
Figures
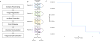
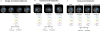
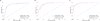
Similar articles
-
Machine learning reveals multimodal MRI patterns predictive of isocitrate dehydrogenase and 1p/19q status in diffuse low- and high-grade gliomas.J Neurooncol. 2019 Apr;142(2):299-307. doi: 10.1007/s11060-019-03096-0. Epub 2019 Jan 19. J Neurooncol. 2019. PMID: 30661193 Free PMC article.
-
Prediction of IDH genotype in gliomas with dynamic susceptibility contrast perfusion MR imaging using an explainable recurrent neural network.Neuro Oncol. 2019 Sep 6;21(9):1197-1209. doi: 10.1093/neuonc/noz095. Neuro Oncol. 2019. PMID: 31127834 Free PMC article.
-
Multimodal MRI features predict isocitrate dehydrogenase genotype in high-grade gliomas.Neuro Oncol. 2017 Jan;19(1):109-117. doi: 10.1093/neuonc/now121. Epub 2016 Jun 26. Neuro Oncol. 2017. PMID: 27353503 Free PMC article.
-
Diagnostic accuracy and potential covariates for machine learning to identify IDH mutations in glioma patients: evidence from a meta-analysis.Eur Radiol. 2020 Aug;30(8):4664-4674. doi: 10.1007/s00330-020-06717-9. Epub 2020 Mar 19. Eur Radiol. 2020. PMID: 32193643 Review.
-
Radiogenomic association between the T2-FLAIR mismatch sign and IDH mutation status in adult patients with lower-grade gliomas: an updated systematic review and meta-analysis.Eur Radiol. 2022 Aug;32(8):5339-5352. doi: 10.1007/s00330-022-08607-8. Epub 2022 Feb 15. Eur Radiol. 2022. PMID: 35169897 Review.
Cited by
-
Deep Learning: A Primer for Neurosurgeons.Adv Exp Med Biol. 2024;1462:39-70. doi: 10.1007/978-3-031-64892-2_4. Adv Exp Med Biol. 2024. PMID: 39523259 Review.
-
Leveraging State-of-the-Art AI Algorithms in Personalized Oncology: From Transcriptomics to Treatment.Diagnostics (Basel). 2024 Sep 29;14(19):2174. doi: 10.3390/diagnostics14192174. Diagnostics (Basel). 2024. PMID: 39410578 Free PMC article.
-
-New frontiers in domain-inspired radiomics and radiogenomics: increasing role of molecular diagnostics in CNS tumor classification and grading following WHO CNS-5 updates.Cancer Imaging. 2024 Oct 7;24(1):133. doi: 10.1186/s40644-024-00769-6. Cancer Imaging. 2024. PMID: 39375809 Free PMC article. Review.
-
Non-invasive classification of IDH mutation status of gliomas from multi-modal MRI using a 3D convolutional neural network.Proc SPIE Int Soc Opt Eng. 2023 Feb;12465:124650W. doi: 10.1117/12.2651391. Epub 2023 Apr 7. Proc SPIE Int Soc Opt Eng. 2023. PMID: 39257452 Free PMC article.
-
TGIF2 is a potential biomarker for diagnosis and prognosis of glioma.Front Immunol. 2024 Feb 26;15:1356833. doi: 10.3389/fimmu.2024.1356833. eCollection 2024. Front Immunol. 2024. PMID: 38629068 Free PMC article.
References
-
- Cancer Genome Atlas Research Network. Brat DJ, Verhaak RGW, Aldape KD, Yung WKA, Salama SR, et al. Comprehensive, Integrative Genomic Analysis of Diffuse Lower-Grade Gliomas. N Engl J Med [Internet] 2015;372:2481–98. cited 2017 Feb 28. Available from: http://www.ncbi.nlm.nih.gov/pubmed/26061751. - PMC - PubMed
-
- Schomas DA, Laack NNI, Rao RD, Meyer FB, Shaw EG, O’Neill BP, et al. Intracranial low-grade gliomas in adults: 30-year experience with long-term follow-up at Mayo Clinic. Neuro Oncol [Internet] 2009;11:437–45. cited 2017 Feb 28. Available from: http://www.ncbi.nlm.nih.gov/pubmed/19018039. - PMC - PubMed
-
- Pouratian N, Schiff D. Management of Low-Grade Glioma. Curr Neurol Neurosci Rep [Internet] 2010;10:224–31. cited 2017 Apr 7. Available from: http://www.ncbi.nlm.nih.gov/pubmed/20425038. - PMC - PubMed
-
- Venneti S, Huse JT. The Evolving Molecular Genetics of Low-grade Glioma. Adv Anat Pathol [Internet] 2015;22:94–101. cited 2017 Apr 7. Available from: http://www.ncbi.nlm.nih.gov/pubmed/25664944. - PMC - PubMed
-
- Ostrom QT, Gittleman H, Fulop J, Liu M, Blanda R, Kromer C, et al. CBTRUS Statistical Report: Primary Brain and Central Nervous System Tumors Diagnosed in the United States in 2008–2012. Neuro Oncol [Internet] 2015;17(Suppl 4):iv1–iv62. cited 2015 Nov 7. Available from: http://www.ncbi.nlm.nih.gov/pubmed/26511214. - PMC - PubMed
Publication types
MeSH terms
Substances
Grants and funding
LinkOut - more resources
Full Text Sources
Other Literature Sources
Medical
Miscellaneous