Teaching a Machine to Feel Postoperative Pain: Combining High-Dimensional Clinical Data with Machine Learning Algorithms to Forecast Acute Postoperative Pain
- PMID: 26031220
- PMCID: PMC4504764
- DOI: 10.1111/pme.12713
Teaching a Machine to Feel Postoperative Pain: Combining High-Dimensional Clinical Data with Machine Learning Algorithms to Forecast Acute Postoperative Pain
Abstract
Background: Given their ability to process highly dimensional datasets with hundreds of variables, machine learning algorithms may offer one solution to the vexing challenge of predicting postoperative pain.
Methods: Here, we report on the application of machine learning algorithms to predict postoperative pain outcomes in a retrospective cohort of 8,071 surgical patients using 796 clinical variables. Five algorithms were compared in terms of their ability to forecast moderate to severe postoperative pain: Least Absolute Shrinkage and Selection Operator (LASSO), gradient-boosted decision tree, support vector machine, neural network, and k-nearest neighbor (k-NN), with logistic regression included for baseline comparison.
Results: In forecasting moderate to severe postoperative pain for postoperative day (POD) 1, the LASSO algorithm, using all 796 variables, had the highest accuracy with an area under the receiver-operating curve (ROC) of 0.704. Next, the gradient-boosted decision tree had an ROC of 0.665 and the k-NN algorithm had an ROC of 0.643. For POD 3, the LASSO algorithm, using all variables, again had the highest accuracy, with an ROC of 0.727. Logistic regression had a lower ROC of 0.5 for predicting pain outcomes on POD 1 and 3.
Conclusions: Machine learning algorithms, when combined with complex and heterogeneous data from electronic medical record systems, can forecast acute postoperative pain outcomes with accuracies similar to methods that rely only on variables specifically collected for pain outcome prediction.
Keywords: Algorithm; Machine Learning; Pain Prediction; Postoperative Pain.
Wiley Periodicals, Inc.
Conflict of interest statement
The authors have no conflicts of interests to report.
Figures

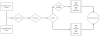
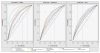
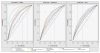
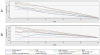
Similar articles
-
Predicting hospitalization following psychiatric crisis care using machine learning.BMC Med Inform Decis Mak. 2020 Dec 10;20(1):332. doi: 10.1186/s12911-020-01361-1. BMC Med Inform Decis Mak. 2020. PMID: 33302948 Free PMC article.
-
Machine learning applications for the prediction of surgical site infection in neurological operations.Neurosurg Focus. 2019 Aug 1;47(2):E7. doi: 10.3171/2019.5.FOCUS19241. Neurosurg Focus. 2019. PMID: 31370028
-
Prediction of Allogeneic Hematopoietic Stem-Cell Transplantation Mortality 100 Days After Transplantation Using a Machine Learning Algorithm: A European Group for Blood and Marrow Transplantation Acute Leukemia Working Party Retrospective Data Mining Study.J Clin Oncol. 2015 Oct 1;33(28):3144-51. doi: 10.1200/JCO.2014.59.1339. Epub 2015 Aug 3. J Clin Oncol. 2015. PMID: 26240227
-
Review of Medical Decision Support and Machine-Learning Methods.Vet Pathol. 2019 Jul;56(4):512-525. doi: 10.1177/0300985819829524. Epub 2019 Mar 13. Vet Pathol. 2019. PMID: 30866728 Review.
-
An extensive experimental survey of regression methods.Neural Netw. 2019 Mar;111:11-34. doi: 10.1016/j.neunet.2018.12.010. Epub 2018 Dec 21. Neural Netw. 2019. PMID: 30654138 Review.
Cited by
-
Advancing Symptom Science Through Symptom Cluster Research: Expert Panel Proceedings and Recommendations.J Natl Cancer Inst. 2017 Jan 24;109(4):djw253. doi: 10.1093/jnci/djw253. Print 2017 Apr. J Natl Cancer Inst. 2017. PMID: 28119347 Free PMC article. Review.
-
Use of Mobile Health Apps and Wearable Technology to Assess Changes and Predict Pain During Treatment of Acute Pain in Sickle Cell Disease: Feasibility Study.JMIR Mhealth Uhealth. 2019 Dec 2;7(12):e13671. doi: 10.2196/13671. JMIR Mhealth Uhealth. 2019. PMID: 31789599 Free PMC article.
-
Development of a random forest model for hypotension prediction after anesthesia induction for cardiac surgery.World J Clin Cases. 2021 Oct 16;9(29):8729-8739. doi: 10.12998/wjcc.v9.i29.8729. World J Clin Cases. 2021. PMID: 34734051 Free PMC article.
-
SCAMPs for Multimodal Post-Operative Analgesia: A Concept to Standardize and Individualize Care.Curr Pain Headache Rep. 2017 Jan;21(1):5. doi: 10.1007/s11916-017-0603-2. Curr Pain Headache Rep. 2017. PMID: 28150136 Review.
-
Predicting Postoperative Pain and Opioid Use with Machine Learning Applied to Longitudinal Electronic Health Record and Wearable Data.Appl Clin Inform. 2024 May;15(3):569-582. doi: 10.1055/a-2321-0397. Epub 2024 May 7. Appl Clin Inform. 2024. PMID: 38714212
References
-
- Apfelbaum JL, Chen C, Mehta SS, Gan ATJ. Postoperative pain experience: Results from a National survey suggest postoperative pain continues to be undermanaged. Anesth Analg. 2003;97:534–40. - PubMed
-
- Kehlet H, Jensen TS, Woolf CJ. Persistent postsurgical pain: Risk factors and prevention. Lancet. 2006;367:1618–5. - PubMed
-
- Buvanendran A, Kroin JS. Multimodal analgesia for controlling acute postoperative pain. Curr Opin Anaesthesiol. 2009;22:588–93. - PubMed
-
- Katz J, Clarke H, Seltzer Z. Preventive analgesia. Anesth Analg. 2011;113:1242–53. - PubMed
-
- Ip HYV, Abrishami A, Peng PWH, Wong J, Chung F. Predictors of postoperative pain and analgesic consumption: A qualitative systematic review. Anesthesiology. 2009;111:657–77. - PubMed
Publication types
MeSH terms
Grants and funding
LinkOut - more resources
Full Text Sources
Other Literature Sources