Comparison of geostatistical interpolation and remote sensing techniques for estimating long-term exposure to ambient PM2.5 concentrations across the continental United States
- PMID: 23033456
- PMCID: PMC3546366
- DOI: 10.1289/ehp.1205006
Comparison of geostatistical interpolation and remote sensing techniques for estimating long-term exposure to ambient PM2.5 concentrations across the continental United States
Abstract
Background: A better understanding of the adverse health effects of chronic exposure to fine particulate matter (PM2.5) requires accurate estimates of PM2.5 variation at fine spatial scales. Remote sensing has emerged as an important means of estimating PM2.5 exposures, but relatively few studies have compared remote-sensing estimates to those derived from monitor-based data.
Objective: We evaluated and compared the predictive capabilities of remote sensing and geostatistical interpolation.
Methods: We developed a space-time geostatistical kriging model to predict PM2.5 over the continental United States and compared resulting predictions to estimates derived from satellite retrievals.
Results: The kriging estimate was more accurate for locations that were about 100 km from a monitoring station, whereas the remote sensing estimate was more accurate for locations that were > 100 km from a monitoring station. Based on this finding, we developed a hybrid map that combines the kriging and satellite-based PM2.5 estimates.
Conclusions: We found that for most of the populated areas of the continental United States, geostatistical interpolation produced more accurate estimates than remote sensing. The differences between the estimates resulting from the two methods, however, were relatively small. In areas with extensive monitoring networks, the interpolation may provide more accurate estimates, but in the many areas of the world without such monitoring, remote sensing can provide useful exposure estimates that perform nearly as well.
Conflict of interest statement
The authors declare they have no actual or potential competing financial interests.
Figures
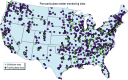
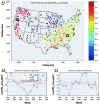
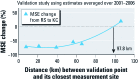
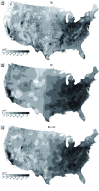
Similar articles
-
Assessment and statistical modeling of the relationship between remotely sensed aerosol optical depth and PM2.5 in the eastern United States.Res Rep Health Eff Inst. 2012 May;(167):5-83; discussion 85-91. Res Rep Health Eff Inst. 2012. PMID: 22838153
-
Evaluation of predictive capabilities of ordinary geostatistical interpolation, hybrid interpolation, and machine learning methods for estimating PM2.5 constituents over space.Environ Res. 2019 Aug;175:421-433. doi: 10.1016/j.envres.2019.05.025. Epub 2019 May 28. Environ Res. 2019. PMID: 31154232
-
A hybrid approach to estimating national scale spatiotemporal variability of PM2.5 in the contiguous United States.Environ Sci Technol. 2013 Jul 2;47(13):7233-41. doi: 10.1021/es400039u. Epub 2013 Jun 11. Environ Sci Technol. 2013. PMID: 23701364 Free PMC article.
-
Satellite remote sensing in epidemiological studies.Curr Opin Pediatr. 2016 Apr;28(2):228-34. doi: 10.1097/MOP.0000000000000326. Curr Opin Pediatr. 2016. PMID: 26859287 Free PMC article. Review.
-
Progress in the Remote Sensing Monitoring of the Ecological Environment in Mining Areas.Int J Environ Res Public Health. 2020 Mar 12;17(6):1846. doi: 10.3390/ijerph17061846. Int J Environ Res Public Health. 2020. PMID: 32178376 Free PMC article. Review.
Cited by
-
Targeting disparate spaces: new technology and old tools.Front Public Health. 2024 Apr 23;12:1366179. doi: 10.3389/fpubh.2024.1366179. eCollection 2024. Front Public Health. 2024. PMID: 38716239 Free PMC article.
-
Ambient fine particulate matter and daily mortality: a comparative analysis of observed and estimated exposure in 347 cities.Int J Epidemiol. 2024 Apr 11;53(3):dyae066. doi: 10.1093/ije/dyae066. Int J Epidemiol. 2024. PMID: 38725299 Free PMC article.
-
Spatial modeling of PM2.5 concentrations with a multifactoral radial basis function neural network.Environ Sci Pollut Res Int. 2015 Jul;22(14):10395-404. doi: 10.1007/s11356-015-4380-3. Epub 2015 Mar 28. Environ Sci Pollut Res Int. 2015. PMID: 25813644
-
Spatial Patterns of Satellite-Retrieved PM2.5 and Long-Term Exposure Assessment of China from 1998 to 2016.Int J Environ Res Public Health. 2018 Dec 8;15(12):2785. doi: 10.3390/ijerph15122785. Int J Environ Res Public Health. 2018. PMID: 30544813 Free PMC article.
-
Spatial-Temporal Evolution of PM2.5 Concentration and its Socioeconomic Influence Factors in Chinese Cities in 2014⁻2017.Int J Environ Res Public Health. 2019 Mar 19;16(6):985. doi: 10.3390/ijerph16060985. Int J Environ Res Public Health. 2019. PMID: 30893835 Free PMC article.
References
-
- BMElib. The Bayesian Maximum Entropy Software for Space/Time Geostatistics, and Temporal GIS Data Integration. 2012. Available: http://www.unc.edu/depts/case/BMELIB [accessed 31 October 2012]
-
- Christakos G. Mineola, NY: Dover Publications; 1992. Random Field Models in Earth Sciences.
-
- Christakos G. Modern Spatiotemporal Geostatistics. New York:Oxford University Press 2000
Publication types
MeSH terms
Substances
Grants and funding
LinkOut - more resources
Full Text Sources