Predicting green: really radical (plant) predictive processing
- PMID: 28637913
- PMCID: PMC5493793
- DOI: 10.1098/rsif.2017.0096
Predicting green: really radical (plant) predictive processing
Abstract
In this article we account for the way plants respond to salient features of their environment under the free-energy principle for biological systems. Biological self-organization amounts to the minimization of surprise over time. We posit that any self-organizing system must embody a generative model whose predictions ensure that (expected) free energy is minimized through action. Plants respond in a fast, and yet coordinated manner, to environmental contingencies. They pro-actively sample their local environment to elicit information with an adaptive value. Our main thesis is that plant behaviour takes place by way of a process (active inference) that predicts the environmental sources of sensory stimulation. This principle, we argue, endows plants with a form of perception that underwrites purposeful, anticipatory behaviour. The aim of the article is to assess the prospects of a radical predictive processing story that would follow naturally from the free-energy principle for biological systems; an approach that may ultimately bear upon our understanding of life and cognition more broadly.
Keywords: affordance; embodiment; free energy; perceptual/active inference; plant intelligence; predictive processing.
© 2017 The Author(s).
Conflict of interest statement
The authors have no competing interests.
Figures
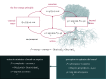



Similar articles
-
What is plant behaviour?Plant Cell Environ. 2009 Jun;32(6):606-16. doi: 10.1111/j.1365-3040.2009.01929.x. Epub 2009 Jan 1. Plant Cell Environ. 2009. PMID: 19143994 Review.
-
Toward an Embodied, Embedded Predictive Processing Account.Front Psychol. 2021 Jan 29;12:543076. doi: 10.3389/fpsyg.2021.543076. eCollection 2021. Front Psychol. 2021. PMID: 33584461 Free PMC article.
-
Aspects of plant intelligence.Ann Bot. 2003 Jul;92(1):1-20. doi: 10.1093/aob/mcg101. Epub 2003 May 9. Ann Bot. 2003. PMID: 12740212 Free PMC article. Review.
-
Plant development and reproduction in a changing environment.J Exp Bot. 2024 Jul 23;75(14):4167-4170. doi: 10.1093/jxb/erae285. J Exp Bot. 2024. PMID: 39039837
-
"Feature Detection" vs. "Predictive Coding" Models of Plant Behavior.Front Psychol. 2016 Oct 4;7:1505. doi: 10.3389/fpsyg.2016.01505. eCollection 2016. Front Psychol. 2016. PMID: 27757094 Free PMC article.
Cited by
-
Plants are intelligent, here's how.Ann Bot. 2020 Jan 8;125(1):11-28. doi: 10.1093/aob/mcz155. Ann Bot. 2020. PMID: 31563953 Free PMC article.
-
Future medicine: from molecular pathways to the collective intelligence of the body.Trends Mol Med. 2023 Sep;29(9):687-710. doi: 10.1016/j.molmed.2023.06.007. Epub 2023 Jul 20. Trends Mol Med. 2023. PMID: 37481382 Free PMC article. Review.
-
How Psychedelic-Assisted Treatment Works in the Bayesian Brain.Front Psychiatry. 2022 Mar 8;13:812180. doi: 10.3389/fpsyt.2022.812180. eCollection 2022. Front Psychiatry. 2022. PMID: 35360137 Free PMC article.
-
The Active Inference Approach to Ecological Perception: General Information Dynamics for Natural and Artificial Embodied Cognition.Front Robot AI. 2018 Mar 8;5:21. doi: 10.3389/frobt.2018.00021. eCollection 2018. Front Robot AI. 2018. PMID: 33500908 Free PMC article.
-
How particular is the physics of the free energy principle?Phys Life Rev. 2022 Mar;40:24-50. doi: 10.1016/j.plrev.2021.11.001. Epub 2021 Nov 23. Phys Life Rev. 2022. PMID: 34895862 Free PMC article. Review.
References
-
- Baluška F, Mancuso S, Volkmann D (eds). 2006. Communication in plants: neuronal aspects of plant life. New York, NY: Springer.
-
- Trewavas A. 2014. Plant behaviour and intelligence. Oxford, UK: Oxford University Press.
-
- Calvo P. 2016. The philosophy of plant neurobiology: a manifesto. Synthese 193, 1323–1343. (10.1007/s11229-016-1040-1) - DOI
-
- Calvo P, Martín E, Symons J. 2014. The emergence of systematicity in minimally cognitive agents. In The architecture of cognition: rethinking Fodor and Pylyshyn's systematicity challenge (eds Calvo P, Symons J), pp. 397–434. Cambridge, MA: MIT Press.
Publication types
MeSH terms
Grants and funding
LinkOut - more resources
Full Text Sources
Other Literature Sources