Segmentation of PET images for computer-aided functional quantification of tuberculosis in small animal models
- PMID: 24235292
- PMCID: PMC4196700
- DOI: 10.1109/TBME.2013.2288258
Segmentation of PET images for computer-aided functional quantification of tuberculosis in small animal models
Abstract
Pulmonary infections often cause spatially diffuse and multi-focal radiotracer uptake in positron emission tomography (PET) images, which makes accurate quantification of the disease extent challenging. Image segmentation plays a vital role in quantifying uptake due to the distributed nature of immuno-pathology and associated metabolic activities in pulmonary infection, specifically tuberculosis (TB). For this task, thresholding-based segmentation methods may be better suited over other methods; however, performance of the thresholding-based methods depend on the selection of thresholding parameters, which are often suboptimal. Several optimal thresholding techniques have been proposed in the literature, but there is currently no consensus on how to determine the optimal threshold for precise identification of spatially diffuse and multi-focal radiotracer uptake. In this study, we propose a method to select optimal thresholding levels by utilizing a novel intensity affinity metric within the affinity propagation clustering framework. We tested the proposed method against 70 longitudinal PET images of rabbits infected with TB. The overall dice similarity coefficient between the segmentation from the proposed method and two expert segmentations was found to be 91.25 ±8.01% with a sensitivity of 88.80 ±12.59% and a specificity of 96.01 ±9.20%. High accuracy and heightened efficiency of our proposed method, as compared to other PET image segmentation methods, were reported with various quantification metrics.
Figures
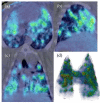
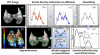
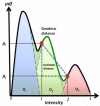
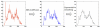
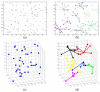
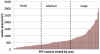
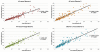
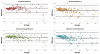
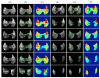
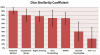
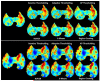
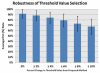
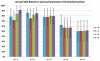
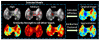
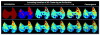
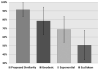
Similar articles
-
A computational pipeline for quantification of pulmonary infections in small animal models using serial PET-CT imaging.EJNMMI Res. 2013 Jul 23;3(1):55. doi: 10.1186/2191-219X-3-55. EJNMMI Res. 2013. PMID: 23879987 Free PMC article.
-
Computer-aided detection and quantification of cavitary tuberculosis from CT scans.Med Phys. 2013 Nov;40(11):113701. doi: 10.1118/1.4824979. Med Phys. 2013. PMID: 24320475 Free PMC article.
-
Reproducibility of F18-FDG PET radiomic features for different cervical tumor segmentation methods, gray-level discretization, and reconstruction algorithms.J Appl Clin Med Phys. 2017 Nov;18(6):32-48. doi: 10.1002/acm2.12170. Epub 2017 Sep 11. J Appl Clin Med Phys. 2017. PMID: 28891217 Free PMC article.
-
PET-guided delineation of radiation therapy treatment volumes: a survey of image segmentation techniques.Eur J Nucl Med Mol Imaging. 2010 Nov;37(11):2165-87. doi: 10.1007/s00259-010-1423-3. Epub 2010 Mar 25. Eur J Nucl Med Mol Imaging. 2010. PMID: 20336455 Review.
-
Intensity threshold based solid tumour segmentation method for Positron Emission Tomography (PET) images: A review.Heliyon. 2020 Oct 27;6(10):e05267. doi: 10.1016/j.heliyon.2020.e05267. eCollection 2020 Oct. Heliyon. 2020. PMID: 33163642 Free PMC article. Review.
Cited by
-
DeepPulmoTB: A benchmark dataset for multi-task learning of tuberculosis lesions in lung computerized tomography (CT).Heliyon. 2024 Feb 7;10(4):e25490. doi: 10.1016/j.heliyon.2024.e25490. eCollection 2024 Feb 29. Heliyon. 2024. PMID: 38370224 Free PMC article.
-
Joint Tumor Segmentation in PET-CT Images Using Co-Clustering and Fusion Based on Belief Functions.IEEE Trans Image Process. 2019 Feb;28(2):755-766. doi: 10.1109/TIP.2018.2872908. Epub 2018 Oct 5. IEEE Trans Image Process. 2019. PMID: 30296224 Free PMC article.
-
Does consensus contour improve robustness and accuracy in 18F-FDG PET radiomic features?EJNMMI Phys. 2024 Jun 6;11(1):48. doi: 10.1186/s40658-024-00652-0. EJNMMI Phys. 2024. PMID: 38839641 Free PMC article.
-
Automated detection of third molars and mandibular nerve by deep learning.Sci Rep. 2019 Jun 21;9(1):9007. doi: 10.1038/s41598-019-45487-3. Sci Rep. 2019. PMID: 31227772 Free PMC article.
-
Quantitative Analysis of Heterogeneous [18F]FDG Static (SUV) vs. Patlak (Ki) Whole-body PET Imaging Using Different Segmentation Methods: a Simulation Study.Mol Imaging Biol. 2019 Apr;21(2):317-327. doi: 10.1007/s11307-018-1241-8. Mol Imaging Biol. 2019. PMID: 29956119
References
-
- Kaufmann P, Camici P. Myocardial blood flow measurement by pet: Technical aspects and clinical applications. J. Nucl. Med. 2005;46(1):75–88. - PubMed
-
- Zhao B, Schwartz L, Larson S. Imaging surrogates of tumor response to therapy: Anatomic and functional biomarkers. J. Nucl. Med. 2009;50(2):239–249. - PubMed
-
- Nestle U, Kremp S, Grosu A. Practical integration of [18 F]-FDG-PET and PET-CT in the planning of radiotherapy for non-small cell lung cancer (NSCLC): The technical basis, ICRU-target volumes, problems, perspectives. Radiother. Oncol. 2006;81(2):209–225. - PubMed
Publication types
MeSH terms
Grants and funding
LinkOut - more resources
Full Text Sources
Other Literature Sources
Medical