A review on segmentation of positron emission tomography images
- PMID: 24845019
- PMCID: PMC4060809
- DOI: 10.1016/j.compbiomed.2014.04.014
A review on segmentation of positron emission tomography images
Abstract
Positron Emission Tomography (PET), a non-invasive functional imaging method at the molecular level, images the distribution of biologically targeted radiotracers with high sensitivity. PET imaging provides detailed quantitative information about many diseases and is often used to evaluate inflammation, infection, and cancer by detecting emitted photons from a radiotracer localized to abnormal cells. In order to differentiate abnormal tissue from surrounding areas in PET images, image segmentation methods play a vital role; therefore, accurate image segmentation is often necessary for proper disease detection, diagnosis, treatment planning, and follow-ups. In this review paper, we present state-of-the-art PET image segmentation methods, as well as the recent advances in image segmentation techniques. In order to make this manuscript self-contained, we also briefly explain the fundamentals of PET imaging, the challenges of diagnostic PET image analysis, and the effects of these challenges on the segmentation results.
Keywords: Image segmentation; MRI-PET; PET; PET-CT; Review; SUV; Thresholding.
Published by Elsevier Ltd.
Figures
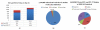
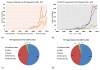
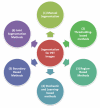
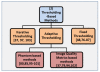
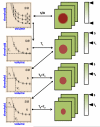
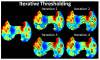
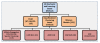
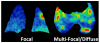
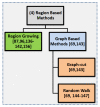
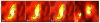
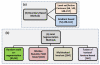
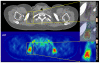
Similar articles
-
PET attenuation correction using synthetic CT from ultrashort echo-time MR imaging.J Nucl Med. 2014 Dec;55(12):2071-7. doi: 10.2967/jnumed.114.143958. Epub 2014 Nov 20. J Nucl Med. 2014. PMID: 25413135 Free PMC article.
-
PET/MRI with diagnostic MR sequences vs PET/CT in the detection of abdominal and pelvic cancer.Eur J Radiol. 2016 Apr;85(4):751-9. doi: 10.1016/j.ejrad.2016.01.010. Epub 2016 Jan 21. Eur J Radiol. 2016. PMID: 26971419
-
Real-time volume rendering visualization of dual-modality PET/CT images with interactive fuzzy thresholding segmentation.IEEE Trans Inf Technol Biomed. 2007 Mar;11(2):161-9. doi: 10.1109/titb.2006.875669. IEEE Trans Inf Technol Biomed. 2007. PMID: 17390986
-
Hybrid Positron Emission Tomography/Magnetic Resonance Imaging: Challenges, Methods, and State of the Art of Hardware Component Attenuation Correction.Invest Radiol. 2016 Oct;51(10):624-34. doi: 10.1097/RLI.0000000000000289. Invest Radiol. 2016. PMID: 27175550 Review.
-
PET-guided delineation of radiation therapy treatment volumes: a survey of image segmentation techniques.Eur J Nucl Med Mol Imaging. 2010 Nov;37(11):2165-87. doi: 10.1007/s00259-010-1423-3. Epub 2010 Mar 25. Eur J Nucl Med Mol Imaging. 2010. PMID: 20336455 Review.
Cited by
-
Investigation and optimization of PET-guided SPECT reconstructions for improved radionuclide therapy dosimetry estimates.Front Nucl Med. 2023 Jun 21;3:1124283. doi: 10.3389/fnume.2023.1124283. eCollection 2023. Front Nucl Med. 2023. PMID: 39380952 Free PMC article.
-
Segmentation of Dynamic Total-Body [18F]-FDG PET Images Using Unsupervised Clustering.Int J Biomed Imaging. 2023 Dec 5;2023:3819587. doi: 10.1155/2023/3819587. eCollection 2023. Int J Biomed Imaging. 2023. PMID: 38089593 Free PMC article.
-
Joint Tumor Segmentation in PET-CT Images Using Co-Clustering and Fusion Based on Belief Functions.IEEE Trans Image Process. 2019 Feb;28(2):755-766. doi: 10.1109/TIP.2018.2872908. Epub 2018 Oct 5. IEEE Trans Image Process. 2019. PMID: 30296224 Free PMC article.
-
Adaptive region-growing with maximum curvature strategy for tumor segmentation in 18F-FDG PET.Phys Med Biol. 2017 Jul 7;62(13):5383-5402. doi: 10.1088/1361-6560/aa6e20. Epub 2017 Jun 12. Phys Med Biol. 2017. PMID: 28604372 Free PMC article.
-
Multi-site quality and variability analysis of 3D FDG PET segmentations based on phantom and clinical image data.Med Phys. 2017 Feb;44(2):479-496. doi: 10.1002/mp.12041. Med Phys. 2017. PMID: 28205306 Free PMC article.
References
-
- Seute T, Leffers P, ten Velde G, Twijnstra A. Detection of brain metastases from small cell lung cancer. Cancer. 2008;112(8):1827–1834. - PubMed
-
- MacManus M, Nestle U, Rosenzweig K, Carrio I, Messa C, Belohlavek O, Danna M, Inoue T, Deniaud-Alexandre E, Schipani S, et al. Use of pet and pet/ct for radiation therapy planning: Iaea expert report 2006–2007. Radiotherapy and oncology. 2009;91(1):85–94. - PubMed
-
- Basu S, Kwee T, Surti S, Akin E, Yoo D, Alavi A. Fundamentals of pet and pet/ct imaging. Annals of the New York Academy of Sciences. 2011;1228(1):1–18. - PubMed
-
- Lardinois D, Weder W, Hany T, Kamel E, Korom S, Seifert B, von Schulthess G, Steinert H. Staging of non–small-cell lung cancer with integrated positron-emission tomography and computed tomography. New England Journal of Medicine. 2003;348(25):2500–2507. - PubMed
-
- Kostakoglu L, Agress H, Jr, Goldsmith S. Clinical role of fdg pet in evaluation of cancer patients. Radiographics. 2003;23(2):315–340. - PubMed
Publication types
MeSH terms
Substances
Grants and funding
LinkOut - more resources
Full Text Sources
Other Literature Sources