The anatomy of choice: active inference and agency
- PMID: 24093015
- PMCID: PMC3782702
- DOI: 10.3389/fnhum.2013.00598
The anatomy of choice: active inference and agency
Abstract
This paper considers agency in the setting of embodied or active inference. In brief, we associate a sense of agency with prior beliefs about action and ask what sorts of beliefs underlie optimal behavior. In particular, we consider prior beliefs that action minimizes the Kullback-Leibler (KL) divergence between desired states and attainable states in the future. This allows one to formulate bounded rationality as approximate Bayesian inference that optimizes a free energy bound on model evidence. We show that constructs like expected utility, exploration bonuses, softmax choice rules and optimism bias emerge as natural consequences of this formulation. Previous accounts of active inference have focused on predictive coding and Bayesian filtering schemes for minimizing free energy. Here, we consider variational Bayes as an alternative scheme that provides formal constraints on the computational anatomy of inference and action-constraints that are remarkably consistent with neuroanatomy. Furthermore, this scheme contextualizes optimal decision theory and economic (utilitarian) formulations as pure inference problems. For example, expected utility theory emerges as a special case of free energy minimization, where the sensitivity or inverse temperature (of softmax functions and quantal response equilibria) has a unique and Bayes-optimal solution-that minimizes free energy. This sensitivity corresponds to the precision of beliefs about behavior, such that attainable goals are afforded a higher precision or confidence. In turn, this means that optimal behavior entails a representation of confidence about outcomes that are under an agent's control.
Keywords: Bayesian; active inference; agency; bounded rationality; embodied cognition; free energy; inference; utility theory.
Figures
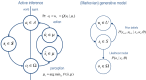
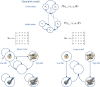
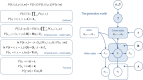
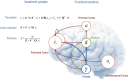
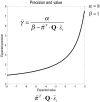
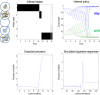
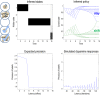
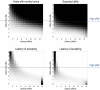
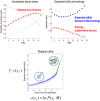
Similar articles
-
The anatomy of choice: dopamine and decision-making.Philos Trans R Soc Lond B Biol Sci. 2014 Nov 5;369(1655):20130481. doi: 10.1098/rstb.2013.0481. Philos Trans R Soc Lond B Biol Sci. 2014. PMID: 25267823 Free PMC article.
-
Active inference and epistemic value.Cogn Neurosci. 2015;6(4):187-214. doi: 10.1080/17588928.2015.1020053. Epub 2015 Mar 13. Cogn Neurosci. 2015. PMID: 25689102
-
Active inference and agency: optimal control without cost functions.Biol Cybern. 2012 Oct;106(8-9):523-41. doi: 10.1007/s00422-012-0512-8. Epub 2012 Aug 3. Biol Cybern. 2012. PMID: 22864468
-
Computational Neuropsychology and Bayesian Inference.Front Hum Neurosci. 2018 Feb 23;12:61. doi: 10.3389/fnhum.2018.00061. eCollection 2018. Front Hum Neurosci. 2018. PMID: 29527157 Free PMC article. Review.
-
Active inference leads to Bayesian neurophysiology.Neurosci Res. 2022 Feb;175:38-45. doi: 10.1016/j.neures.2021.12.003. Epub 2021 Dec 27. Neurosci Res. 2022. PMID: 34968557 Review.
Cited by
-
Computational Psychiatry: towards a mathematically informed understanding of mental illness.J Neurol Neurosurg Psychiatry. 2016 Jan;87(1):53-63. doi: 10.1136/jnnp-2015-310737. Epub 2015 Jul 8. J Neurol Neurosurg Psychiatry. 2016. PMID: 26157034 Free PMC article. Review.
-
Permutation Entropy as a Universal Disorder Criterion: How Disorders at Different Scale Levels Are Manifestations of the Same Underlying Principle.Entropy (Basel). 2021 Dec 20;23(12):1701. doi: 10.3390/e23121701. Entropy (Basel). 2021. PMID: 34946007 Free PMC article.
-
Caching mechanisms for habit formation in Active Inference.Neurocomputing (Amst). 2019 Sep 24;359:298-314. doi: 10.1016/j.neucom.2019.05.083. Neurocomputing (Amst). 2019. PMID: 32055104 Free PMC article.
-
The Computational Anatomy of Visual Neglect.Cereb Cortex. 2018 Feb 1;28(2):777-790. doi: 10.1093/cercor/bhx316. Cereb Cortex. 2018. PMID: 29190328 Free PMC article.
-
Retrospective Inference as a Form of Bounded Rationality, and Its Beneficial Influence on Learning.Front Artif Intell. 2020 Feb 18;3:2. doi: 10.3389/frai.2020.00002. eCollection 2020. Front Artif Intell. 2020. PMID: 33733122 Free PMC article.
References
-
- Beal M. J. (2003). Variational Algorithms for Approximate Bayesian Inference. Ph.D. thesis, University College London.
-
- Braun D. A., Ortega P. A., Theodorou E., Schaal S. (2011). Path integral control and bounded rationality, in IEEE Symposium on Adaptive Dynamic Programming and Reinforcement Learning (ADPRL) (Paris: IEEE; ), 202–209
Grants and funding
LinkOut - more resources
Full Text Sources
Other Literature Sources