Major Depression Detection from EEG Signals Using Kernel Eigen-Filter-Bank Common Spatial Patterns
- PMID: 28613237
- PMCID: PMC5492453
- DOI: 10.3390/s17061385
Major Depression Detection from EEG Signals Using Kernel Eigen-Filter-Bank Common Spatial Patterns
Abstract
Major depressive disorder (MDD) has become a leading contributor to the global burden of disease; however, there are currently no reliable biological markers or physiological measurements for efficiently and effectively dissecting the heterogeneity of MDD. Here we propose a novel method based on scalp electroencephalography (EEG) signals and a robust spectral-spatial EEG feature extractor called kernel eigen-filter-bank common spatial pattern (KEFB-CSP). The KEFB-CSP first filters the multi-channel raw EEG signals into a set of frequency sub-bands covering the range from theta to gamma bands, then spatially transforms the EEG signals of each sub-band from the original sensor space to a new space where the new signals (i.e., CSPs) are optimal for the classification between MDD and healthy controls, and finally applies the kernel principal component analysis (kernel PCA) to transform the vector containing the CSPs from all frequency sub-bands to a lower-dimensional feature vector called KEFB-CSP. Twelve patients with MDD and twelve healthy controls participated in this study, and from each participant we collected 54 resting-state EEGs of 6 s length (5 min and 24 s in total). Our results show that the proposed KEFB-CSP outperforms other EEG features including the powers of EEG frequency bands, and fractal dimension, which had been widely applied in previous EEG-based depression detection studies. The results also reveal that the 8 electrodes from the temporal areas gave higher accuracies than other scalp areas. The KEFB-CSP was able to achieve an average EEG classification accuracy of 81.23% in single-trial analysis when only the 8-electrode EEGs of the temporal area and a support vector machine (SVM) classifier were used. We also designed a voting-based leave-one-participant-out procedure to test the participant-independent individual classification accuracy. The voting-based results show that the mean classification accuracy of about 80% can be achieved by the KEFP-CSP feature and the SVM classifier with only several trials, and this level of accuracy seems to become stable as more trials (i.e., <7 trials) are used. These findings therefore suggest that the proposed method has a great potential for developing an efficient (required only a few 6-s EEG signals from the 8 electrodes over the temporal) and effective (~80% classification accuracy) EEG-based brain-computer interface (BCI) system which may, in the future, help psychiatrists provide individualized and effective treatments for MDD patients.
Keywords: brain-computer interface (BCI); common spatial pattern (CSP); electroencephalography (EEG); machine learning; major depressive disorder.
Conflict of interest statement
The authors declare no conflict of interest.
Figures
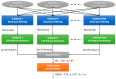
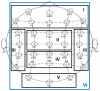
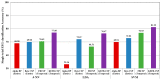
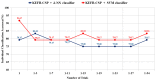
Similar articles
-
Class discrepancy-guided sub-band filter-based common spatial pattern for motor imagery classification.J Neurosci Methods. 2019 Jul 15;323:98-107. doi: 10.1016/j.jneumeth.2019.05.011. Epub 2019 May 26. J Neurosci Methods. 2019. PMID: 31141703
-
The CSP-Based New Features Plus Non-Convex Log Sparse Feature Selection for Motor Imagery EEG Classification.Sensors (Basel). 2020 Aug 22;20(17):4749. doi: 10.3390/s20174749. Sensors (Basel). 2020. PMID: 32842635 Free PMC article.
-
Temporally Constrained Sparse Group Spatial Patterns for Motor Imagery BCI.IEEE Trans Cybern. 2019 Sep;49(9):3322-3332. doi: 10.1109/TCYB.2018.2841847. Epub 2018 Jun 14. IEEE Trans Cybern. 2019. PMID: 29994667
-
Neural Decoding of EEG Signals with Machine Learning: A Systematic Review.Brain Sci. 2021 Nov 18;11(11):1525. doi: 10.3390/brainsci11111525. Brain Sci. 2021. PMID: 34827524 Free PMC article. Review.
-
Electroencephalography Signal Processing: A Comprehensive Review and Analysis of Methods and Techniques.Sensors (Basel). 2023 Jul 16;23(14):6434. doi: 10.3390/s23146434. Sensors (Basel). 2023. PMID: 37514728 Free PMC article. Review.
Cited by
-
SparNet: A Convolutional Neural Network for EEG Space-Frequency Feature Learning and Depression Discrimination.Front Neuroinform. 2022 Jun 2;16:914823. doi: 10.3389/fninf.2022.914823. eCollection 2022. Front Neuroinform. 2022. PMID: 35722169 Free PMC article.
-
Compressibility of High-Density EEG Signals in Stroke Patients.Sensors (Basel). 2018 Nov 23;18(12):4107. doi: 10.3390/s18124107. Sensors (Basel). 2018. PMID: 30477168 Free PMC article.
-
Depressive Disorder Recognition Based on Frontal EEG Signals and Deep Learning.Sensors (Basel). 2023 Oct 23;23(20):8639. doi: 10.3390/s23208639. Sensors (Basel). 2023. PMID: 37896732 Free PMC article.
-
Evaluation of Risk of Bias in Neuroimaging-Based Artificial Intelligence Models for Psychiatric Diagnosis: A Systematic Review.JAMA Netw Open. 2023 Mar 1;6(3):e231671. doi: 10.1001/jamanetworkopen.2023.1671. JAMA Netw Open. 2023. PMID: 36877519 Free PMC article.
-
Automated Depression Detection Using Deep Representation and Sequence Learning with EEG Signals.J Med Syst. 2019 May 28;43(7):205. doi: 10.1007/s10916-019-1345-y. J Med Syst. 2019. PMID: 31139932
References
-
- American Psychiatric Association . Diagnostic and Statistical Manual of Mental Disorders DSM-IV-TR Washington 2000. American Psychiatric Association; Arlington, VA, USA: 2000.
-
- Vos T., Flaxman A.D., Naghavi M., Lozano R., Michaud C., Ezzati M., Shibuya K., Salomon J.A., Abdalla S., Aboyans V., et al. Years lived with disability (YLDs) for 1160 sequelae of 289 diseases and injuries 1990–2010: A systematic analysis for the Global Burden of Disease Study 2010. Lancet. 2012;380:2163–2196. doi: 10.1016/S0140-6736(12)61729-2. - DOI - PMC - PubMed
MeSH terms
LinkOut - more resources
Full Text Sources
Other Literature Sources