Clinical decision support systems for improving diagnostic accuracy and achieving precision medicine
- PMID: 25834725
- PMCID: PMC4381462
- DOI: 10.1186/s13336-015-0019-3
Clinical decision support systems for improving diagnostic accuracy and achieving precision medicine
Abstract
As research laboratories and clinics collaborate to achieve precision medicine, both communities are required to understand mandated electronic health/medical record (EHR/EMR) initiatives that will be fully implemented in all clinics in the United States by 2015. Stakeholders will need to evaluate current record keeping practices and optimize and standardize methodologies to capture nearly all information in digital format. Collaborative efforts from academic and industry sectors are crucial to achieving higher efficacy in patient care while minimizing costs. Currently existing digitized data and information are present in multiple formats and are largely unstructured. In the absence of a universally accepted management system, departments and institutions continue to generate silos of information. As a result, invaluable and newly discovered knowledge is difficult to access. To accelerate biomedical research and reduce healthcare costs, clinical and bioinformatics systems must employ common data elements to create structured annotation forms enabling laboratories and clinics to capture sharable data in real time. Conversion of these datasets to knowable information should be a routine institutionalized process. New scientific knowledge and clinical discoveries can be shared via integrated knowledge environments defined by flexible data models and extensive use of standards, ontologies, vocabularies, and thesauri. In the clinical setting, aggregated knowledge must be displayed in user-friendly formats so that physicians, non-technical laboratory personnel, nurses, data/research coordinators, and end-users can enter data, access information, and understand the output. The effort to connect astronomical numbers of data points, including '-omics'-based molecular data, individual genome sequences, experimental data, patient clinical phenotypes, and follow-up data is a monumental task. Roadblocks to this vision of integration and interoperability include ethical, legal, and logistical concerns. Ensuring data security and protection of patient rights while simultaneously facilitating standardization is paramount to maintaining public support. The capabilities of supercomputing need to be applied strategically. A standardized, methodological implementation must be applied to developed artificial intelligence systems with the ability to integrate data and information into clinically relevant knowledge. Ultimately, the integration of bioinformatics and clinical data in a clinical decision support system promises precision medicine and cost effective and personalized patient care.
Keywords: Artificial intelligence; Bioinformatics; Clinical decision support system; Clinical informatics; Clinical outcome; Integrated knowledge environment; Patient care; Personalized medicine; Precision medicine; Watson.
Figures
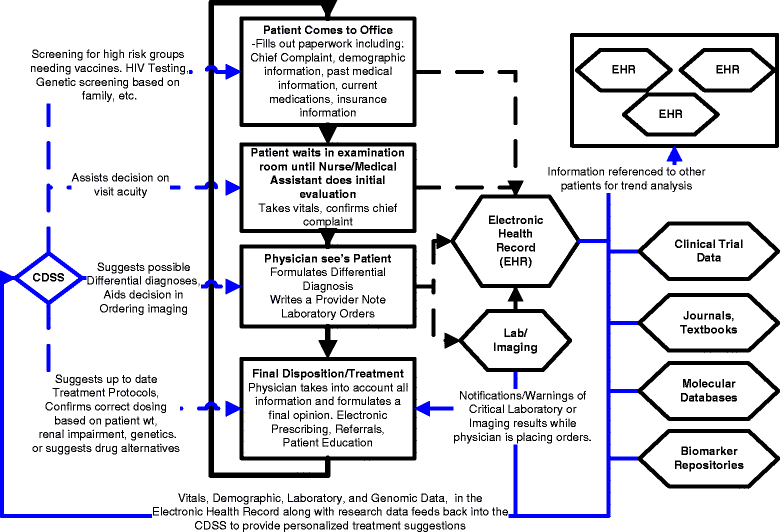
Similar articles
-
THE FUTURE OF MEDICINE, healthcare innovation through precision medicine: policy case study of Qatar.Life Sci Soc Policy. 2020 Nov 1;16(1):12. doi: 10.1186/s40504-020-00107-1. Life Sci Soc Policy. 2020. PMID: 33129349 Free PMC article. Review.
