Neural modularity helps organisms evolve to learn new skills without forgetting old skills
- PMID: 25837826
- PMCID: PMC4383335
- DOI: 10.1371/journal.pcbi.1004128
Neural modularity helps organisms evolve to learn new skills without forgetting old skills
Abstract
A long-standing goal in artificial intelligence is creating agents that can learn a variety of different skills for different problems. In the artificial intelligence subfield of neural networks, a barrier to that goal is that when agents learn a new skill they typically do so by losing previously acquired skills, a problem called catastrophic forgetting. That occurs because, to learn the new task, neural learning algorithms change connections that encode previously acquired skills. How networks are organized critically affects their learning dynamics. In this paper, we test whether catastrophic forgetting can be reduced by evolving modular neural networks. Modularity intuitively should reduce learning interference between tasks by separating functionality into physically distinct modules in which learning can be selectively turned on or off. Modularity can further improve learning by having a reinforcement learning module separate from sensory processing modules, allowing learning to happen only in response to a positive or negative reward. In this paper, learning takes place via neuromodulation, which allows agents to selectively change the rate of learning for each neural connection based on environmental stimuli (e.g. to alter learning in specific locations based on the task at hand). To produce modularity, we evolve neural networks with a cost for neural connections. We show that this connection cost technique causes modularity, confirming a previous result, and that such sparsely connected, modular networks have higher overall performance because they learn new skills faster while retaining old skills more and because they have a separate reinforcement learning module. Our results suggest (1) that encouraging modularity in neural networks may help us overcome the long-standing barrier of networks that cannot learn new skills without forgetting old ones, and (2) that one benefit of the modularity ubiquitous in the brains of natural animals might be to alleviate the problem of catastrophic forgetting.
Conflict of interest statement
The authors have declared that no competing interests exist.
Figures
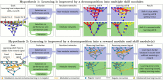
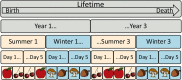
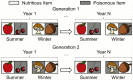
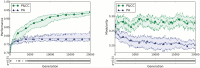
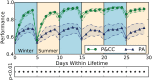
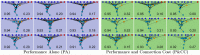
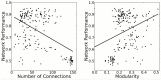
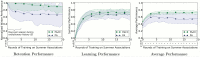
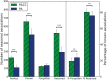
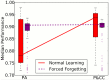
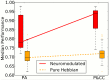
Similar articles
-
Diffusion-based neuromodulation can eliminate catastrophic forgetting in simple neural networks.PLoS One. 2017 Nov 16;12(11):e0187736. doi: 10.1371/journal.pone.0187736. eCollection 2017. PLoS One. 2017. PMID: 29145413 Free PMC article.
-
Tackling learning intractability through topological organization and regulation of cortical networks.IEEE Trans Neural Netw Learn Syst. 2012 Apr;23(4):552-64. doi: 10.1109/TNNLS.2011.2178311. IEEE Trans Neural Netw Learn Syst. 2012. PMID: 24805039
-
Alleviating catastrophic forgetting using context-dependent gating and synaptic stabilization.Proc Natl Acad Sci U S A. 2018 Oct 30;115(44):E10467-E10475. doi: 10.1073/pnas.1803839115. Epub 2018 Oct 12. Proc Natl Acad Sci U S A. 2018. PMID: 30315147 Free PMC article.
-
Born to learn: The inspiration, progress, and future of evolved plastic artificial neural networks.Neural Netw. 2018 Dec;108:48-67. doi: 10.1016/j.neunet.2018.07.013. Epub 2018 Aug 7. Neural Netw. 2018. PMID: 30142505 Review.
-
Reward-dependent learning in neuronal networks for planning and decision making.Prog Brain Res. 2000;126:217-29. doi: 10.1016/S0079-6123(00)26016-0. Prog Brain Res. 2000. PMID: 11105649 Review.
Cited by
-
Emergence and reconfiguration of modular structure for artificial neural networks during continual familiarity detection.Sci Adv. 2024 Jul 26;10(30):eadm8430. doi: 10.1126/sciadv.adm8430. Epub 2024 Jul 26. Sci Adv. 2024. PMID: 39058783 Free PMC article.
-
Network Brain-Computer Interface (nBCI): An Alternative Approach for Cognitive Prosthetics.Front Neurosci. 2018 Nov 1;12:790. doi: 10.3389/fnins.2018.00790. eCollection 2018. Front Neurosci. 2018. PMID: 30443203 Free PMC article.
-
Brain state flexibility accompanies motor-skill acquisition.Neuroimage. 2018 May 1;171:135-147. doi: 10.1016/j.neuroimage.2017.12.093. Epub 2018 Jan 6. Neuroimage. 2018. PMID: 29309897 Free PMC article.
-
Evolving autonomous learning in cognitive networks.Sci Rep. 2017 Dec 1;7(1):16712. doi: 10.1038/s41598-017-16548-2. Sci Rep. 2017. PMID: 29196623 Free PMC article.
-
Brain connectivity meets reservoir computing.PLoS Comput Biol. 2022 Nov 16;18(11):e1010639. doi: 10.1371/journal.pcbi.1010639. eCollection 2022 Nov. PLoS Comput Biol. 2022. PMID: 36383563 Free PMC article.
References
-
- Haykin SS (2009) Neural networks and learning machines. New York: Prentice Hall, 3 edition.
-
- Floreano D, Mattiussi C (2008) Bio-inspired artificial intelligence: theories, methods, and technologies. The MIT Press, 659 pp.
Publication types
MeSH terms
Associated data
Grants and funding
LinkOut - more resources
Full Text Sources
Other Literature Sources