Scene Construction, Visual Foraging, and Active Inference
- PMID: 27378899
- PMCID: PMC4906014
- DOI: 10.3389/fncom.2016.00056
Scene Construction, Visual Foraging, and Active Inference
Abstract
This paper describes an active inference scheme for visual searches and the perceptual synthesis entailed by scene construction. Active inference assumes that perception and action minimize variational free energy, where actions are selected to minimize the free energy expected in the future. This assumption generalizes risk-sensitive control and expected utility theory to include epistemic value; namely, the value (or salience) of information inherent in resolving uncertainty about the causes of ambiguous cues or outcomes. Here, we apply active inference to saccadic searches of a visual scene. We consider the (difficult) problem of categorizing a scene, based on the spatial relationship among visual objects where, crucially, visual cues are sampled myopically through a sequence of saccadic eye movements. This means that evidence for competing hypotheses about the scene has to be accumulated sequentially, calling upon both prediction (planning) and postdiction (memory). Our aim is to highlight some simple but fundamental aspects of the requisite functional anatomy; namely, the link between approximate Bayesian inference under mean field assumptions and functional segregation in the visual cortex. This link rests upon the (neurobiologically plausible) process theory that accompanies the normative formulation of active inference for Markov decision processes. In future work, we hope to use this scheme to model empirical saccadic searches and identify the prior beliefs that underwrite intersubject variability in the way people forage for information in visual scenes (e.g., in schizophrenia).
Keywords: Bayesian inference; active inference; epistemic value; free energy; information gain; salience; scene construction; visual search.
Figures
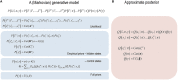
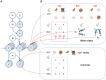
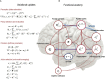
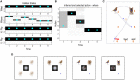
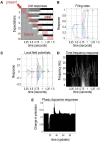
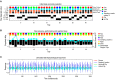
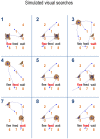
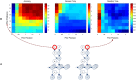
Similar articles
-
Active inference and learning.Neurosci Biobehav Rev. 2016 Sep;68:862-879. doi: 10.1016/j.neubiorev.2016.06.022. Epub 2016 Jun 29. Neurosci Biobehav Rev. 2016. PMID: 27375276 Free PMC article. Review.
-
Deep Active Inference and Scene Construction.Front Artif Intell. 2020 Oct 28;3:509354. doi: 10.3389/frai.2020.509354. eCollection 2020. Front Artif Intell. 2020. PMID: 33733195 Free PMC article.
-
Human visual exploration reduces uncertainty about the sensed world.PLoS One. 2018 Jan 5;13(1):e0190429. doi: 10.1371/journal.pone.0190429. eCollection 2018. PLoS One. 2018. PMID: 29304087 Free PMC article.
-
Perceptions as hypotheses: saccades as experiments.Front Psychol. 2012 May 28;3:151. doi: 10.3389/fpsyg.2012.00151. eCollection 2012. Front Psychol. 2012. PMID: 22654776 Free PMC article.
-
The active construction of the visual world.Neuropsychologia. 2017 Sep;104:92-101. doi: 10.1016/j.neuropsychologia.2017.08.003. Epub 2017 Aug 3. Neuropsychologia. 2017. PMID: 28782543 Free PMC article. Review.
Cited by
-
Generative models, linguistic communication and active inference.Neurosci Biobehav Rev. 2020 Nov;118:42-64. doi: 10.1016/j.neubiorev.2020.07.005. Epub 2020 Jul 17. Neurosci Biobehav Rev. 2020. PMID: 32687883 Free PMC article. Review.
-
The Thalamus as a Blackboard for Perception and Planning.Front Behav Neurosci. 2021 Mar 1;15:633872. doi: 10.3389/fnbeh.2021.633872. eCollection 2021. Front Behav Neurosci. 2021. PMID: 33732119 Free PMC article.
-
Active inference and learning.Neurosci Biobehav Rev. 2016 Sep;68:862-879. doi: 10.1016/j.neubiorev.2016.06.022. Epub 2016 Jun 29. Neurosci Biobehav Rev. 2016. PMID: 27375276 Free PMC article. Review.
-
Forgetting ourselves in flow: an active inference account of flow states and how we experience ourselves within them.Front Psychol. 2024 Jun 3;15:1354719. doi: 10.3389/fpsyg.2024.1354719. eCollection 2024. Front Psychol. 2024. PMID: 38887627 Free PMC article.
-
Neural Dynamics under Active Inference: Plausibility and Efficiency of Information Processing.Entropy (Basel). 2021 Apr 12;23(4):454. doi: 10.3390/e23040454. Entropy (Basel). 2021. PMID: 33921298 Free PMC article.
References
-
- Andreopoulos A., Tsotsos J. (2013). A computational learning theory of active object recognition under uncertainty. Int. J. Comput. Vis. 101, 95–142. 10.1007/s11263-012-0551-6 - DOI
-
- Barlow H. (1961). Possible principles underlying the transformations of sensory messages, in Sensory Communication, ed Rosenblith W. (Cambridge, MA: MIT Press; ), 217–234.
-
- Beal M. J. (2003). Variational Algorithms for Approximate Bayesian Inference. Ph.D. thesis, University College London.
Grants and funding
LinkOut - more resources
Full Text Sources
Other Literature Sources