Network clustering: probing biological heterogeneity by sparse graphical models
- PMID: 21317141
- PMCID: PMC3065697
- DOI: 10.1093/bioinformatics/btr070
Network clustering: probing biological heterogeneity by sparse graphical models
Abstract
Motivation: Networks and pathways are important in describing the collective biological function of molecular players such as genes or proteins. In many areas of biology, for example in cancer studies, available data may harbour undiscovered subtypes which differ in terms of network phenotype. That is, samples may be heterogeneous with respect to underlying molecular networks. This motivates a need for unsupervised methods capable of discovering such subtypes and elucidating the corresponding network structures.
Results: We exploit recent results in sparse graphical model learning to put forward a 'network clustering' approach in which data are partitioned into subsets that show evidence of underlying, subset-level network structure. This allows us to simultaneously learn subset-specific networks and corresponding subset membership under challenging small-sample conditions. We illustrate this approach on synthetic and proteomic data.
Availability: go.warwick.ac.uk/sachmukherjee/networkclustering.
Figures
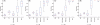
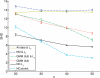
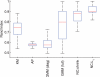
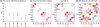
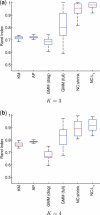
Similar articles
-
Cancer subtype discovery and biomarker identification via a new robust network clustering algorithm.PLoS One. 2013 Jun 17;8(6):e66256. doi: 10.1371/journal.pone.0066256. Print 2013. PLoS One. 2013. PMID: 23799085 Free PMC article.
-
Generic, network schema agnostic sparse tensor factorization for single-pass clustering of heterogeneous information networks.PLoS One. 2017 Feb 28;12(2):e0172323. doi: 10.1371/journal.pone.0172323. eCollection 2017. PLoS One. 2017. PMID: 28245222 Free PMC article.
-
Wrangling phosphoproteomic data to elucidate cancer signaling pathways.PLoS One. 2013;8(1):e52884. doi: 10.1371/journal.pone.0052884. Epub 2013 Jan 3. PLoS One. 2013. PMID: 23300999 Free PMC article.
-
Functional genomics and proteomics in the clinical neurosciences: data mining and bioinformatics.Prog Brain Res. 2006;158:83-108. doi: 10.1016/S0079-6123(06)58004-5. Prog Brain Res. 2006. PMID: 17027692 Review.
-
Proteomic and computational methods in systems modeling of cellular signaling.Curr Pharm Biotechnol. 2006 Jun;7(3):135-45. doi: 10.2174/138920106777549722. Curr Pharm Biotechnol. 2006. PMID: 16789899 Review.
Cited by
-
Cancer subtype discovery and biomarker identification via a new robust network clustering algorithm.PLoS One. 2013 Jun 17;8(6):e66256. doi: 10.1371/journal.pone.0066256. Print 2013. PLoS One. 2013. PMID: 23799085 Free PMC article.
-
Distinctive properties of biological neural networks and recent advances in bottom-up approaches toward a better biologically plausible neural network.Front Comput Neurosci. 2023 Jun 28;17:1092185. doi: 10.3389/fncom.2023.1092185. eCollection 2023. Front Comput Neurosci. 2023. PMID: 37449083 Free PMC article. Review.
-
Deriving a Boolean dynamics to reveal macrophage activation with in vitro temporal cytokine expression profiles.BMC Bioinformatics. 2019 Dec 18;20(1):725. doi: 10.1186/s12859-019-3304-5. BMC Bioinformatics. 2019. PMID: 31852428 Free PMC article.
-
Stochastic blockmodeling of the modules and core of the Caenorhabditis elegans connectome.PLoS One. 2014 Jul 2;9(7):e97584. doi: 10.1371/journal.pone.0097584. eCollection 2014. PLoS One. 2014. PMID: 24988196 Free PMC article.
-
Learning complex dependency structure of gene regulatory networks from high dimensional microarray data with Gaussian Bayesian networks.Sci Rep. 2022 Nov 4;12(1):18704. doi: 10.1038/s41598-022-21957-z. Sci Rep. 2022. PMID: 36333425 Free PMC article.
References
-
- Banerjee O., et al. Model selection through sparse maximum likelihood estimation for multivariate Gaussian or binary data. J. Mach. Learn. Res. 2008;9:485–516.
-
- Dempster A.P. Covariance selection. Biometrics. 1972;28:157–175.
-
- Dobra A., et al. Sparse graphical models for exploring gene expression data. J. Multivar. Anal. 2004;90:196–212.
-
- Frey B.J., Dueck D. Clustering by passing messages between data points. Science. 2007;315:972–976. - PubMed