-
Precision omics data integration and analysis with interoperable ontologies and their application for COVID-19 research.Brief Funct Genomics. 2021 Jul 17;20(4):235-248. doi: 10.1093/bfgp/elab029. Brief Funct Genomics. 2021. PMID: 34159360 Free PMC article.
-
Cognitive IT-systems for big data analysis in medicine.Int J Risk Saf Med. 2015;27 Suppl 1:S108-9. doi: 10.3233/JRS-150711. Int J Risk Saf Med. 2015. PMID: 26639685
-
Data Integration for Future Medicine (DIFUTURE).Methods Inf Med. 2018 Jul;57(S 01):e57-e65. doi: 10.3414/ME17-02-0022. Epub 2018 Jul 17. Methods Inf Med. 2018. PMID: 30016812 Free PMC article.
-
Tuberculosis.In: Holmes KK, Bertozzi S, Bloom BR, Jha P, editors. Major Infectious Diseases. 3rd edition. Washington (DC): The International Bank for Reconstruction and Development / The World Bank; 2017 Nov 3. Chapter 11. In: Holmes KK, Bertozzi S, Bloom BR, Jha P, editors. Major Infectious Diseases. 3rd edition. Washington (DC): The International Bank for Reconstruction and Development / The World Bank; 2017 Nov 3. Chapter 11. PMID: 30212088 Free Books & Documents. Review.
Cited by
-
Artificial intelligence, radiomics and other horizons in body composition assessment.Quant Imaging Med Surg. 2020 Aug;10(8):1650-1660. doi: 10.21037/qims.2020.03.10. Quant Imaging Med Surg. 2020. PMID: 32742958 Free PMC article. Review.
-
FHIRChain: Applying Blockchain to Securely and Scalably Share Clinical Data.Comput Struct Biotechnol J. 2018 Jul 29;16:267-278. doi: 10.1016/j.csbj.2018.07.004. eCollection 2018. Comput Struct Biotechnol J. 2018. PMID: 30108685 Free PMC article.
-
Role of Health Information Technology in Addressing Health Disparities: Patient, Clinician, and System Perspectives.Med Care. 2019 Jun;57 Suppl 6 Suppl 2(Suppl 6 2):S115-S120. doi: 10.1097/MLR.0000000000001092. Med Care. 2019. PMID: 31095049 Free PMC article.
-
Toward Precision Healthcare: Context and Mathematical Challenges.Front Physiol. 2017 Mar 21;8:136. doi: 10.3389/fphys.2017.00136. eCollection 2017. Front Physiol. 2017. PMID: 28377724 Free PMC article.
-
A novel method using Covid-19 dataset and machine learning algorithms FOR THE MOST ACCURATE DIAGNOSIS that can be obtained in medical diagnosis.Biomed Signal Process Control. 2022 Aug;77:103836. doi: 10.1016/j.bspc.2022.103836. Epub 2022 May 30. Biomed Signal Process Control. 2022. PMID: 35663432 Free PMC article.
References
-
- “Clinical Informatics”. Home. N.p., n.d. Web. 29 Dec. 2013. <http://www.amia.org/applications-informatics/clinical-informatics>.
-
- “New Clinical Informatics Subspecialty and First Class of Diplomates Signal Pivot in Healthcare Delivery”. Home. N.p., n.d. Web. 29 Dec. 2013. <http://www.amia.org/news-and-publications/press-release/new-clinical-inf...>.
-
- “Translational Bioinformatics”. Home. N.p., n.d. Web. 29 Dec. 2013. <http://www.amia.org/applications-informatics/translational-bioinformatics>.
LinkOut - more resources
Full Text Sources
Other Literature Sources
Research Materials