Imaging plus X: multimodal models of neurodegenerative disease
- PMID: 28520598
- PMCID: PMC5491241
- DOI: 10.1097/WCO.0000000000000460
Imaging plus X: multimodal models of neurodegenerative disease
Abstract
Purpose of review: This article argues that the time is approaching for data-driven disease modelling to take centre stage in the study and management of neurodegenerative disease. The snowstorm of data now available to the clinician defies qualitative evaluation; the heterogeneity of data types complicates integration through traditional statistical methods; and the large datasets becoming available remain far from the big-data sizes necessary for fully data-driven machine-learning approaches. The recent emergence of data-driven disease progression models provides a balance between imposed knowledge of disease features and patterns learned from data. The resulting models are both predictive of disease progression in individual patients and informative in terms of revealing underlying biological patterns.
Recent findings: Largely inspired by observational models, data-driven disease progression models have emerged in the last few years as a feasible means for understanding the development of neurodegenerative diseases. These models have revealed insights into frontotemporal dementia, Huntington's disease, multiple sclerosis, Parkinson's disease and other conditions. For example, event-based models have revealed finer graded understanding of progression patterns; self-modelling regression and differential equation models have provided data-driven biomarker trajectories; spatiotemporal models have shown that brain shape changes, for example of the hippocampus, can occur before detectable neurodegeneration; and network models have provided some support for prion-like mechanistic hypotheses of disease propagation. The most mature results are in sporadic Alzheimer's disease, in large part because of the availability of the Alzheimer's disease neuroimaging initiative dataset. Results generally support the prevailing amyloid-led hypothetical model of Alzheimer's disease, while revealing finer detail and insight into disease progression.
Summary: The emerging field of disease progression modelling provides a natural mechanism to integrate different kinds of information, for example from imaging, serum and cerebrospinal fluid markers and cognitive tests, to obtain new insights into progressive diseases. Such insights include fine-grained longitudinal patterns of neurodegeneration, from early stages, and the heterogeneity of these trajectories over the population. More pragmatically, such models enable finer precision in patient staging and stratification, prediction of progression rates and earlier and better identification of at-risk individuals. We argue that this will make disease progression modelling invaluable for recruitment and end-points in future clinical trials, potentially ameliorating the high failure rate in trials of, e.g., Alzheimer's disease therapies. We review the state of the art in these techniques and discuss the future steps required to translate the ideas to front-line application.
Figures
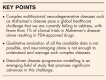
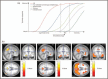
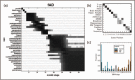
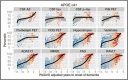
Similar articles
-
DIVE: A spatiotemporal progression model of brain pathology in neurodegenerative disorders.Neuroimage. 2019 May 15;192:166-177. doi: 10.1016/j.neuroimage.2019.02.053. Epub 2019 Mar 4. Neuroimage. 2019. PMID: 30844504
-
A data-driven model of biomarker changes in sporadic Alzheimer's disease.Brain. 2014 Sep;137(Pt 9):2564-77. doi: 10.1093/brain/awu176. Epub 2014 Jul 9. Brain. 2014. PMID: 25012224 Free PMC article.
-
Predictive Big Data Analytics: A Study of Parkinson's Disease Using Large, Complex, Heterogeneous, Incongruent, Multi-Source and Incomplete Observations.PLoS One. 2016 Aug 5;11(8):e0157077. doi: 10.1371/journal.pone.0157077. eCollection 2016. PLoS One. 2016. PMID: 27494614 Free PMC article.
-
Presymptomatic and longitudinal neuroimaging in neurodegeneration--from snapshots to motion picture: a systematic review.J Neurol Neurosurg Psychiatry. 2015 Oct;86(10):1089-96. doi: 10.1136/jnnp-2014-309888. Epub 2015 Jan 28. J Neurol Neurosurg Psychiatry. 2015. PMID: 25632156 Review.
-
Beyond the usual suspects: multi-factorial computational models in the search for neurodegenerative disease mechanisms.Transl Psychiatry. 2024 Sep 23;14(1):386. doi: 10.1038/s41398-024-03073-w. Transl Psychiatry. 2024. PMID: 39313512 Free PMC article. Review.
Cited by
-
Chronic neuropsychiatric sequelae of SARS-CoV-2: Protocol and methods from the Alzheimer's Association Global Consortium.Alzheimers Dement (N Y). 2022 Sep 22;8(1):e12348. doi: 10.1002/trc2.12348. eCollection 2022. Alzheimers Dement (N Y). 2022. PMID: 36185993 Free PMC article.
-
Disease Progression Modeling in Chronic Obstructive Pulmonary Disease.Am J Respir Crit Care Med. 2020 Feb 1;201(3):294-302. doi: 10.1164/rccm.201908-1600OC. Am J Respir Crit Care Med. 2020. PMID: 31657634 Free PMC article.
-
Sequences of cognitive decline in typical Alzheimer's disease and posterior cortical atrophy estimated using a novel event-based model of disease progression.Alzheimers Dement. 2020 Jul;16(7):965-973. doi: 10.1002/alz.12083. Epub 2020 Jun 2. Alzheimers Dement. 2020. PMID: 32489019 Free PMC article.
-
Cyclase-associated protein 2 dimerization regulates cofilin in synaptic plasticity and Alzheimer's disease.Brain Commun. 2020 Jun 26;2(2):fcaa086. doi: 10.1093/braincomms/fcaa086. eCollection 2020. Brain Commun. 2020. PMID: 33094279 Free PMC article.
-
Differences in topological progression profile among neurodegenerative diseases from imaging data.Elife. 2019 Dec 13;8:e49298. doi: 10.7554/eLife.49298. Elife. 2019. PMID: 31793876 Free PMC article.
References
-
- Alzheimer's Disease International, Prince M, Comas-Herrera A, Knapp M, et al. World Alzheimer Report 2016 [Internet]. 2016; https://www.alz.co.uk/research/WorldAlzheimerReport2016.pdf
-
- Bozzali M, Serra L, Cercignani M. Quantitative MRI to understand Alzheimer's disease pathophysiology. Curr Opin Neurol 2016; 29:437–444. - PubMed
-
- Springer International Publishing, Cury C, Lorenzi M, Cash D. Reuter M, Wachinger C, Lombaert H, et al. Spatio-temporal shape analysis of cross-sectional data for detection of early changes in neurodegenerative disease. Spectral and Shape Analysis in Medical Imaging: First International Workshop, SeSAMI 2016, Held in Conjunction with MICCAI 2016, Athens, Greece, October 21, 2016, Revised Selected Papers 2016; 63–75.
Publication types
MeSH terms
LinkOut - more resources
Full Text Sources
Other Literature Sources
Medical
Research Materials