Cognitive control over learning: creating, clustering, and generalizing task-set structure
- PMID: 23356780
- PMCID: PMC3974273
- DOI: 10.1037/a0030852
Cognitive control over learning: creating, clustering, and generalizing task-set structure
Abstract
Learning and executive functions such as task-switching share common neural substrates, notably prefrontal cortex and basal ganglia. Understanding how they interact requires studying how cognitive control facilitates learning but also how learning provides the (potentially hidden) structure, such as abstract rules or task-sets, needed for cognitive control. We investigate this question from 3 complementary angles. First, we develop a new context-task-set (C-TS) model, inspired by nonparametric Bayesian methods, specifying how the learner might infer hidden structure (hierarchical rules) and decide to reuse or create new structure in novel situations. Second, we develop a neurobiologically explicit network model to assess mechanisms of such structured learning in hierarchical frontal cortex and basal ganglia circuits. We systematically explore the link between these modeling levels across task demands. We find that the network provides an approximate implementation of high-level C-TS computations, with specific neural mechanisms modulating distinct C-TS parameters. Third, this synergism yields predictions about the nature of human optimal and suboptimal choices and response times during learning and task-switching. In particular, the models suggest that participants spontaneously build task-set structure into a learning problem when not cued to do so, which predicts positive and negative transfer in subsequent generalization tests. We provide experimental evidence for these predictions and show that C-TS provides a good quantitative fit to human sequences of choices. These findings implicate a strong tendency to interactively engage cognitive control and learning, resulting in structured abstract representations that afford generalization opportunities and, thus, potentially long-term rather than short-term optimality.
Figures
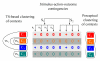
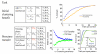
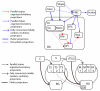
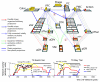
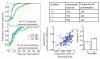
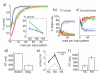
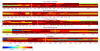
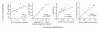
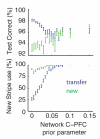
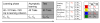
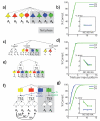
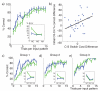
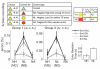
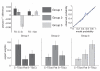
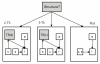
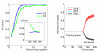
Similar articles
-
Dissociable Neural Systems Support the Learning and Transfer of Hierarchical Control Structure.J Neurosci. 2020 Aug 19;40(34):6624-6637. doi: 10.1523/JNEUROSCI.0847-20.2020. Epub 2020 Jul 20. J Neurosci. 2020. PMID: 32690614 Free PMC article.
-
Hierarchically Organized Medial Frontal Cortex-Basal Ganglia Loops Selectively Control Task- and Response-Selection.J Neurosci. 2017 Aug 16;37(33):7893-7905. doi: 10.1523/JNEUROSCI.3289-16.2017. Epub 2017 Jul 17. J Neurosci. 2017. PMID: 28716966 Free PMC article.
-
Role of Prefrontal Cortex in Learning and Generalizing Hierarchical Rules in 8-Month-Old Infants.J Neurosci. 2016 Oct 5;36(40):10314-10322. doi: 10.1523/JNEUROSCI.1351-16.2016. J Neurosci. 2016. PMID: 27707968 Free PMC article.
-
The frontal cortex-basal ganglia system in primates.Crit Rev Neurobiol. 1996;10(3-4):317-56. doi: 10.1615/critrevneurobiol.v10.i3-4.30. Crit Rev Neurobiol. 1996. PMID: 8978985 Review.
-
Structured sequence processing and combinatorial binding: neurobiologically and computationally informed hypotheses.Philos Trans R Soc Lond B Biol Sci. 2020 Feb 3;375(1791):20190304. doi: 10.1098/rstb.2019.0304. Epub 2019 Dec 16. Philos Trans R Soc Lond B Biol Sci. 2020. PMID: 31840585 Free PMC article. Review.
Cited by
-
Wave-like dopamine dynamics as a mechanism for spatiotemporal credit assignment.Cell. 2021 May 13;184(10):2733-2749.e16. doi: 10.1016/j.cell.2021.03.046. Epub 2021 Apr 15. Cell. 2021. PMID: 33861952 Free PMC article.
-
Hierarchical clustering optimizes the tradeoff between compositionality and expressivity of task structures for flexible reinforcement learning.Artif Intell. 2022 Nov;312:103770. doi: 10.1016/j.artint.2022.103770. Epub 2022 Aug 5. Artif Intell. 2022. PMID: 36711165 Free PMC article.
-
Visuospatial information foraging describes search behavior in learning latent environmental features.Sci Rep. 2023 Jan 20;13(1):1126. doi: 10.1038/s41598-023-27662-9. Sci Rep. 2023. PMID: 36670132 Free PMC article.
-
Chunking as a rational strategy for lossy data compression in visual working memory.Psychol Rev. 2018 Jul;125(4):486-511. doi: 10.1037/rev0000101. Psychol Rev. 2018. PMID: 29952621 Free PMC article.
-
Hierarchical consciousness: the Nested Observer Windows model.Neurosci Conscious. 2024 Mar 18;2024(1):niae010. doi: 10.1093/nc/niae010. eCollection 2024. Neurosci Conscious. 2024. PMID: 38504828 Free PMC article.
References
-
- Aisa B, Mingus B, O’Reilly R. The emergent neural modeling system. Neural networks: the official journal of the International Neural Network Society. 2008;21(8):1146–52. - PubMed
-
- Akaike H. A new look at the statistical model identification. IEEE Transactions on Automatic Control. 1974;19(6):716–723.
-
- Aldous D. Exchangeability and related topics. École dÉté de Probabilités de SaintFlour XIII 1983. 1985;1117(2):1–198.
-
- Alexander G, DeLong M. Parallel organization of functionally segregated circuits linking basal ganglia and cortex. Annual review of neuroscience. 1986 - PubMed
Publication types
MeSH terms
Grants and funding
LinkOut - more resources
Full Text Sources
Other Literature Sources
Research Materials