A computational neurodegenerative disease progression score: method and results with the Alzheimer's disease Neuroimaging Initiative cohort
- PMID: 22885136
- PMCID: PMC3472161
- DOI: 10.1016/j.neuroimage.2012.07.059
A computational neurodegenerative disease progression score: method and results with the Alzheimer's disease Neuroimaging Initiative cohort
Abstract
While neurodegenerative diseases are characterized by steady degeneration over relatively long timelines, it is widely believed that the early stages are the most promising for therapeutic intervention, before irreversible neuronal loss occurs. Developing a therapeutic response requires a precise measure of disease progression. However, since the early stages are for the most part asymptomatic, obtaining accurate measures of disease progression is difficult. Longitudinal databases of hundreds of subjects observed during several years with tens of validated biomarkers are becoming available, allowing the use of computational methods. We propose a widely applicable statistical methodology for creating a disease progression score (DPS), using multiple biomarkers, for subjects with a neurodegenerative disease. The proposed methodology was evaluated for Alzheimer's disease (AD) using the publicly available AD Neuroimaging Initiative (ADNI) database, yielding an Alzheimer's DPS or ADPS score for each subject and each time-point in the database. In addition, a common description of biomarker changes was produced allowing for an ordering of the biomarkers. The Rey Auditory Verbal Learning Test delayed recall was found to be the earliest biomarker to become abnormal. The group of biomarkers comprising the volume of the hippocampus and the protein concentration amyloid beta and Tau were next in the timeline, and these were followed by three cognitive biomarkers. The proposed methodology thus has potential to stage individuals according to their state of disease progression relative to a population and to deduce common behaviors of biomarkers in the disease itself.
Copyright © 2012 Elsevier Inc. All rights reserved.
Figures
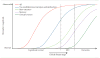
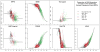
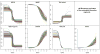
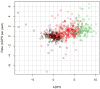
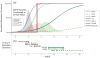
Similar articles
-
A computational method for computing an Alzheimer's disease progression score; experiments and validation with the ADNI data set.Neurobiol Aging. 2015 Jan;36 Suppl 1:S178-84. doi: 10.1016/j.neurobiolaging.2014.03.043. Epub 2014 Oct 17. Neurobiol Aging. 2015. PMID: 25444605 Free PMC article.
-
Disease progression timeline estimation for Alzheimer's disease using discriminative event based modeling.Neuroimage. 2019 Feb 1;186:518-532. doi: 10.1016/j.neuroimage.2018.11.024. Epub 2018 Nov 22. Neuroimage. 2019. PMID: 30471388
-
Analyzing the effect of APOE on Alzheimer's disease progression using an event-based model for stratified populations.Neuroimage. 2021 Feb 15;227:117646. doi: 10.1016/j.neuroimage.2020.117646. Epub 2020 Dec 16. Neuroimage. 2021. PMID: 33338617
-
Core candidate neurochemical and imaging biomarkers of Alzheimer's disease.Alzheimers Dement. 2008 Jan;4(1):38-48. doi: 10.1016/j.jalz.2007.08.006. Epub 2007 Dec 21. Alzheimers Dement. 2008. PMID: 18631949 Review.
-
Update on the biomarker core of the Alzheimer's Disease Neuroimaging Initiative subjects.Alzheimers Dement. 2010 May;6(3):230-8. doi: 10.1016/j.jalz.2010.03.008. Alzheimers Dement. 2010. PMID: 20451871 Free PMC article. Review.
Cited by
-
Simulating the outcome of amyloid treatments in Alzheimer's disease from imaging and clinical data.Brain Commun. 2021 Apr 28;3(2):fcab091. doi: 10.1093/braincomms/fcab091. eCollection 2021. Brain Commun. 2021. PMID: 34085040 Free PMC article.
-
MAPPING ALZHEIMER'S DISEASE PSEUDO-PROGRESSION WITH MULTIMODAL BIOMARKER TRAJECTORY EMBEDDINGS.Proc IEEE Int Symp Biomed Imaging. 2024 May;2024:10.1109/isbi56570.2024.10635249. doi: 10.1109/isbi56570.2024.10635249. Epub 2024 Aug 22. Proc IEEE Int Symp Biomed Imaging. 2024. PMID: 39371469
-
Temporal Trajectory and Progression Score Estimation from Voxelwise Longitudinal Imaging Measures: Application to Amyloid Imaging.Inf Process Med Imaging. 2015;24:424-36. doi: 10.1007/978-3-319-19992-4_33. Inf Process Med Imaging. 2015. PMID: 26221692 Free PMC article.
-
Verbal memory and brain aging: an exploratory analysis of the role of error responses in the Framingham Study.Am J Alzheimers Dis Other Demen. 2015 Sep;30(6):622-8. doi: 10.1177/1533317515577184. Epub 2015 Mar 18. Am J Alzheimers Dis Other Demen. 2015. PMID: 25788434 Free PMC article.
-
Predictors for the development of motoric cognitive risk syndrome in older adults.BMC Geriatr. 2024 Jul 3;24(1):575. doi: 10.1186/s12877-024-05179-8. BMC Geriatr. 2024. PMID: 38961342 Free PMC article.
References
-
- Ashford J, Shan M, Butler S, Rajasekar A, Schmitt F. Temporal quantification of Alzheimer’s disease severity: ‘Time Index’ model. Dementia. 1995;6(5):269–280. - PubMed
-
- Bennett DA, Schneider JA, Arvanitakis Z, Kelly JF, Aggarwal NT, Shah RC, Wilson RS. Neuropathology of older persons without cognitive impairment from two community-based studies. Neurology. 2006;66(12):1837–1844. - PubMed
-
- Berg L, Miller JP, Storandt M, Duchek J, Morris JC, Rubin EH, Burke WJ, Coben LA. Mild senile dementia of the alzheimer type: 2. Longitudinal assessment. Annals of Neurology. 1988;23(5):477–484. - PubMed
-
- Brooks DJ, Pavese N. Imaging biomarkers in parkinson’s disease. Progress in Neurobiology. 2011 Aug; URL http://dx.doi.org/10.1016/j.pneurobio.2011.08.009. - DOI - PubMed
-
- Brooks J, 3rd, Kraemer H, Tanke E, Yesavage J. The methodology of studying decline in Alzheimer’s disease. Journal of the American Geriatrics Society. 1993;41(6):623–628. - PubMed
Publication types
MeSH terms
Substances
Grants and funding
LinkOut - more resources
Full Text Sources
Medical