Spike-Based Bayesian-Hebbian Learning of Temporal Sequences
- PMID: 27213810
- PMCID: PMC4877102
- DOI: 10.1371/journal.pcbi.1004954
Spike-Based Bayesian-Hebbian Learning of Temporal Sequences
Abstract
Many cognitive and motor functions are enabled by the temporal representation and processing of stimuli, but it remains an open issue how neocortical microcircuits can reliably encode and replay such sequences of information. To better understand this, a modular attractor memory network is proposed in which meta-stable sequential attractor transitions are learned through changes to synaptic weights and intrinsic excitabilities via the spike-based Bayesian Confidence Propagation Neural Network (BCPNN) learning rule. We find that the formation of distributed memories, embodied by increased periods of firing in pools of excitatory neurons, together with asymmetrical associations between these distinct network states, can be acquired through plasticity. The model's feasibility is demonstrated using simulations of adaptive exponential integrate-and-fire model neurons (AdEx). We show that the learning and speed of sequence replay depends on a confluence of biophysically relevant parameters including stimulus duration, level of background noise, ratio of synaptic currents, and strengths of short-term depression and adaptation. Moreover, sequence elements are shown to flexibly participate multiple times in the sequence, suggesting that spiking attractor networks of this type can support an efficient combinatorial code. The model provides a principled approach towards understanding how multiple interacting plasticity mechanisms can coordinate hetero-associative learning in unison.
Conflict of interest statement
The authors have declared that no competing interests exist.
Figures
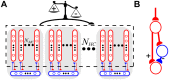
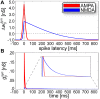
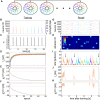
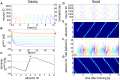
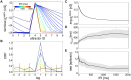
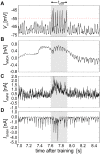
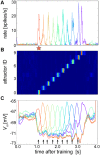
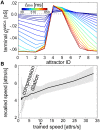
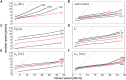
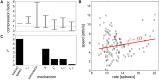
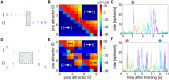
Similar articles
-
Probabilistic associative learning suffices for learning the temporal structure of multiple sequences.PLoS One. 2019 Aug 1;14(8):e0220161. doi: 10.1371/journal.pone.0220161. eCollection 2019. PLoS One. 2019. PMID: 31369571 Free PMC article.
-
Online Learning and Memory of Neural Trajectory Replays for Prefrontal Persistent and Dynamic Representations in the Irregular Asynchronous State.Front Neural Circuits. 2021 Jul 8;15:648538. doi: 10.3389/fncir.2021.648538. eCollection 2021. Front Neural Circuits. 2021. PMID: 34305535 Free PMC article.
-
Sequence learning, prediction, and replay in networks of spiking neurons.PLoS Comput Biol. 2022 Jun 21;18(6):e1010233. doi: 10.1371/journal.pcbi.1010233. eCollection 2022 Jun. PLoS Comput Biol. 2022. PMID: 35727857 Free PMC article.
-
Modelling the formation of working memory with networks of integrate-and-fire neurons connected by plastic synapses.J Physiol Paris. 2003 Jul-Nov;97(4-6):659-81. doi: 10.1016/j.jphysparis.2004.01.021. J Physiol Paris. 2003. PMID: 15242673 Review.
-
Predictive learning of temporal sequences in recurrent neocortical circuits.Novartis Found Symp. 2001;239:208-29; discussion 229-40. doi: 10.1002/0470846674.ch16. Novartis Found Symp. 2001. PMID: 11529313 Review.
Cited by
-
Traces of semantization - from episodic to semantic memory in a spiking cortical network model.eNeuro. 2022 Jul 8;9(4):ENEURO.0062-22.2022. doi: 10.1523/ENEURO.0062-22.2022. Online ahead of print. eNeuro. 2022. PMID: 35803714 Free PMC article.
-
Temporal-Sequential Learning With a Brain-Inspired Spiking Neural Network and Its Application to Musical Memory.Front Comput Neurosci. 2020 Jul 2;14:51. doi: 10.3389/fncom.2020.00051. eCollection 2020. Front Comput Neurosci. 2020. PMID: 32714173 Free PMC article.
-
An Indexing Theory for Working Memory Based on Fast Hebbian Plasticity.eNeuro. 2020 Apr 23;7(2):ENEURO.0374-19.2020. doi: 10.1523/ENEURO.0374-19.2020. Print 2020 Mar/Apr. eNeuro. 2020. PMID: 32127347 Free PMC article.
-
Learning multiple variable-speed sequences in striatum via cortical tutoring.Elife. 2017 May 8;6:e26084. doi: 10.7554/eLife.26084. Elife. 2017. PMID: 28481200 Free PMC article.
-
Learning precise spatiotemporal sequences via biophysically realistic learning rules in a modular, spiking network.Elife. 2021 Mar 18;10:e63751. doi: 10.7554/eLife.63751. Elife. 2021. PMID: 33734085 Free PMC article.
References
Publication types
MeSH terms
Substances
Grants and funding
LinkOut - more resources
Full Text Sources
Other Literature Sources