Waking and dreaming consciousness: neurobiological and functional considerations
- PMID: 22609044
- PMCID: PMC3389346
- DOI: 10.1016/j.pneurobio.2012.05.003
Waking and dreaming consciousness: neurobiological and functional considerations
Abstract
This paper presents a theoretical review of rapid eye movement sleep with a special focus on pontine-geniculate-occipital waves and what they might tell us about the functional anatomy of sleep and consciousness. In particular, we review established ideas about the nature and purpose of sleep in terms of protoconsciousness and free energy minimization. By combining these theoretical perspectives, we discover answers to some fundamental questions about sleep: for example, why is homeothermy suspended during sleep? Why is sleep necessary? Why are we not surprised by our dreams? What is the role of synaptic regression in sleep? The imperatives for sleep that emerge also allow us to speculate about the functional role of PGO waves and make some empirical predictions that can, in principle, be tested using recent advances in the modeling of electrophysiological data.
Copyright © 2012 Elsevier Ltd. All rights reserved.
Figures
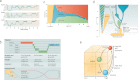
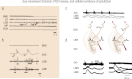
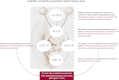
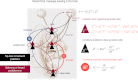
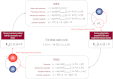
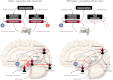
Similar articles
-
REM sleep burst neurons, PGO waves, and eye movement information.J Neurophysiol. 1983 Oct;50(4):784-97. doi: 10.1152/jn.1983.50.4.784. J Neurophysiol. 1983. PMID: 6631463
-
Localization of pontine PGO wave generation sites and their anatomical projections in the rat.Synapse. 1998 Dec;30(4):409-23. doi: 10.1002/(SICI)1098-2396(199812)30:4<409::AID-SYN8>3.0.CO;2-#. Synapse. 1998. PMID: 9826233
-
Serotonin neurons and sleep. I. Long term recordings of dorsal raphe discharge frequency and PGO waves.Arch Ital Biol. 1987 Oct;125(4):317-43. Arch Ital Biol. 1987. PMID: 3439827
-
Sleep and dreaming: induction and mediation of REM sleep by cholinergic mechanisms.Curr Opin Neurobiol. 1992 Dec;2(6):759-63. doi: 10.1016/0959-4388(92)90130-d. Curr Opin Neurobiol. 1992. PMID: 1477541 Review.
-
REM sleep and dreaming: towards a theory of protoconsciousness.Nat Rev Neurosci. 2009 Nov;10(11):803-13. doi: 10.1038/nrn2716. Epub 2009 Oct 1. Nat Rev Neurosci. 2009. PMID: 19794431 Review.
Cited by
-
Structure learning enhances concept formation in synthetic Active Inference agents.PLoS One. 2022 Nov 14;17(11):e0277199. doi: 10.1371/journal.pone.0277199. eCollection 2022. PLoS One. 2022. PMID: 36374909 Free PMC article.
-
Free Energy and Virtual Reality in Neuroscience and Psychoanalysis: A Complexity Theory of Dreaming and Mental Disorder.Front Psychol. 2016 Jul 15;7:922. doi: 10.3389/fpsyg.2016.00922. eCollection 2016. Front Psychol. 2016. PMID: 27471478 Free PMC article.
-
Ganzfeld stimulation or sleep enhance long term motor memory consolidation compared to normal viewing in saccadic adaptation paradigm.PLoS One. 2015 Apr 13;10(4):e0123831. doi: 10.1371/journal.pone.0123831. eCollection 2015. PLoS One. 2015. PMID: 25867186 Free PMC article. Clinical Trial.
-
Feasibility of a Personal Neuromorphic Emulation.Entropy (Basel). 2024 Sep 5;26(9):759. doi: 10.3390/e26090759. Entropy (Basel). 2024. PMID: 39330092 Free PMC article.
-
Model Reduction Through Progressive Latent Space Pruning in Deep Active Inference.Front Neurorobot. 2022 Mar 11;16:795846. doi: 10.3389/fnbot.2022.795846. eCollection 2022. Front Neurorobot. 2022. PMID: 35360827 Free PMC article.
References
-
- Ashby W.R. Principles of the self-organizing dynamic system. Journal of General Psychology. 1947;37:125–128. - PubMed
-
- Aston-Jones G., Chiang C., Alexinsky T. Discharge of noradrenergic locus coeruleus neurons in behaving rats and monkeys suggests a role in vigilance. Progress in Brain Research. 1991;88:501–520. - PubMed
-
- Barlow H.B. Inductive inference, coding, perception, and language. Perception. 1974;3:123–134. - PubMed
-
- Beal, M.J., 2003. Variational algorithms for approximate bayesian inference. PhD. Thesis. University College London.
-
- Bernard C. Charles C Thomas; Springfield (IL): 1974. Lectures on the Phenomena Common to Animals and Plants.
Publication types
MeSH terms
Grants and funding
LinkOut - more resources
Full Text Sources