Selectionist and evolutionary approaches to brain function: a critical appraisal
- PMID: 22557963
- PMCID: PMC3337445
- DOI: 10.3389/fncom.2012.00024
Selectionist and evolutionary approaches to brain function: a critical appraisal
Abstract
We consider approaches to brain dynamics and function that have been claimed to be Darwinian. These include Edelman's theory of neuronal group selection, Changeux's theory of synaptic selection and selective stabilization of pre-representations, Seung's Darwinian synapse, Loewenstein's synaptic melioration, Adam's selfish synapse, and Calvin's replicating activity patterns. Except for the last two, the proposed mechanisms are selectionist but not truly Darwinian, because no replicators with information transfer to copies and hereditary variation can be identified in them. All of them fit, however, a generalized selectionist framework conforming to the picture of Price's covariance formulation, which deliberately was not specific even to selection in biology, and therefore does not imply an algorithmic picture of biological evolution. Bayesian models and reinforcement learning are formally in agreement with selection dynamics. A classification of search algorithms is shown to include Darwinian replicators (evolutionary units with multiplication, heredity, and variability) as the most powerful mechanism for search in a sparsely occupied search space. Examples are given of cases where parallel competitive search with information transfer among the units is more efficient than search without information transfer between units. Finally, we review our recent attempts to construct and analyze simple models of true Darwinian evolutionary units in the brain in terms of connectivity and activity copying of neuronal groups. Although none of the proposed neuronal replicators include miraculous mechanisms, their identification remains a challenge but also a great promise.
Keywords: Darwinian neurodynamics; Izhikevich spiking networks; causal inference; hill-climbers; neural Darwinism; neuronal group selection; neuronal replicator hypothesis; price equation.
Figures
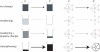
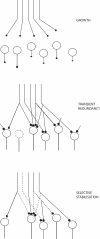
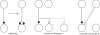
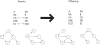
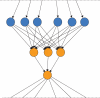
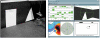
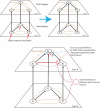
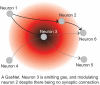
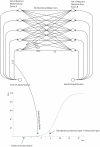
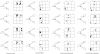
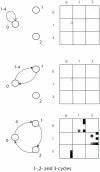
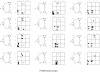
Similar articles
-
Breeding novel solutions in the brain: a model of Darwinian neurodynamics.F1000Res. 2016 Sep 28;5:2416. doi: 10.12688/f1000research.9630.2. eCollection 2016. F1000Res. 2016. PMID: 27990266 Free PMC article.
-
Behavioral and neural Darwinism: selectionist function and mechanism in adaptive behavior dynamics.Behav Processes. 2010 May;84(1):358-65. doi: 10.1016/j.beproc.2009.11.011. Epub 2009 Nov 24. Behav Processes. 2010. PMID: 19941941
-
The neuronal replicator hypothesis.Neural Comput. 2010 Nov;22(11):2809-57. doi: 10.1162/NECO_a_00031. Neural Comput. 2010. PMID: 20804380
-
Price's equation made clear.Philos Trans R Soc Lond B Biol Sci. 2020 Apr 27;375(1797):20190361. doi: 10.1098/rstb.2019.0361. Epub 2020 Mar 9. Philos Trans R Soc Lond B Biol Sci. 2020. PMID: 32146889 Free PMC article. Review.
-
Is pre-Darwinian evolution plausible?Biol Direct. 2018 Sep 21;13(1):18. doi: 10.1186/s13062-018-0216-7. Biol Direct. 2018. PMID: 30241560 Free PMC article. Review.
Cited by
-
Insight into the ten-penny problem: guiding search by constraints and maximization.Psychol Res. 2017 Sep;81(5):925-938. doi: 10.1007/s00426-016-0800-3. Epub 2016 Sep 3. Psychol Res. 2017. PMID: 27592343 Free PMC article.
-
Search and insight processes in card sorting games.Front Psychol. 2023 May 5;14:1118976. doi: 10.3389/fpsyg.2023.1118976. eCollection 2023. Front Psychol. 2023. PMID: 37213381 Free PMC article.
-
Expansion and Renormalization of Human Brain Structure During Skill Acquisition.Trends Cogn Sci. 2017 Dec;21(12):930-939. doi: 10.1016/j.tics.2017.09.008. Trends Cogn Sci. 2017. PMID: 29149999 Free PMC article. Review.
-
On Having No Head: Cognition throughout Biological Systems.Front Psychol. 2016 Jun 21;7:902. doi: 10.3389/fpsyg.2016.00902. eCollection 2016. Front Psychol. 2016. PMID: 27445884 Free PMC article. Review.
-
Cognitive Architecture with Evolutionary Dynamics Solves Insight Problem.Front Psychol. 2017 Mar 29;8:427. doi: 10.3389/fpsyg.2017.00427. eCollection 2017. Front Psychol. 2017. PMID: 28405191 Free PMC article.
References
-
- Arnold D., Beyer H. (2003). A comparison of evolution strategies with other direct search methods in the presence of noise. Comput. Optim. Appl. 24, 135–15910.1023/A:1021810301763 - DOI
-
- Aunger R. (2002). The Electric Meme: A New Theory of How We Think. New York: The Free Press
-
- Barnett L. (2001). “Netcrawling-optimal evolutionary search with neutral networks,” in Congress on Evolutionary Computation CEC’01 (Seoul: IEEE Press; ), 30–37
LinkOut - more resources
Full Text Sources
Other Literature Sources