Interpreting findings from Mendelian randomization using the MR-Egger method
- PMID: 28527048
- PMCID: PMC5506233
- DOI: 10.1007/s10654-017-0255-x
Interpreting findings from Mendelian randomization using the MR-Egger method
Erratum in
-
Erratum to: Interpreting findings from Mendelian randomization using the MR-Egger method.Eur J Epidemiol. 2017 May;32(5):391-392. doi: 10.1007/s10654-017-0276-5. Eur J Epidemiol. 2017. PMID: 28664250 Free PMC article. No abstract available.
Abstract
Mendelian randomization-Egger (MR-Egger) is an analysis method for Mendelian randomization using summarized genetic data. MR-Egger consists of three parts: (1) a test for directional pleiotropy, (2) a test for a causal effect, and (3) an estimate of the causal effect. While conventional analysis methods for Mendelian randomization assume that all genetic variants satisfy the instrumental variable assumptions, the MR-Egger method is able to assess whether genetic variants have pleiotropic effects on the outcome that differ on average from zero (directional pleiotropy), as well as to provide a consistent estimate of the causal effect, under a weaker assumption-the InSIDE (INstrument Strength Independent of Direct Effect) assumption. In this paper, we provide a critical assessment of the MR-Egger method with regard to its implementation and interpretation. While the MR-Egger method is a worthwhile sensitivity analysis for detecting violations of the instrumental variable assumptions, there are several reasons why causal estimates from the MR-Egger method may be biased and have inflated Type 1 error rates in practice, including violations of the InSIDE assumption and the influence of outlying variants. The issues raised in this paper have potentially serious consequences for causal inferences from the MR-Egger approach. We give examples of scenarios in which the estimates from conventional Mendelian randomization methods and MR-Egger differ, and discuss how to interpret findings in such cases.
Keywords: Instrumental variable; MR-Egger; Mendelian randomization; Robust methods; Summarized data.
Figures
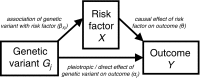
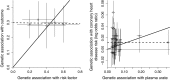
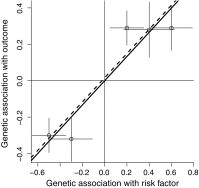
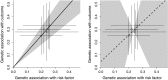
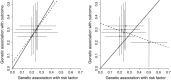
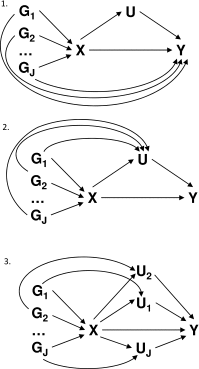
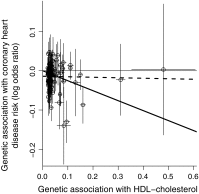
Similar articles
-
Mendelian randomization with invalid instruments: effect estimation and bias detection through Egger regression.Int J Epidemiol. 2015 Apr;44(2):512-25. doi: 10.1093/ije/dyv080. Epub 2015 Jun 6. Int J Epidemiol. 2015. PMID: 26050253 Free PMC article.
-
Extending the MR-Egger method for multivariable Mendelian randomization to correct for both measured and unmeasured pleiotropy.Stat Med. 2017 Dec 20;36(29):4705-4718. doi: 10.1002/sim.7492. Epub 2017 Sep 27. Stat Med. 2017. PMID: 28960498 Free PMC article.
-
Assessing the suitability of summary data for two-sample Mendelian randomization analyses using MR-Egger regression: the role of the I2 statistic.Int J Epidemiol. 2016 Dec 1;45(6):1961-1974. doi: 10.1093/ije/dyw220. Int J Epidemiol. 2016. PMID: 27616674 Free PMC article.
-
Meta-analysis and Mendelian randomization: A review.Res Synth Methods. 2019 Dec;10(4):486-496. doi: 10.1002/jrsm.1346. Epub 2019 Apr 23. Res Synth Methods. 2019. PMID: 30861319 Free PMC article. Review.
-
Methodological challenges in mendelian randomization.Epidemiology. 2014 May;25(3):427-35. doi: 10.1097/EDE.0000000000000081. Epidemiology. 2014. PMID: 24681576 Free PMC article. Review.
Cited by
-
The causal relationship between rheumatoid arthritis and interstitial lung disease in East Asian population: A two-sample Mendelian randomization study.Medicine (Baltimore). 2024 Oct 4;103(40):e39903. doi: 10.1097/MD.0000000000039903. Medicine (Baltimore). 2024. PMID: 39465724 Free PMC article.
-
C-Reactive Protein Level as a Novel Serum Biomarker in Sarcopenia.Mediators Inflamm. 2024 Aug 20;2024:3362336. doi: 10.1155/2024/3362336. eCollection 2024. Mediators Inflamm. 2024. PMID: 39502753 Free PMC article.
-
Estimating the causal effect of frailty index on vestibular disorders: A two-sample Mendelian randomization.Front Neurosci. 2022 Aug 24;16:990682. doi: 10.3389/fnins.2022.990682. eCollection 2022. Front Neurosci. 2022. PMID: 36090295 Free PMC article.
-
No genetic causal association between human papillomavirus and lung cancer risk: a bidirectional two-sample Mendelian randomization analysis.Trials. 2024 Sep 3;25(1):582. doi: 10.1186/s13063-024-08366-5. Trials. 2024. PMID: 39227966 Free PMC article.
-
The association between IGF-1 levels and four types of osteoarthritis: a bidirectional and two-step mendelian randomization study.Front Genet. 2024 Jul 10;15:1366138. doi: 10.3389/fgene.2024.1366138. eCollection 2024. Front Genet. 2024. PMID: 39050250 Free PMC article.
References
-
- Burgess S, Thompson SG. Mendelian randomization: methods for using genetic variants in causal estimation. London: Chapman & Hall; 2015.
Publication types
MeSH terms
Grants and funding
LinkOut - more resources
Full Text Sources
Other Literature Sources