A brain-machine interface enables bimanual arm movements in monkeys
- PMID: 24197735
- PMCID: PMC3967722
- DOI: 10.1126/scitranslmed.3006159
A brain-machine interface enables bimanual arm movements in monkeys
Abstract
Brain-machine interfaces (BMIs) are artificial systems that aim to restore sensation and movement to paralyzed patients. So far, BMIs have enabled only one arm to be moved at a time. Control of bimanual arm movements remains a major challenge. We have developed and tested a bimanual BMI that enables rhesus monkeys to control two avatar arms simultaneously. The bimanual BMI was based on the extracellular activity of 374 to 497 neurons recorded from several frontal and parietal cortical areas of both cerebral hemispheres. Cortical activity was transformed into movements of the two arms with a decoding algorithm called a fifth-order unscented Kalman filter (UKF). The UKF was trained either during a manual task performed with two joysticks or by having the monkeys passively observe the movements of avatar arms. Most cortical neurons changed their modulation patterns when both arms were engaged simultaneously. Representing the two arms jointly in a single UKF decoder resulted in improved decoding performance compared with using separate decoders for each arm. As the animals' performance in bimanual BMI control improved over time, we observed widespread plasticity in frontal and parietal cortical areas. Neuronal representation of the avatar and reach targets was enhanced with learning, whereas pairwise correlations between neurons initially increased and then decreased. These results suggest that cortical networks may assimilate the two avatar arms through BMI control. These findings should help in the design of more sophisticated BMIs capable of enabling bimanual motor control in human patients.
Conflict of interest statement
Figures
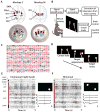
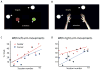
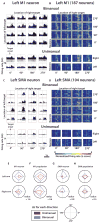
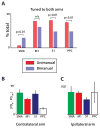
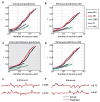
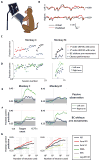
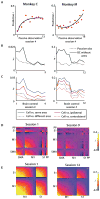
Similar articles
-
Decoding Movements from Cortical Ensemble Activity Using a Long Short-Term Memory Recurrent Network.Neural Comput. 2019 Jun;31(6):1085-1113. doi: 10.1162/neco_a_01189. Epub 2019 Apr 12. Neural Comput. 2019. PMID: 30979355
-
Decoding continuous limb movements from high-density epidural electrode arrays using custom spatial filters.J Neural Eng. 2013 Jun;10(3):036015. doi: 10.1088/1741-2560/10/3/036015. Epub 2013 Apr 23. J Neural Eng. 2013. PMID: 23611833 Free PMC article.
-
Timing of bimanual movements in human and non-human primates in relation to neuronal activity in primary motor cortex and supplementary motor area.Exp Brain Res. 2002 Oct;146(3):322-35. doi: 10.1007/s00221-002-1174-x. Epub 2002 Aug 17. Exp Brain Res. 2002. PMID: 12232689
-
Arm movements in monkeys: behavior and neurophysiology.J Comp Physiol A. 1996 Nov;179(5):603-12. doi: 10.1007/BF00216125. J Comp Physiol A. 1996. PMID: 8888576 Review.
-
Parieto-frontal coding of reaching: an integrated framework.Exp Brain Res. 1999 Dec;129(3):325-46. doi: 10.1007/s002210050902. Exp Brain Res. 1999. PMID: 10591906 Review.
Cited by
-
Large-scale recording of thalamocortical circuits: in vivo electrophysiology with the two-dimensional electronic depth control silicon probe.J Neurophysiol. 2016 Nov 1;116(5):2312-2330. doi: 10.1152/jn.00318.2016. Epub 2016 Aug 17. J Neurophysiol. 2016. PMID: 27535370 Free PMC article.
-
Affective Brain-Computer Interfaces As Enabling Technology for Responsive Psychiatric Stimulation.Brain Comput Interfaces (Abingdon). 2014 Apr 1;1(2):126-136. doi: 10.1080/2326263X.2014.912885. Brain Comput Interfaces (Abingdon). 2014. PMID: 25580443 Free PMC article.
-
Neuronal Assemblies Evidence Distributed Interactions within a Tactile Discrimination Task in Rats.Front Neural Circuits. 2018 Jan 11;11:114. doi: 10.3389/fncir.2017.00114. eCollection 2017. Front Neural Circuits. 2018. PMID: 29375324 Free PMC article.
-
Preservation of Partially Mixed Selectivity in Human Posterior Parietal Cortex across Changes in Task Context.eNeuro. 2020 Mar 12;7(2):ENEURO.0222-19.2019. doi: 10.1523/ENEURO.0222-19.2019. Print 2020 Mar/Apr. eNeuro. 2020. PMID: 31969321 Free PMC article.
-
In Vivo Neuromechanics: Decoding Causal Motor Neuron Behavior with Resulting Musculoskeletal Function.Sci Rep. 2017 Oct 18;7(1):13465. doi: 10.1038/s41598-017-13766-6. Sci Rep. 2017. PMID: 29044165 Free PMC article.
References
-
- Swinnen SP, Duysens J. Neuro-behavioral determinants of interlimb coordination: a multidisciplinary approach. Kluwer Academic; Boston: 2004. p. xxx.p. 329.
-
- Lebedev MA, Nicolelis MA. Brain-machine interfaces: past, present and future. Trends Neurosci. 2006 Sep;29:536. - PubMed
-
- Nicolelis MA, Lebedev MA. Principles of neural ensemble physiology underlying the operation of brain-machine interfaces. Nat Rev Neurosci. 2009 Jul;10:530. - PubMed
-
- Lebedev MA, Nicolelis MA. Toward a whole-body neuroprosthetic. Prog Brain Res. 2011;194:47. - PubMed
-
- Chestek CA, et al. Neural prosthetic systems: current problems and future directions. Conf Proc IEEE Eng Med Biol Soc. 2009;2009:3369. - PubMed
Publication types
MeSH terms
Grants and funding
LinkOut - more resources
Full Text Sources
Other Literature Sources
Research Materials