Sparse synaptic connectivity is required for decorrelation and pattern separation in feedforward networks
- PMID: 29061964
- PMCID: PMC5653655
- DOI: 10.1038/s41467-017-01109-y
Sparse synaptic connectivity is required for decorrelation and pattern separation in feedforward networks
Abstract
Pattern separation is a fundamental function of the brain. The divergent feedforward networks thought to underlie this computation are widespread, yet exhibit remarkably similar sparse synaptic connectivity. Marr-Albus theory postulates that such networks separate overlapping activity patterns by mapping them onto larger numbers of sparsely active neurons. But spatial correlations in synaptic input and those introduced by network connectivity are likely to compromise performance. To investigate the structural and functional determinants of pattern separation we built models of the cerebellar input layer with spatially correlated input patterns, and systematically varied their synaptic connectivity. Performance was quantified by the learning speed of a classifier trained on either the input or output patterns. Our results show that sparse synaptic connectivity is essential for separating spatially correlated input patterns over a wide range of network activity, and that expansion and correlations, rather than sparse activity, are the major determinants of pattern separation.
Conflict of interest statement
The authors declare no competing financial interests.
Figures
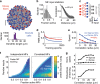
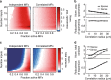
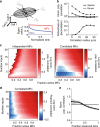
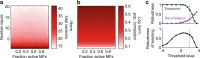
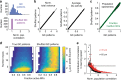
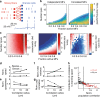
Similar articles
-
Network structure within the cerebellar input layer enables lossless sparse encoding.Neuron. 2014 Aug 20;83(4):960-74. doi: 10.1016/j.neuron.2014.07.020. Epub 2014 Aug 7. Neuron. 2014. PMID: 25123311 Free PMC article.
-
The hypergeometric connectivity hypothesis: divergent performance of brain circuits with different synaptic connectivity distributions.Brain Res. 2008 Apr 2;1202:3-13. doi: 10.1016/j.brainres.2007.06.044. Epub 2007 Jul 10. Brain Res. 2008. PMID: 17719016
-
Propagation delays determine neuronal activity and synaptic connectivity patterns emerging in plastic neuronal networks.Chaos. 2018 Oct;28(10):106308. doi: 10.1063/1.5037309. Chaos. 2018. PMID: 30384625 Review.
-
Modulation of the dynamics of cerebellar Purkinje cells through the interaction of excitatory and inhibitory feedforward pathways.PLoS Comput Biol. 2021 Feb 10;17(2):e1008670. doi: 10.1371/journal.pcbi.1008670. eCollection 2021 Feb. PLoS Comput Biol. 2021. PMID: 33566820 Free PMC article.
-
Computer simulation of cerebellar information processing.Nat Neurosci. 2000 Nov;3 Suppl:1205-11. doi: 10.1038/81486. Nat Neurosci. 2000. PMID: 11127839 Review.
Cited by
-
Neural Classifiers with Limited Connectivity and Recurrent Readouts.J Neurosci. 2018 Nov 14;38(46):9900-9924. doi: 10.1523/JNEUROSCI.3506-17.2018. Epub 2018 Sep 24. J Neurosci. 2018. PMID: 30249794 Free PMC article.
-
Gradient-free training of recurrent neural networks using random perturbations.Front Neurosci. 2024 Jul 10;18:1439155. doi: 10.3389/fnins.2024.1439155. eCollection 2024. Front Neurosci. 2024. PMID: 39050673 Free PMC article.
-
Predictive learning as a network mechanism for extracting low-dimensional latent space representations.Nat Commun. 2021 Mar 3;12(1):1417. doi: 10.1038/s41467-021-21696-1. Nat Commun. 2021. PMID: 33658520 Free PMC article.
-
Cerebellar granule cell axons support high-dimensional representations.Nat Neurosci. 2021 Aug;24(8):1142-1150. doi: 10.1038/s41593-021-00873-x. Epub 2021 Jun 24. Nat Neurosci. 2021. PMID: 34168340 Free PMC article.
-
GluA4 facilitates cerebellar expansion coding and enables associative memory formation.Elife. 2021 Jul 5;10:e65152. doi: 10.7554/eLife.65152. Elife. 2021. PMID: 34219651 Free PMC article.
References
-
- Albus JS. A Theory of Cerebellar Function. Math. Biosci. 1971;10:25–61. doi: 10.1016/0025-5564(71)90051-4. - DOI
-
- Kanerva, P. Sparse Distributed Memory. (MIT Press, Cambridge, Massachusetts, 1988).
-
- Földiak, P. Sparse coding in the primate cortex. In The Handbook of Brain Theory and Neural Networks (ed. Arbib, M. A.) 1064–1068 (MIT Press, 2002).
Publication types
MeSH terms
Grants and funding
LinkOut - more resources
Full Text Sources
Other Literature Sources
Molecular Biology Databases