Development and evaluation of a computer-aided diagnostic scheme for lung nodule detection in chest radiographs by means of two-stage nodule enhancement with support vector classification
- PMID: 21626918
- PMCID: PMC3069992
- DOI: 10.1118/1.3561504
Development and evaluation of a computer-aided diagnostic scheme for lung nodule detection in chest radiographs by means of two-stage nodule enhancement with support vector classification
Abstract
Purpose: To develop a computer-aided detection (CADe) scheme for nodules in chest radiographs (CXRs) with a high sensitivity and a low false-positive (FP) rate.
Methods: The authors developed a CADe scheme consisting of five major steps, which were developed for improving the overall performance of CADe schemes. First, to segment the lung fields accurately, the authors developed a multisegment active shape model. Then, a two-stage nodule-enhancement technique was developed for improving the conspicuity of nodules. Initial nodule candidates were detected and segmented by using the clustering watershed algorithm. Thirty-one shape-, gray-level-, surface-, and gradient-based features were extracted from each segmented candidate for determining the feature space, including one of the new features based on the Canny edge detector to eliminate a major FP source caused by rib crossings. Finally, a nonlinear support vector machine (SVM) with a Gaussian kernel was employed for classification of the nodule candidates.
Results: To evaluate and compare the scheme to other published CADe schemes, the authors used a publicly available database containing 140 nodules in 140 CXRs and 93 normal CXRs. The CADe scheme based on the SVM classifier achieved sensitivities of 78.6% (110/140) and 71.4% (100/140) with averages of 5.0 (1165/233) FPs/image and 2.0 (466/233) FPs/image, respectively, in a leave-one-out cross-validation test, whereas the CADe scheme based on a linear discriminant analysis classifier had a sensitivity of 60.7% (85/140) at an FP rate of 5.0 FPs/image. For nodules classified as "very subtle" and "extremely subtle," a sensitivity of 57.1% (24/42) was achieved at an FP rate of 5.0 FPs/image. When the authors used a database developed at the University of Chicago, the sensitivities was 83.3% (40/48) and 77.1% (37/48) at an FP rate of 5.0 (240/48) FPs/image and 2.0 (96/48) FPs/image, respectively.
Conclusions: These results compare favorably to those described for other commercial and non-commercial CADe nodule detection systems.
Figures
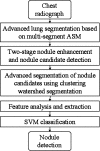
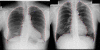
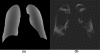
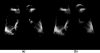

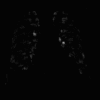
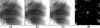
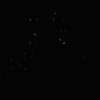
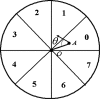
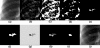
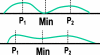
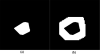
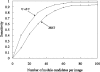
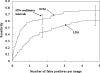
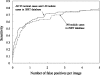
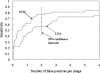
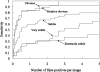
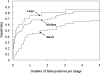
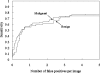
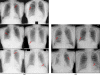
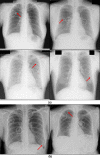
Similar articles
-
Computerized detection of lung nodules by means of "virtual dual-energy" radiography.IEEE Trans Biomed Eng. 2013 Feb;60(2):369-78. doi: 10.1109/TBME.2012.2226583. Epub 2012 Nov 15. IEEE Trans Biomed Eng. 2013. PMID: 23193306 Free PMC article.
-
Computer-aided diagnostic scheme for the detection of lung nodules on chest radiographs: localized search method based on anatomical classification.Med Phys. 2006 Jul;33(7):2642-53. doi: 10.1118/1.2208739. Med Phys. 2006. PMID: 16898468
-
A parameterized logarithmic image processing method with Laplacian of Gaussian filtering for lung nodule enhancement in chest radiographs.Med Biol Eng Comput. 2016 Nov;54(11):1793-1806. doi: 10.1007/s11517-016-1469-x. Epub 2016 Mar 25. Med Biol Eng Comput. 2016. PMID: 27016368
-
Computer-aided detection system for lung cancer in computed tomography scans: review and future prospects.Biomed Eng Online. 2014 Apr 8;13:41. doi: 10.1186/1475-925X-13-41. Biomed Eng Online. 2014. PMID: 24713067 Free PMC article. Review.
-
Computer-aided detection in chest radiography based on artificial intelligence: a survey.Biomed Eng Online. 2018 Aug 22;17(1):113. doi: 10.1186/s12938-018-0544-y. Biomed Eng Online. 2018. PMID: 30134902 Free PMC article. Review.
Cited by
-
Review: On Segmentation of Nodules from Posterior and Anterior Chest Radiographs.Int J Biomed Imaging. 2018 Oct 18;2018:9752638. doi: 10.1155/2018/9752638. eCollection 2018. Int J Biomed Imaging. 2018. PMID: 30498510 Free PMC article.
-
Overview of deep learning in medical imaging.Radiol Phys Technol. 2017 Sep;10(3):257-273. doi: 10.1007/s12194-017-0406-5. Epub 2017 Jul 8. Radiol Phys Technol. 2017. PMID: 28689314 Review.
-
Computerized detection of lung nodules by means of "virtual dual-energy" radiography.IEEE Trans Biomed Eng. 2013 Feb;60(2):369-78. doi: 10.1109/TBME.2012.2226583. Epub 2012 Nov 15. IEEE Trans Biomed Eng. 2013. PMID: 23193306 Free PMC article.
-
Lung Segmentation using Active Shape Model to Detect the Disease from Chest Radiography.J Biomed Phys Eng. 2021 Dec 1;11(6):747-756. doi: 10.31661/jbpe.v0i0.2105-1346. eCollection 2021 Dec. J Biomed Phys Eng. 2021. PMID: 34904071 Free PMC article.
-
Computer-assisted diagnosis for an early identification of lung cancer in chest X rays.Sci Rep. 2023 May 12;13(1):7720. doi: 10.1038/s41598-023-34835-z. Sci Rep. 2023. PMID: 37173327 Free PMC article.
References
-
- American Cancer Society, American Cancer Society Complete Guide to Complementary & Alternative Cancer Therapies, 2nd ed. (American Cancer Society, Atlanta, GA, 2009).
-
- Murphy G. P.et al., American Cancer Society Textbook of Clinical Oncology, 2nd ed. (The Society, Atlanta, GA, 1995).
Publication types
MeSH terms
Grants and funding
LinkOut - more resources
Full Text Sources
Other Literature Sources
Medical
Miscellaneous