Energetic cost of brain functional connectivity
- PMID: 23898179
- PMCID: PMC3746878
- DOI: 10.1073/pnas.1303346110
Energetic cost of brain functional connectivity
Abstract
The brain's functional connectivity is complex, has high energetic cost, and requires efficient use of glucose, the brain's main energy source. It has been proposed that regions with a high degree of functional connectivity are energy efficient and can minimize consumption of glucose. However, the relationship between functional connectivity and energy consumption in the brain is poorly understood. To address this neglect, here we propose a simple model for the energy demands of brain functional connectivity, which we tested with positron emission tomography and MRI in 54 healthy volunteers at rest. Higher glucose metabolism was associated with proportionally larger MRI signal amplitudes, and a higher degree of connectivity was associated with nonlinear increases in metabolism, supporting our hypothesis for the energy efficiency of the connectivity hubs. Basal metabolism (in the absence of connectivity) accounted for 30% of brain glucose utilization, which suggests that the spontaneous brain activity accounts for 70% of the energy consumed by the brain. The energy efficiency of the connectivity hubs was higher for ventral precuneus, cerebellum, and subcortical hubs than for cortical hubs. The higher energy demands of brain communication that hinges upon higher connectivity could render brain hubs more vulnerable to deficits in energy delivery or utilization and help explain their sensitivity to neurodegenerative conditions, such as Alzheimer's disease.
Keywords: PET-FDG; allometric scaling; energy budget; fMRI connectivity; graph theory.
Conflict of interest statement
The authors declare no conflict of interest.
Figures
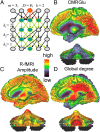
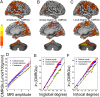
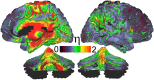
Similar articles
-
The brain's "dark energy" puzzle: How strongly is glucose metabolism linked to resting-state brain activity?J Cereb Blood Flow Metab. 2024 Aug;44(8):1433-1449. doi: 10.1177/0271678X241237974. Epub 2024 Mar 5. J Cereb Blood Flow Metab. 2024. PMID: 38443762
-
Dynamic brain glucose metabolism identifies anti-correlated cortical-cerebellar networks at rest.J Cereb Blood Flow Metab. 2017 Dec;37(12):3659-3670. doi: 10.1177/0271678X17708692. Epub 2017 May 23. J Cereb Blood Flow Metab. 2017. PMID: 28534658 Free PMC article.
-
Default-mode network functional connectivity is closely related to metabolic activity.Hum Brain Mapp. 2015 Jun;36(6):2027-38. doi: 10.1002/hbm.22753. Epub 2015 Feb 3. Hum Brain Mapp. 2015. PMID: 25644693 Free PMC article.
-
Cerebral energy metabolism and the brain's functional network architecture: an integrative review.J Cereb Blood Flow Metab. 2013 Sep;33(9):1347-54. doi: 10.1038/jcbfm.2013.94. Epub 2013 Jun 12. J Cereb Blood Flow Metab. 2013. PMID: 23756687 Free PMC article. Review.
-
Graph theoretical analysis of structural and functional connectivity MRI in normal and pathological brain networks.MAGMA. 2010 Dec;23(5-6):409-21. doi: 10.1007/s10334-010-0205-z. Epub 2010 Mar 27. MAGMA. 2010. PMID: 20349109 Review.
Cited by
-
Effects of chronic and acute stimulants on brain functional connectivity hubs.Brain Res. 2015 Dec 2;1628(Pt A):147-56. doi: 10.1016/j.brainres.2015.02.002. Epub 2015 Feb 24. Brain Res. 2015. PMID: 25721787 Free PMC article.
-
With Great Brain Hub Connectivity Comes Great Vulnerability.CNS Neurosci Ther. 2015 Jul;21(7):541-2. doi: 10.1111/cns.12407. CNS Neurosci Ther. 2015. PMID: 26096045 Free PMC article. No abstract available.
-
Gene transcription profiles associated with inter-modular hubs and connection distance in human functional magnetic resonance imaging networks.Philos Trans R Soc Lond B Biol Sci. 2016 Oct 5;371(1705):20150362. doi: 10.1098/rstb.2015.0362. Philos Trans R Soc Lond B Biol Sci. 2016. PMID: 27574314 Free PMC article.
-
Measures of Brain Connectivity and Cognition by Sex in US Children.JAMA Netw Open. 2023 Feb 1;6(2):e230157. doi: 10.1001/jamanetworkopen.2023.0157. JAMA Netw Open. 2023. PMID: 36809470 Free PMC article.
-
Resting-state functional connectivity alterations in periventricular nodular heterotopia related epilepsy.Sci Rep. 2019 Dec 5;9(1):18473. doi: 10.1038/s41598-019-55002-3. Sci Rep. 2019. PMID: 31804610 Free PMC article.
References
-
- Clark D, Sokoloff L. In: Basic Neurochemistry: Molecular, Cellular, and Medical Aspects. Siegel G, Agranoff B, editors. Philadelphia: Lippincott Williams & Wilkins; 1999. pp. 637–670.
-
- Bullmore E, Sporns O. The economy of brain network organization. Nat Rev Neurosci. 2012;13(5):336–349. - PubMed
-
- Alle H, Roth A, Geiger JR. Energy-efficient action potentials in hippocampal mossy fibers. Science. 2009;325(5946):1405–1408. - PubMed
Publication types
MeSH terms
Substances
Grants and funding
LinkOut - more resources
Full Text Sources
Other Literature Sources