Dopamine, reward learning, and active inference
- PMID: 26581305
- PMCID: PMC4631836
- DOI: 10.3389/fncom.2015.00136
Dopamine, reward learning, and active inference
Abstract
Temporal difference learning models propose phasic dopamine signaling encodes reward prediction errors that drive learning. This is supported by studies where optogenetic stimulation of dopamine neurons can stand in lieu of actual reward. Nevertheless, a large body of data also shows that dopamine is not necessary for learning, and that dopamine depletion primarily affects task performance. We offer a resolution to this paradox based on an hypothesis that dopamine encodes the precision of beliefs about alternative actions, and thus controls the outcome-sensitivity of behavior. We extend an active inference scheme for solving Markov decision processes to include learning, and show that simulated dopamine dynamics strongly resemble those actually observed during instrumental conditioning. Furthermore, simulated dopamine depletion impairs performance but spares learning, while simulated excitation of dopamine neurons drives reward learning, through aberrant inference about outcome states. Our formal approach provides a novel and parsimonious reconciliation of apparently divergent experimental findings.
Keywords: active inference; dopamine; incentive salience; instrumental conditioning; learning; reward; reward learning; variational inference.
Figures
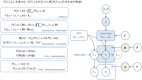
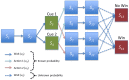
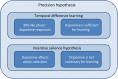
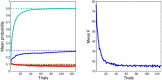
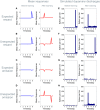
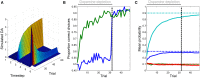
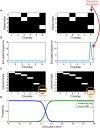
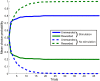
Similar articles
-
Compromised NMDA/Glutamate Receptor Expression in Dopaminergic Neurons Impairs Instrumental Learning, But Not Pavlovian Goal Tracking or Sign Tracking.eNeuro. 2015 Jun 10;2(3):ENEURO.0040-14.2015. doi: 10.1523/ENEURO.0040-14.2015. eCollection 2015 May-Jun. eNeuro. 2015. PMID: 26464985 Free PMC article.
-
A neural network model with dopamine-like reinforcement signal that learns a spatial delayed response task.Neuroscience. 1999;91(3):871-90. doi: 10.1016/s0306-4522(98)00697-6. Neuroscience. 1999. PMID: 10391468
-
Toward isolating the role of dopamine in the acquisition of incentive salience attribution.Neuropharmacology. 2016 Oct;109:320-331. doi: 10.1016/j.neuropharm.2016.06.028. Epub 2016 Jun 28. Neuropharmacology. 2016. PMID: 27371135 Free PMC article.
-
Dopamine signals as temporal difference errors: recent advances.Curr Opin Neurobiol. 2021 Apr;67:95-105. doi: 10.1016/j.conb.2020.08.014. Epub 2020 Nov 10. Curr Opin Neurobiol. 2021. PMID: 33186815 Free PMC article. Review.
-
Predictive reward signal of dopamine neurons.J Neurophysiol. 1998 Jul;80(1):1-27. doi: 10.1152/jn.1998.80.1.1. J Neurophysiol. 1998. PMID: 9658025 Review.
Cited by
-
How mood tunes prediction: a neurophenomenological account of mood and its disturbance in major depression.Neurosci Conscious. 2020 Jun 2;2020(1):niaa003. doi: 10.1093/nc/niaa003. eCollection 2020. Neurosci Conscious. 2020. PMID: 32818063 Free PMC article.
-
In the Body's Eye: The computational anatomy of interoceptive inference.PLoS Comput Biol. 2022 Sep 13;18(9):e1010490. doi: 10.1371/journal.pcbi.1010490. eCollection 2022 Sep. PLoS Comput Biol. 2022. PMID: 36099315 Free PMC article.
-
An Integrated theory of false insights and beliefs under psychedelics.Commun Psychol. 2024 Aug 1;2(1):69. doi: 10.1038/s44271-024-00120-6. Commun Psychol. 2024. PMID: 39242747 Free PMC article. Review.
-
Generalised free energy and active inference.Biol Cybern. 2019 Dec;113(5-6):495-513. doi: 10.1007/s00422-019-00805-w. Epub 2019 Sep 27. Biol Cybern. 2019. PMID: 31562544 Free PMC article.
-
Variability in Action Selection Relates to Striatal Dopamine 2/3 Receptor Availability in Humans: A PET Neuroimaging Study Using Reinforcement Learning and Active Inference Models.Cereb Cortex. 2020 May 18;30(6):3573-3589. doi: 10.1093/cercor/bhz327. Cereb Cortex. 2020. PMID: 32083297 Free PMC article.
References
-
- Beal M. J. (2003). Variational Algorithms for Approximate Bayesian Inference. Ph.D. Thesis, University College London.
Grants and funding
LinkOut - more resources
Full Text Sources
Other Literature Sources