Speed/accuracy trade-off between the habitual and the goal-directed processes
- PMID: 21637741
- PMCID: PMC3102758
- DOI: 10.1371/journal.pcbi.1002055
Speed/accuracy trade-off between the habitual and the goal-directed processes
Abstract
Instrumental responses are hypothesized to be of two kinds: habitual and goal-directed, mediated by the sensorimotor and the associative cortico-basal ganglia circuits, respectively. The existence of the two heterogeneous associative learning mechanisms can be hypothesized to arise from the comparative advantages that they have at different stages of learning. In this paper, we assume that the goal-directed system is behaviourally flexible, but slow in choice selection. The habitual system, in contrast, is fast in responding, but inflexible in adapting its behavioural strategy to new conditions. Based on these assumptions and using the computational theory of reinforcement learning, we propose a normative model for arbitration between the two processes that makes an approximately optimal balance between search-time and accuracy in decision making. Behaviourally, the model can explain experimental evidence on behavioural sensitivity to outcome at the early stages of learning, but insensitivity at the later stages. It also explains that when two choices with equal incentive values are available concurrently, the behaviour remains outcome-sensitive, even after extensive training. Moreover, the model can explain choice reaction time variations during the course of learning, as well as the experimental observation that as the number of choices increases, the reaction time also increases. Neurobiologically, by assuming that phasic and tonic activities of midbrain dopamine neurons carry the reward prediction error and the average reward signals used by the model, respectively, the model predicts that whereas phasic dopamine indirectly affects behaviour through reinforcing stimulus-response associations, tonic dopamine can directly affect behaviour through manipulating the competition between the habitual and the goal-directed systems and thus, affect reaction time.
Conflict of interest statement
The authors have declared that no competing interests exist.
Figures
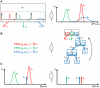



















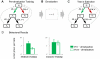





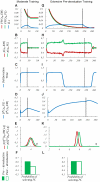


























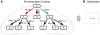










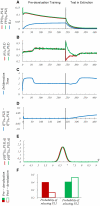











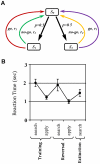















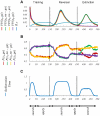










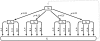


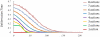
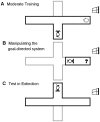
Similar articles
-
Goal-Directed Decision Making with Spiking Neurons.J Neurosci. 2016 Feb 3;36(5):1529-46. doi: 10.1523/JNEUROSCI.2854-15.2016. J Neurosci. 2016. PMID: 26843636 Free PMC article.
-
Navigating complex decision spaces: Problems and paradigms in sequential choice.Psychol Bull. 2014 Mar;140(2):466-86. doi: 10.1037/a0033455. Epub 2013 Jul 8. Psychol Bull. 2014. PMID: 23834192 Free PMC article. Review.
-
Striatal dopamine ramping may indicate flexible reinforcement learning with forgetting in the cortico-basal ganglia circuits.Front Neural Circuits. 2014 Apr 9;8:36. doi: 10.3389/fncir.2014.00036. eCollection 2014. Front Neural Circuits. 2014. PMID: 24782717 Free PMC article.
-
A neural network model with dopamine-like reinforcement signal that learns a spatial delayed response task.Neuroscience. 1999;91(3):871-90. doi: 10.1016/s0306-4522(98)00697-6. Neuroscience. 1999. PMID: 10391468
-
Cost, benefit, tonic, phasic: what do response rates tell us about dopamine and motivation?Ann N Y Acad Sci. 2007 May;1104:357-76. doi: 10.1196/annals.1390.018. Epub 2007 Apr 7. Ann N Y Acad Sci. 2007. PMID: 17416928 Review.
Cited by
-
Effects of subclinical depression on prefrontal-striatal model-based and model-free learning.PLoS Comput Biol. 2021 May 14;17(5):e1009003. doi: 10.1371/journal.pcbi.1009003. eCollection 2021 May. PLoS Comput Biol. 2021. PMID: 33989284 Free PMC article.
-
Reduced model-based decision-making in gambling disorder.Sci Rep. 2019 Dec 23;9(1):19625. doi: 10.1038/s41598-019-56161-z. Sci Rep. 2019. PMID: 31873133 Free PMC article. Clinical Trial.
-
Model-Based and Model-Free Replay Mechanisms for Reinforcement Learning in Neurorobotics.Front Neurorobot. 2022 Jun 24;16:864380. doi: 10.3389/fnbot.2022.864380. eCollection 2022. Front Neurorobot. 2022. PMID: 35812782 Free PMC article.
-
Modulating Visuomotor Sequence Learning by Repetitive Transcranial Magnetic Stimulation: What Do We Know So Far?J Intell. 2023 Oct 13;11(10):201. doi: 10.3390/jintelligence11100201. J Intell. 2023. PMID: 37888433 Free PMC article. Review.
-
Action-value comparisons in the dorsolateral prefrontal cortex control choice between goal-directed actions.Nat Commun. 2014 Jul 23;5:4390. doi: 10.1038/ncomms5390. Nat Commun. 2014. PMID: 25055179 Free PMC article.
References
-
- Dickinson A, Balleine BW. The role of learning in motivation. In: Gallistel CR, editor. Steven's Handbook of Experimental Psychology: Learning, Motivation, and Emotion. New York: Wiley; 2002. pp. 497–533. Volume 3. 3rd edition.
-
- Adams CD. Variations in the sensitivity of instrumental responding to reinforcer devaluation. Q J Exp Psychol. 1982;34:77–98.
-
- Daw ND, Niv Y, Dayan P. Uncertainty-based competition between prefrontal and dorsolateral striatal systems for behavioral control. Nat Neurosci. 2005;8:1704–11. - PubMed
MeSH terms
Substances
LinkOut - more resources
Full Text Sources
Molecular Biology Databases