Reach and grasp by people with tetraplegia using a neurally controlled robotic arm
- PMID: 22596161
- PMCID: PMC3640850
- DOI: 10.1038/nature11076
Reach and grasp by people with tetraplegia using a neurally controlled robotic arm
Abstract
Paralysis following spinal cord injury, brainstem stroke, amyotrophic lateral sclerosis and other disorders can disconnect the brain from the body, eliminating the ability to perform volitional movements. A neural interface system could restore mobility and independence for people with paralysis by translating neuronal activity directly into control signals for assistive devices. We have previously shown that people with long-standing tetraplegia can use a neural interface system to move and click a computer cursor and to control physical devices. Able-bodied monkeys have used a neural interface system to control a robotic arm, but it is unknown whether people with profound upper extremity paralysis or limb loss could use cortical neuronal ensemble signals to direct useful arm actions. Here we demonstrate the ability of two people with long-standing tetraplegia to use neural interface system-based control of a robotic arm to perform three-dimensional reach and grasp movements. Participants controlled the arm and hand over a broad space without explicit training, using signals decoded from a small, local population of motor cortex (MI) neurons recorded from a 96-channel microelectrode array. One of the study participants, implanted with the sensor 5 years earlier, also used a robotic arm to drink coffee from a bottle. Although robotic reach and grasp actions were not as fast or accurate as those of an able-bodied person, our results demonstrate the feasibility for people with tetraplegia, years after injury to the central nervous system, to recreate useful multidimensional control of complex devices directly from a small sample of neural signals.
Figures
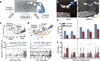
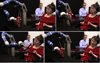
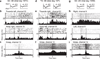
Comment in
-
Neuroscience: Brain-controlled robot grabs attention.Nature. 2012 May 16;485(7398):317-8. doi: 10.1038/485317a. Nature. 2012. PMID: 22596151 No abstract available.
-
Neural repair and rehabilitation: Neurally controlled robotic arm enables tetraplegic patient to drink coffee of her own volition.Nat Rev Neurol. 2012 Jun 12;8(7):353. doi: 10.1038/nrneurol.2012.101. Nat Rev Neurol. 2012. PMID: 22688782 No abstract available.
Similar articles
-
Restoration of reaching and grasping movements through brain-controlled muscle stimulation in a person with tetraplegia: a proof-of-concept demonstration.Lancet. 2017 May 6;389(10081):1821-1830. doi: 10.1016/S0140-6736(17)30601-3. Epub 2017 Mar 28. Lancet. 2017. PMID: 28363483 Free PMC article. Clinical Trial.
-
Restoring cortical control of functional movement in a human with quadriplegia.Nature. 2016 May 12;533(7602):247-50. doi: 10.1038/nature17435. Epub 2016 Apr 13. Nature. 2016. PMID: 27074513
-
Neural control of cursor trajectory and click by a human with tetraplegia 1000 days after implant of an intracortical microelectrode array.J Neural Eng. 2011 Apr;8(2):025027. doi: 10.1088/1741-2560/8/2/025027. Epub 2011 Mar 24. J Neural Eng. 2011. PMID: 21436513 Free PMC article.
-
Assistive technology and robotic control using motor cortex ensemble-based neural interface systems in humans with tetraplegia.J Physiol. 2007 Mar 15;579(Pt 3):603-11. doi: 10.1113/jphysiol.2006.127209. Epub 2007 Feb 1. J Physiol. 2007. PMID: 17272345 Free PMC article. Review.
-
A review of methods for achieving upper limb movement following spinal cord injury through hybrid muscle stimulation and robotic assistance.Exp Neurol. 2020 Jun;328:113274. doi: 10.1016/j.expneurol.2020.113274. Epub 2020 Mar 5. Exp Neurol. 2020. PMID: 32145251 Review.
Cited by
-
Motor directional tuning across brain areas: directional resonance and the role of inhibition for directional accuracy.Front Neural Circuits. 2013 May 15;7:92. doi: 10.3389/fncir.2013.00092. eCollection 2013. Front Neural Circuits. 2013. PMID: 23720612 Free PMC article. Review.
-
Decoding upper limb residual muscle activity in severe chronic stroke.Ann Clin Transl Neurol. 2015 Jan;2(1):1-11. doi: 10.1002/acn3.122. Epub 2014 Dec 9. Ann Clin Transl Neurol. 2015. PMID: 25642429 Free PMC article.
-
Neural repair and rehabilitation: Achieving complex control of a neuroprosthetic arm.Nat Rev Neurol. 2013 Feb;9(2):62. doi: 10.1038/nrneurol.2013.1. Epub 2013 Jan 15. Nat Rev Neurol. 2013. PMID: 23318294 No abstract available.
-
Hybrid Neuroprosthesis for the Upper Limb: Combining Brain-Controlled Neuromuscular Stimulation with a Multi-Joint Arm Exoskeleton.Front Neurosci. 2016 Aug 9;10:367. doi: 10.3389/fnins.2016.00367. eCollection 2016. Front Neurosci. 2016. PMID: 27555805 Free PMC article.
-
A Review of Control Strategies in Closed-Loop Neuroprosthetic Systems.Front Neurosci. 2016 Jul 12;10:312. doi: 10.3389/fnins.2016.00312. eCollection 2016. Front Neurosci. 2016. PMID: 27462202 Free PMC article. Review.
References
-
- Donoghue JP. Bridging the brain to the world: a perspective on neural interface systems. Neuron. 2008;60:511–521. - PubMed
-
- Schwartz AB, Cui XT, Weber DJ, Moran DW. Brain-controlled interfaces: Movement restoration with neural prosthetics. Neuron. 2006;52:205–220. - PubMed
-
- Nicolelis MAL, Lebedev MA. Principles of neural ensemble physiology underlying the operation of brain-machine interfaces. Nature Reviews Neuroscience. 2009;10:530–540. - PubMed
-
- Green AM, Kalaska JF. Learning to move machines with the mind. Trends Neurosci. 2011;34:61–75. - PubMed
Publication types
MeSH terms
Grants and funding
- HHSN275201100018C/HD/NICHD NIH HHS/United States
- N01HD53403/HD/NICHD NIH HHS/United States
- R01DC009899/DC/NIDCD NIH HHS/United States
- R01 NS025074/NS/NINDS NIH HHS/United States
- R01 DC009899-02/DC/NIDCD NIH HHS/United States
- N01 HD053403/HD/NICHD NIH HHS/United States
- R56 NS025074-23/NS/NINDS NIH HHS/United States
- RC1 HD063931/HD/NICHD NIH HHS/United States
- N01HD10018/HD/NICHD NIH HHS/United States
- R37 NS025074/NS/NINDS NIH HHS/United States
- R01EB007401/EB/NIBIB NIH HHS/United States
- R01 DC009899/DC/NIDCD NIH HHS/United States
- RC1 HD063931-02/HD/NICHD NIH HHS/United States
- R01 EB007401/EB/NIBIB NIH HHS/United States
- NS25074/NS/NINDS NIH HHS/United States
- RC1HD063931/HD/NICHD NIH HHS/United States
- R01 EB007401-05/EB/NIBIB NIH HHS/United States
LinkOut - more resources
Full Text Sources
Other Literature Sources