Bayesian non-parametrics and the probabilistic approach to modelling
- PMID: 23277609
- PMCID: PMC3538441
- DOI: 10.1098/rsta.2011.0553
Bayesian non-parametrics and the probabilistic approach to modelling
Abstract
Modelling is fundamental to many fields of science and engineering. A model can be thought of as a representation of possible data one could predict from a system. The probabilistic approach to modelling uses probability theory to express all aspects of uncertainty in the model. The probabilistic approach is synonymous with Bayesian modelling, which simply uses the rules of probability theory in order to make predictions, compare alternative models, and learn model parameters and structure from data. This simple and elegant framework is most powerful when coupled with flexible probabilistic models. Flexibility is achieved through the use of Bayesian non-parametrics. This article provides an overview of probabilistic modelling and an accessible survey of some of the main tools in Bayesian non-parametrics. The survey covers the use of Bayesian non-parametrics for modelling unknown functions, density estimation, clustering, time-series modelling, and representing sparsity, hierarchies, and covariance structure. More specifically, it gives brief non-technical overviews of Gaussian processes, Dirichlet processes, infinite hidden Markov models, Indian buffet processes, Kingman's coalescent, Dirichlet diffusion trees and Wishart processes.
Figures
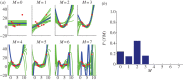
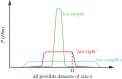
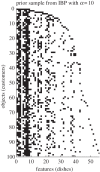
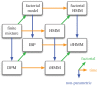
Similar articles
-
Probabilistic machine learning and artificial intelligence.Nature. 2015 May 28;521(7553):452-9. doi: 10.1038/nature14541. Nature. 2015. PMID: 26017444 Review.
-
Generalized species sampling priors with latent Beta reinforcements.J Am Stat Assoc. 2014 Dec 1;109(508):1466-1480. doi: 10.1080/01621459.2014.950735. J Am Stat Assoc. 2014. PMID: 25870462 Free PMC article.
-
Understanding Past Population Dynamics: Bayesian Coalescent-Based Modeling with Covariates.Syst Biol. 2016 Nov;65(6):1041-1056. doi: 10.1093/sysbio/syw050. Epub 2016 Jul 1. Syst Biol. 2016. PMID: 27368344 Free PMC article.
-
Describing disease processes using a probabilistic logic of qualitative time.Artif Intell Med. 2013 Nov;59(3):143-55. doi: 10.1016/j.artmed.2013.09.003. Epub 2013 Oct 7. Artif Intell Med. 2013. PMID: 24183893
-
Bayesian statistical learning for big data biology.Biophys Rev. 2019 Feb;11(1):95-102. doi: 10.1007/s12551-019-00499-1. Epub 2019 Feb 7. Biophys Rev. 2019. PMID: 30729409 Free PMC article. Review.
Cited by
-
Probabilistic machine learning and artificial intelligence.Nature. 2015 May 28;521(7553):452-9. doi: 10.1038/nature14541. Nature. 2015. PMID: 26017444 Review.
-
GEO-CEOS stage 4 validation of the Satellite Image Automatic Mapper lightweight computer program for ESA Earth observation level 2 product generation - Part 1: Theory.Cogent Geosci. 2018 Jun 10;4(1):1-46. doi: 10.1080/23312041.2018.1467357. eCollection 2018. Cogent Geosci. 2018. PMID: 30035156 Free PMC article.
-
Cognitive computational neuroscience.Nat Neurosci. 2018 Sep;21(9):1148-1160. doi: 10.1038/s41593-018-0210-5. Epub 2018 Aug 20. Nat Neurosci. 2018. PMID: 30127428 Free PMC article. Review.
-
Inference for the physical sciences.Philos Trans A Math Phys Eng Sci. 2012 Dec 31;371(1984):20120493. doi: 10.1098/rsta.2012.0493. Print 2013 Feb 13. Philos Trans A Math Phys Eng Sci. 2012. PMID: 23277613 Free PMC article.
-
Predicting gene-level sensitivity to JAK-STAT signaling perturbation using a mechanistic-to-machine learning framework.Cell Syst. 2024 Jan 17;15(1):37-48.e4. doi: 10.1016/j.cels.2023.12.006. Epub 2024 Jan 9. Cell Syst. 2024. PMID: 38198893
References
-
- Wolpert DM, Ghahramani Z, Jordan MI. 1995. An internal model for sensorimotor integration. Science 269, 1880–188210.1126/science.7569931 (doi:10.1126/science.7569931) - DOI - DOI - PubMed
-
- Knill D, Richards W. 1996. Perception as Bayesian inference. Cambridge, UK: Cambridge University Press.
-
- Griffiths TL, Tenenbaum JB. 2006. Optimal predictions in everyday cognition. Psychol. Sci. 17, 767–77310.1111/j.1467-9280.2006.01780.x (doi:10.1111/j.1467-9280.2006.01780.x) - DOI - DOI - PubMed
-
- Doob JL. 1949. Application of the theory of martingales. Coll. Int. Centre Nat. Res. Sci. 13, 23–27
-
- Le Cam L. 1986. Asymptotic methods in statistical decision theory. Berlin, Germany: Springer.
LinkOut - more resources
Full Text Sources
Other Literature Sources