Within-subject template estimation for unbiased longitudinal image analysis
- PMID: 22430496
- PMCID: PMC3389460
- DOI: 10.1016/j.neuroimage.2012.02.084
Within-subject template estimation for unbiased longitudinal image analysis
Abstract
Longitudinal image analysis has become increasingly important in clinical studies of normal aging and neurodegenerative disorders. Furthermore, there is a growing appreciation of the potential utility of longitudinally acquired structural images and reliable image processing to evaluate disease modifying therapies. Challenges have been related to the variability that is inherent in the available cross-sectional processing tools, to the introduction of bias in longitudinal processing and to potential over-regularization. In this paper we introduce a novel longitudinal image processing framework, based on unbiased, robust, within-subject template creation, for automatic surface reconstruction and segmentation of brain MRI of arbitrarily many time points. We demonstrate that it is essential to treat all input images exactly the same as removing only interpolation asymmetries is not sufficient to remove processing bias. We successfully reduce variability and avoid over-regularization by initializing the processing in each time point with common information from the subject template. The presented results show a significant increase in precision and discrimination power while preserving the ability to detect large anatomical deviations; as such they hold great potential in clinical applications, e.g. allowing for smaller sample sizes or shorter trials to establish disease specific biomarkers or to quantify drug effects.
Copyright © 2012 Elsevier Inc. All rights reserved.
Figures
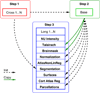
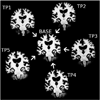
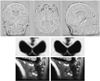
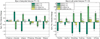
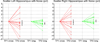
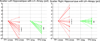
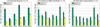
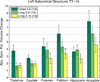
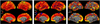
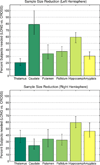
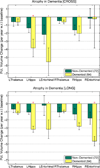
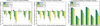
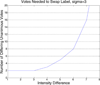
Similar articles
-
Brain morphometry reproducibility in multi-center 3T MRI studies: a comparison of cross-sectional and longitudinal segmentations.Neuroimage. 2013 Dec;83:472-84. doi: 10.1016/j.neuroimage.2013.05.007. Epub 2013 May 11. Neuroimage. 2013. PMID: 23668971
-
A novel longitudinal atlas construction framework by groupwise registration of subject image sequences.Inf Process Med Imaging. 2011;22:283-95. doi: 10.1007/978-3-642-22092-0_24. Inf Process Med Imaging. 2011. PMID: 21761664 Free PMC article.
-
A novel framework for longitudinal atlas construction with groupwise registration of subject image sequences.Neuroimage. 2012 Jan 16;59(2):1275-89. doi: 10.1016/j.neuroimage.2011.07.095. Epub 2011 Aug 22. Neuroimage. 2012. PMID: 21884801 Free PMC article.
-
Sparse unbiased analysis of anatomical variance in longitudinal imaging.Med Image Comput Comput Assist Interv. 2010;13(Pt 1):324-31. doi: 10.1007/978-3-642-15705-9_40. Med Image Comput Comput Assist Interv. 2010. PMID: 20879247 Free PMC article.
-
Brain functional localization: a survey of image registration techniques.IEEE Trans Med Imaging. 2007 Apr;26(4):427-51. doi: 10.1109/TMI.2007.892508. IEEE Trans Med Imaging. 2007. PMID: 17427731 Review.
Cited by
-
Brain Volumetric Analysis Using Artificial Intelligence Software in Premanifest Huntington's Disease Individuals from a Colombian Caribbean Population.Biomedicines. 2024 Sep 24;12(10):2166. doi: 10.3390/biomedicines12102166. Biomedicines. 2024. PMID: 39457479 Free PMC article.
-
Selective Brain Activations and Connectivities Related to the Storage and Recall of Human Object-Location, Reward-Location, and Word-Pair Episodic Memories.Hum Brain Mapp. 2024 Oct 15;45(15):e70056. doi: 10.1002/hbm.70056. Hum Brain Mapp. 2024. PMID: 39436048 Free PMC article.
-
Plasma markers predict changes in amyloid, tau, atrophy and cognition in non-demented subjects.Brain. 2021 Oct 22;144(9):2826-2836. doi: 10.1093/brain/awab163. Brain. 2021. PMID: 34077494 Free PMC article. Clinical Trial.
-
Estimating anatomical trajectories with Bayesian mixed-effects modeling.Neuroimage. 2015 Nov 1;121:51-68. doi: 10.1016/j.neuroimage.2015.06.094. Epub 2015 Jul 17. Neuroimage. 2015. PMID: 26190405 Free PMC article.
-
Inferior Prefrontal Cortex Mediates the Relationship between Phosphatidylcholine and Executive Functions in Healthy, Older Adults.Front Aging Neurosci. 2016 Sep 28;8:226. doi: 10.3389/fnagi.2016.00226. eCollection 2016. Front Aging Neurosci. 2016. PMID: 27733825 Free PMC article.
