Model averaging, optimal inference, and habit formation
- PMID: 25018724
- PMCID: PMC4071291
- DOI: 10.3389/fnhum.2014.00457
Model averaging, optimal inference, and habit formation
Abstract
Postulating that the brain performs approximate Bayesian inference generates principled and empirically testable models of neuronal function-the subject of much current interest in neuroscience and related disciplines. Current formulations address inference and learning under some assumed and particular model. In reality, organisms are often faced with an additional challenge-that of determining which model or models of their environment are the best for guiding behavior. Bayesian model averaging-which says that an agent should weight the predictions of different models according to their evidence-provides a principled way to solve this problem. Importantly, because model evidence is determined by both the accuracy and complexity of the model, optimal inference requires that these be traded off against one another. This means an agent's behavior should show an equivalent balance. We hypothesize that Bayesian model averaging plays an important role in cognition, given that it is both optimal and realizable within a plausible neuronal architecture. We outline model averaging and how it might be implemented, and then explore a number of implications for brain and behavior. In particular, we propose that model averaging can explain a number of apparently suboptimal phenomena within the framework of approximate (bounded) Bayesian inference, focusing particularly upon the relationship between goal-directed and habitual behavior.
Keywords: Bayesian inference; active inference; habit; interference effect; predictive coding.
Figures
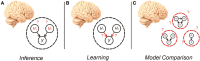
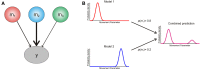
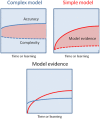
Similar articles
-
Optimal inference with suboptimal models: addiction and active Bayesian inference.Med Hypotheses. 2015 Feb;84(2):109-17. doi: 10.1016/j.mehy.2014.12.007. Epub 2014 Dec 15. Med Hypotheses. 2015. PMID: 25561321 Free PMC article.
-
The anatomy of choice: active inference and agency.Front Hum Neurosci. 2013 Sep 25;7:598. doi: 10.3389/fnhum.2013.00598. eCollection 2013. Front Hum Neurosci. 2013. PMID: 24093015 Free PMC article.
-
An Introduction to Predictive Processing Models of Perception and Decision-Making.Top Cogn Sci. 2023 Oct 29. doi: 10.1111/tops.12704. Online ahead of print. Top Cogn Sci. 2023. PMID: 37899002
-
Bayesian causal inference: A unifying neuroscience theory.Neurosci Biobehav Rev. 2022 Jun;137:104619. doi: 10.1016/j.neubiorev.2022.104619. Epub 2022 Mar 21. Neurosci Biobehav Rev. 2022. PMID: 35331819 Review.
-
Active inference and learning.Neurosci Biobehav Rev. 2016 Sep;68:862-879. doi: 10.1016/j.neubiorev.2016.06.022. Epub 2016 Jun 29. Neurosci Biobehav Rev. 2016. PMID: 27375276 Free PMC article. Review.
Cited by
-
Unifying treatments for depression: an application of the Free Energy Principle.Front Psychol. 2015 Feb 20;6:153. doi: 10.3389/fpsyg.2015.00153. eCollection 2015. Front Psychol. 2015. PMID: 25750630 Free PMC article.
-
The learning of prospective and retrospective cognitive maps within neural circuits.Neuron. 2021 Nov 17;109(22):3552-3575. doi: 10.1016/j.neuron.2021.09.034. Epub 2021 Oct 21. Neuron. 2021. PMID: 34678148 Free PMC article. Review.
-
Editorial: Habits: plasticity, learning and freedom.Front Hum Neurosci. 2015 Aug 27;9:468. doi: 10.3389/fnhum.2015.00468. eCollection 2015. Front Hum Neurosci. 2015. PMID: 26379531 Free PMC article. No abstract available.
-
A unifying Bayesian account of contextual effects in value-based choice.PLoS Comput Biol. 2017 Oct 5;13(10):e1005769. doi: 10.1371/journal.pcbi.1005769. eCollection 2017 Oct. PLoS Comput Biol. 2017. PMID: 28981514 Free PMC article.
-
The Computational Anatomy of Visual Neglect.Cereb Cortex. 2018 Feb 1;28(2):777-790. doi: 10.1093/cercor/bhx316. Cereb Cortex. 2018. PMID: 29190328 Free PMC article.
References
-
- Adams C., Dickinson A. (1981). Instrumental responding following reinforcer devaluation. Q. J. Exp. Psychol. 33, 109–121 10.1080/14640748108400816 - DOI
-
- Attias H. (2000). A variational Bayesian framework for graphical models. Adv. Neural Inf. Process. Syst. 12, 209–215
Grants and funding
LinkOut - more resources
Full Text Sources
Other Literature Sources