Structure learning in human sequential decision-making
- PMID: 21151963
- PMCID: PMC2996460
- DOI: 10.1371/journal.pcbi.1001003
Structure learning in human sequential decision-making
Abstract
Studies of sequential decision-making in humans frequently find suboptimal performance relative to an ideal actor that has perfect knowledge of the model of how rewards and events are generated in the environment. Rather than being suboptimal, we argue that the learning problem humans face is more complex, in that it also involves learning the structure of reward generation in the environment. We formulate the problem of structure learning in sequential decision tasks using Bayesian reinforcement learning, and show that learning the generative model for rewards qualitatively changes the behavior of an optimal learning agent. To test whether people exhibit structure learning, we performed experiments involving a mixture of one-armed and two-armed bandit reward models, where structure learning produces many of the qualitative behaviors deemed suboptimal in previous studies. Our results demonstrate humans can perform structure learning in a near-optimal manner.
Conflict of interest statement
The authors have declared that no competing interests exist.
Figures
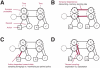
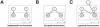



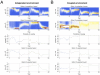


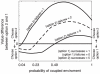





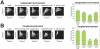

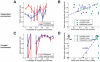
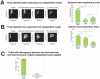
Similar articles
-
Reinforcement learning signals in the human striatum distinguish learners from nonlearners during reward-based decision making.J Neurosci. 2007 Nov 21;27(47):12860-7. doi: 10.1523/JNEUROSCI.2496-07.2007. J Neurosci. 2007. PMID: 18032658 Free PMC article.
-
Mouse tracking reveals structure knowledge in the absence of model-based choice.Nat Commun. 2020 Apr 20;11(1):1893. doi: 10.1038/s41467-020-15696-w. Nat Commun. 2020. PMID: 32312966 Free PMC article.
-
Overtaking method based on sand-sifter mechanism: Why do optimistic value functions find optimal solutions in multi-armed bandit problems?Biosystems. 2015 Sep;135:55-65. doi: 10.1016/j.biosystems.2015.06.009. Epub 2015 Jul 10. Biosystems. 2015. PMID: 26166266
-
[Mathematical models of decision making and learning].Brain Nerve. 2008 Jul;60(7):791-8. Brain Nerve. 2008. PMID: 18646619 Review. Japanese.
-
Reinforcement learning: the good, the bad and the ugly.Curr Opin Neurobiol. 2008 Apr;18(2):185-96. doi: 10.1016/j.conb.2008.08.003. Epub 2008 Aug 22. Curr Opin Neurobiol. 2008. PMID: 18708140 Review.
Cited by
-
Structure learning and the Occam's razor principle: a new view of human function acquisition.Front Comput Neurosci. 2014 Sep 30;8:121. doi: 10.3389/fncom.2014.00121. eCollection 2014. Front Comput Neurosci. 2014. PMID: 25324770 Free PMC article.
-
Models that learn how humans learn: The case of decision-making and its disorders.PLoS Comput Biol. 2019 Jun 11;15(6):e1006903. doi: 10.1371/journal.pcbi.1006903. eCollection 2019 Jun. PLoS Comput Biol. 2019. PMID: 31185008 Free PMC article.
-
A Bayesian Account of Generalist and Specialist Formation Under the Active Inference Framework.Front Artif Intell. 2020 Sep 3;3:69. doi: 10.3389/frai.2020.00069. eCollection 2020. Front Artif Intell. 2020. PMID: 33733186 Free PMC article.
-
A Bayesian model of context-sensitive value attribution.Elife. 2016 Jun 22;5:e16127. doi: 10.7554/eLife.16127. Elife. 2016. PMID: 27328323 Free PMC article.
-
Model averaging, optimal inference, and habit formation.Front Hum Neurosci. 2014 Jun 26;8:457. doi: 10.3389/fnhum.2014.00457. eCollection 2014. Front Hum Neurosci. 2014. PMID: 25018724 Free PMC article.
References
-
- Bellman RE. A problem in the sequential design of experiments. Sankhyā. 1956;16:221–229.
-
- Gittins JC. Multi-armed bandit allocation indices. Chichester [West Sussex]; New York: Wiley; 1989.
-
- Whittle P. Restless bandits: activity allocation in a changing world. J Appl Probab. 1988;25:287–298.
-
- Yi MS, Steyvers M, Lee M. Modeling human performance in restless bandits with particle filters. The Journal of Problem Solving. 2009;2 Available: http://docs.lib.purdue.edu/jps/vol2/iss2/5/
Publication types
MeSH terms
Grants and funding
LinkOut - more resources
Full Text Sources