Computer-aided classification of lung nodules on computed tomography images via deep learning technique
- PMID: 26346558
- PMCID: PMC4531007
- DOI: 10.2147/OTT.S80733
Computer-aided classification of lung nodules on computed tomography images via deep learning technique
Abstract
Lung cancer has a poor prognosis when not diagnosed early and unresectable lesions are present. The management of small lung nodules noted on computed tomography scan is controversial due to uncertain tumor characteristics. A conventional computer-aided diagnosis (CAD) scheme requires several image processing and pattern recognition steps to accomplish a quantitative tumor differentiation result. In such an ad hoc image analysis pipeline, every step depends heavily on the performance of the previous step. Accordingly, tuning of classification performance in a conventional CAD scheme is very complicated and arduous. Deep learning techniques, on the other hand, have the intrinsic advantage of an automatic exploitation feature and tuning of performance in a seamless fashion. In this study, we attempted to simplify the image analysis pipeline of conventional CAD with deep learning techniques. Specifically, we introduced models of a deep belief network and a convolutional neural network in the context of nodule classification in computed tomography images. Two baseline methods with feature computing steps were implemented for comparison. The experimental results suggest that deep learning methods could achieve better discriminative results and hold promise in the CAD application domain.
Keywords: convolutional neural network; deep belief network; deep learning; nodule classification.
Figures
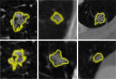
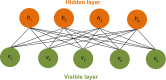
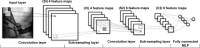
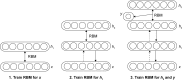
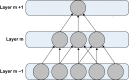
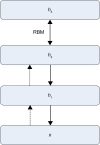
Similar articles
-
Appraisal of Deep-Learning Techniques on Computer-Aided Lung Cancer Diagnosis with Computed Tomography Screening.J Med Phys. 2020 Apr-Jun;45(2):98-106. doi: 10.4103/jmp.JMP_101_19. Epub 2020 Jul 20. J Med Phys. 2020. PMID: 32831492 Free PMC article.
-
Automatic lung nodule detection using a 3D deep convolutional neural network combined with a multi-scale prediction strategy in chest CTs.Comput Biol Med. 2018 Dec 1;103:220-231. doi: 10.1016/j.compbiomed.2018.10.011. Epub 2018 Oct 12. Comput Biol Med. 2018. PMID: 30390571
-
CAD system for lung nodule detection using deep learning with CNN.Med Biol Eng Comput. 2022 Jan;60(1):221-228. doi: 10.1007/s11517-021-02462-3. Epub 2021 Nov 22. Med Biol Eng Comput. 2022. PMID: 34811644
-
Deep learning-based CAD schemes for the detection and classification of lung nodules from CT images: A survey.J Xray Sci Technol. 2020;28(4):591-617. doi: 10.3233/XST-200660. J Xray Sci Technol. 2020. PMID: 32568165 Review.
-
A survey of computer-aided diagnosis of lung nodules from CT scans using deep learning.Comput Biol Med. 2021 Oct;137:104806. doi: 10.1016/j.compbiomed.2021.104806. Epub 2021 Aug 25. Comput Biol Med. 2021. PMID: 34461501 Review.
Cited by
-
Cancer Diagnosis Using Deep Learning: A Bibliographic Review.Cancers (Basel). 2019 Aug 23;11(9):1235. doi: 10.3390/cancers11091235. Cancers (Basel). 2019. PMID: 31450799 Free PMC article. Review.
-
Comparative effectiveness of convolutional neural network (CNN) and recurrent neural network (RNN) architectures for radiology text report classification.Artif Intell Med. 2019 Jun;97:79-88. doi: 10.1016/j.artmed.2018.11.004. Epub 2018 Nov 23. Artif Intell Med. 2019. PMID: 30477892 Free PMC article.
-
Classification of CT Scan Images of Lungs Using Deep Convolutional Neural Network with External Shape-Based Features.J Digit Imaging. 2020 Feb;33(1):252-261. doi: 10.1007/s10278-019-00245-9. J Digit Imaging. 2020. PMID: 31243590 Free PMC article.
-
Enhanced lung image segmentation using deep learning.Neural Comput Appl. 2022 Jan 3:1-15. doi: 10.1007/s00521-021-06719-8. Online ahead of print. Neural Comput Appl. 2022. PMID: 35002086 Free PMC article.
-
Artificial intelligence detection of distal radius fractures: a comparison between the convolutional neural network and professional assessments.Acta Orthop. 2019 Aug;90(4):394-400. doi: 10.1080/17453674.2019.1600125. Epub 2019 Apr 3. Acta Orthop. 2019. PMID: 30942136 Free PMC article.
References
LinkOut - more resources
Full Text Sources
Other Literature Sources
Miscellaneous