Computational Phenotyping in Psychiatry: A Worked Example
- PMID: 27517087
- PMCID: PMC4969668
- DOI: 10.1523/ENEURO.0049-16.2016
Computational Phenotyping in Psychiatry: A Worked Example
Abstract
Computational psychiatry is a rapidly emerging field that uses model-based quantities to infer the behavioral and neuronal abnormalities that underlie psychopathology. If successful, this approach promises key insights into (pathological) brain function as well as a more mechanistic and quantitative approach to psychiatric nosology-structuring therapeutic interventions and predicting response and relapse. The basic procedure in computational psychiatry is to build a computational model that formalizes a behavioral or neuronal process. Measured behavioral (or neuronal) responses are then used to infer the model parameters of a single subject or a group of subjects. Here, we provide an illustrative overview over this process, starting from the modeling of choice behavior in a specific task, simulating data, and then inverting that model to estimate group effects. Finally, we illustrate cross-validation to assess whether between-subject variables (e.g., diagnosis) can be recovered successfully. Our worked example uses a simple two-step maze task and a model of choice behavior based on (active) inference and Markov decision processes. The procedural steps and routines we illustrate are not restricted to a specific field of research or particular computational model but can, in principle, be applied in many domains of computational psychiatry.
Keywords: Markov decision process; active inference; computational psychiatry; generative model; model inversion.
Figures
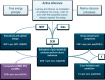
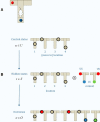
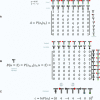
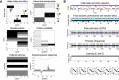
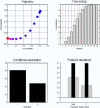
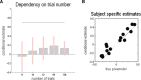
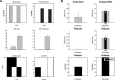
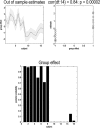
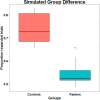
Similar articles
-
Revisiting the importance of model fitting for model-based fMRI: It does matter in computational psychiatry.PLoS Comput Biol. 2021 Feb 9;17(2):e1008738. doi: 10.1371/journal.pcbi.1008738. eCollection 2021 Feb. PLoS Comput Biol. 2021. PMID: 33561125 Free PMC article.
-
Computational approaches to psychiatry.Curr Opin Neurobiol. 2014 Apr;25:85-92. doi: 10.1016/j.conb.2013.12.007. Epub 2013 Dec 29. Curr Opin Neurobiol. 2014. PMID: 24709605 Review.
-
Modeling subjective belief states in computational psychiatry: interoceptive inference as a candidate framework.Psychopharmacology (Berl). 2019 Aug;236(8):2405-2412. doi: 10.1007/s00213-019-05300-5. Epub 2019 Jun 22. Psychopharmacology (Berl). 2019. PMID: 31230144 Free PMC article.
-
Computational psychiatry: from synapses to sentience.Mol Psychiatry. 2023 Jan;28(1):256-268. doi: 10.1038/s41380-022-01743-z. Epub 2022 Sep 2. Mol Psychiatry. 2023. PMID: 36056173 Free PMC article. Review.
-
Active Inference in OpenAI Gym: A Paradigm for Computational Investigations Into Psychiatric Illness.Biol Psychiatry Cogn Neurosci Neuroimaging. 2018 Sep;3(9):809-818. doi: 10.1016/j.bpsc.2018.06.010. Epub 2018 Jul 10. Biol Psychiatry Cogn Neurosci Neuroimaging. 2018. PMID: 30082215
Cited by
-
Prefrontal Computation as Active Inference.Cereb Cortex. 2020 Mar 21;30(2):682-695. doi: 10.1093/cercor/bhz118. Cereb Cortex. 2020. PMID: 31298270 Free PMC article.
-
An Active Inference Approach to Modeling Structure Learning: Concept Learning as an Example Case.Front Comput Neurosci. 2020 May 19;14:41. doi: 10.3389/fncom.2020.00041. eCollection 2020. Front Comput Neurosci. 2020. PMID: 32508611 Free PMC article.
-
Greater decision uncertainty characterizes a transdiagnostic patient sample during approach-avoidance conflict: a computational modelling approach.J Psychiatry Neurosci. 2021 Jan 4;46(1):E74-E87. doi: 10.1503/jpn.200032. J Psychiatry Neurosci. 2021. PMID: 33119490 Free PMC article.
-
Future climates: Markov blankets and active inference in the biosphere.J R Soc Interface. 2020 Nov;17(172):20200503. doi: 10.1098/rsif.2020.0503. Epub 2020 Nov 25. J R Soc Interface. 2020. PMID: 33234063 Free PMC article.
-
Active Inference, Epistemic Value, and Uncertainty in Conceptual Disorganization in First-Episode Schizophrenia.Schizophr Bull. 2023 Mar 22;49(Suppl_2):S115-S124. doi: 10.1093/schbul/sbac125. Schizophr Bull. 2023. PMID: 36946528 Free PMC article.
References
-
- Attias H (2000) A variational Bayesian framework for graphical models . In: Neural information processing systems 12 (Solla SA, Leen TK, Müller K, eds). La Jolla, CA: Neural Information Processing Systems Foundation, Inc.
-
- Beal MJ (2003) Variational algorithms for approximate Bayesian inference. Masters thesis, University of Cambridge, UK.
Publication types
MeSH terms
Grants and funding
LinkOut - more resources
Full Text Sources
Other Literature Sources
Medical