Neural population coding: combining insights from microscopic and mass signals
- PMID: 25670005
- PMCID: PMC4379382
- DOI: 10.1016/j.tics.2015.01.002
Neural population coding: combining insights from microscopic and mass signals
Abstract
Behavior relies on the distributed and coordinated activity of neural populations. Population activity can be measured using multi-neuron recordings and neuroimaging. Neural recordings reveal how the heterogeneity, sparseness, timing, and correlation of population activity shape information processing in local networks, whereas neuroimaging shows how long-range coupling and brain states impact on local activity and perception. To obtain an integrated perspective on neural information processing we need to combine knowledge from both levels of investigation. We review recent progress of how neural recordings, neuroimaging, and computational approaches begin to elucidate how interactions between local neural population activity and large-scale dynamics shape the structure and coding capacity of local information representations, make them state-dependent, and control distributed populations that collectively shape behavior.
Keywords: cross-correlation; multiscale processing; neural code; neuroimaging; oscillation; relative timing; sensory processing; state-dependent coding.
Copyright © 2015 Elsevier Ltd. All rights reserved.
Figures
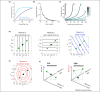
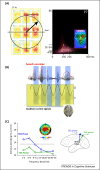
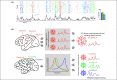
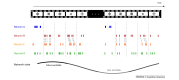
Similar articles
-
Using multi-neuron population recordings for neural prosthetics.Nat Neurosci. 2004 May;7(5):452-5. doi: 10.1038/nn1234. Nat Neurosci. 2004. PMID: 15114357 Review.
-
Multielectrode Recordings in the Somatosensory System.In: Nicolelis MAL, editor. Methods for Neural Ensemble Recordings. 2nd edition. Boca Raton (FL): CRC Press/Taylor & Francis; 2008. Chapter 6. In: Nicolelis MAL, editor. Methods for Neural Ensemble Recordings. 2nd edition. Boca Raton (FL): CRC Press/Taylor & Francis; 2008. Chapter 6. PMID: 21204443 Free Books & Documents. Review.
-
The structures and functions of correlations in neural population codes.Nat Rev Neurosci. 2022 Sep;23(9):551-567. doi: 10.1038/s41583-022-00606-4. Epub 2022 Jun 22. Nat Rev Neurosci. 2022. PMID: 35732917 Review.
-
A tensor-product-kernel framework for multiscale neural activity decoding and control.Comput Intell Neurosci. 2014;2014:870160. doi: 10.1155/2014/870160. Epub 2014 Apr 14. Comput Intell Neurosci. 2014. PMID: 24829569 Free PMC article.
-
Neural heterogeneity and efficient population codes for communication signals.J Neurophysiol. 2010 Nov;104(5):2543-55. doi: 10.1152/jn.00256.2010. Epub 2010 Jul 14. J Neurophysiol. 2010. PMID: 20631220
Cited by
-
Multiunit Frontal Eye Field Activity Codes the Visuomotor Transformation, But Not Gaze Prediction or Retrospective Target Memory, in a Delayed Saccade Task.eNeuro. 2024 Aug 8;11(8):ENEURO.0413-23.2024. doi: 10.1523/ENEURO.0413-23.2024. Print 2024 Aug. eNeuro. 2024. PMID: 39054056 Free PMC article.
-
Dynamics of Population Activity in Rat Sensory Cortex: Network Correlations Predict Anatomical Arrangement and Information Content.Front Neural Circuits. 2016 Jul 6;10:49. doi: 10.3389/fncir.2016.00049. eCollection 2016. Front Neural Circuits. 2016. PMID: 27458347 Free PMC article.
-
Phase of firing coding of learning variables across the fronto-striatal network during feature-based learning.Nat Commun. 2020 Sep 16;11(1):4669. doi: 10.1038/s41467-020-18435-3. Nat Commun. 2020. PMID: 32938940 Free PMC article.
-
The Complexity of Dynamics in Small Neural Circuits.PLoS Comput Biol. 2016 Aug 5;12(8):e1004992. doi: 10.1371/journal.pcbi.1004992. eCollection 2016 Aug. PLoS Comput Biol. 2016. PMID: 27494737 Free PMC article.
-
Modular Organization of Signal Transmission in Primate Somatosensory Cortex.Front Neuroanat. 2022 Jul 8;16:915238. doi: 10.3389/fnana.2022.915238. eCollection 2022. Front Neuroanat. 2022. PMID: 35873660 Free PMC article.
References
Publication types
MeSH terms
Grants and funding
LinkOut - more resources
Full Text Sources
Other Literature Sources