References
-
- Ashburner J. A fast diffeomorphic image registration algorithm URL. NeuroImage. 2007;38(1):95–113. http://www.sciencedirect.com/science/article/pii/S1053811907005848. - PubMed
-
- Avants B, Gee JC. Geodesic estimation for large deformation anatomical shape averaging and interpolation. NeuroImage. 2004;23(1):139–150. - PubMed
-
- Avants B, Anderson C, Grossman M, Gee JC. Spatiotemporal normalization for longitudinal analysis of gray matter atrophy in frontotemporal dementia. Medical Image Computing and Computer-Assisted Intervention - MICCAI 2007: Vol. 4792 of Lecture Notes in Computer Science. 2007:303–310. - PubMed
Publication types
MeSH terms
Grants and funding
- R01 NS070963/NS/NINDS NIH HHS/United States
- P01 NS058793/NS/NINDS NIH HHS/United States
- R01EB006758/EB/NIBIB NIH HHS/United States
- R01 NS052585-05/NS/NINDS NIH HHS/United States
- P50MH071616/MH/NIMH NIH HHS/United States
- U24RR021382/RR/NCRR NIH HHS/United States
- S10 RR023043-01/RR/NCRR NIH HHS/United States
- R01 AG021910/AG/NIA NIH HHS/United States
- R01 AG021910-05/AG/NIA NIH HHS/United States
- P41 RR014075-13/RR/NCRR NIH HHS/United States
- U01 AG024904-07/AG/NIA NIH HHS/United States
- R01AG021910/AG/NIA NIH HHS/United States
- R21 NS072652/NS/NINDS NIH HHS/United States
- S10 RR023401/RR/NCRR NIH HHS/United States
- R01 NS070963-02/NS/NINDS NIH HHS/United States
- U24 RR021382/RR/NCRR NIH HHS/United States
- R01 AG018386/AG/NIA NIH HHS/United States
- R21 NS072652-02/NS/NINDS NIH HHS/United States
- R01 AG022381-10/AG/NIA NIH HHS/United States
- R01 NS042861-09/NS/NINDS NIH HHS/United States
- R01 MH056584/MH/NIMH NIH HHS/United States
- R01 EB006758-04/EB/NIBIB NIH HHS/United States
- S10RR023043/RR/NCRR NIH HHS/United States
- U01 AG024904/AG/NIA NIH HHS/United States
- R01NS052585/NS/NINDS NIH HHS/United States
- S10 RR019307-01/RR/NCRR NIH HHS/United States
- U01AG024904/AG/NIA NIH HHS/United States
- S10 RR019307/RR/NCRR NIH HHS/United States
- P01 AG003991/AG/NIA NIH HHS/United States
- RC1AT005728/AT/NCCIH NIH HHS/United States
- P50 AG005681/AG/NIA NIH HHS/United States
- P01 NS058793-05/NS/NINDS NIH HHS/United States
- P01 AG003991-25/AG/NIA NIH HHS/United States
- S10RR023401/RR/NCRR NIH HHS/United States
- P41 RR014075/RR/NCRR NIH HHS/United States
- P01AG03991/AG/NIA NIH HHS/United States
- R01 NS052585/NS/NINDS NIH HHS/United States
- U24 RR021382-05/RR/NCRR NIH HHS/United States
- R01 MH056584-12/MH/NIMH NIH HHS/United States
- R01AG022381/AG/NIA NIH HHS/United States
- P01NS058793/NS/NINDS NIH HHS/United States
- R01 NS042861/NS/NINDS NIH HHS/United States
- R01MH56584/MH/NIMH NIH HHS/United States
- RC1 AT005728/AT/NCCIH NIH HHS/United States
- R01NS070963/NS/NINDS NIH HHS/United States
- S10RR019307/RR/NCRR NIH HHS/United States
- S10 RR023043/RR/NCRR NIH HHS/United States
- R01NS042861/NS/NINDS NIH HHS/United States
- P50 MH071616/MH/NIMH NIH HHS/United States
- P50AG05681/AG/NIA NIH HHS/United States
- R21NS072652/NS/NINDS NIH HHS/United States
- RC1 AT005728-02/AT/NCCIH NIH HHS/United States
- R01 EB006758/EB/NIBIB NIH HHS/United States
- P41RR14075/RR/NCRR NIH HHS/United States
- S10 RR023401-01A2/RR/NCRR NIH HHS/United States
- P50 MH071616-05/MH/NIMH NIH HHS/United States
- R01 AG022381/AG/NIA NIH HHS/United States
LinkOut - more resources
Full Text Sources
Other Literature Sources
Medical