Evolution and optimality of similar neural mechanisms for perception and action during search
- PMID: 20838589
- PMCID: PMC2936525
- DOI: 10.1371/journal.pcbi.1000930
Evolution and optimality of similar neural mechanisms for perception and action during search
Abstract
A prevailing theory proposes that the brain's two visual pathways, the ventral and dorsal, lead to differing visual processing and world representations for conscious perception than those for action. Others have claimed that perception and action share much of their visual processing. But which of these two neural architectures is favored by evolution? Successful visual search is life-critical and here we investigate the evolution and optimality of neural mechanisms mediating perception and eye movement actions for visual search in natural images. We implement an approximation to the ideal Bayesian searcher with two separate processing streams, one controlling the eye movements and the other stream determining the perceptual search decisions. We virtually evolved the neural mechanisms of the searchers' two separate pathways built from linear combinations of primary visual cortex receptive fields (V1) by making the simulated individuals' probability of survival depend on the perceptual accuracy finding targets in cluttered backgrounds. We find that for a variety of targets, backgrounds, and dependence of target detectability on retinal eccentricity, the mechanisms of the searchers' two processing streams converge to similar representations showing that mismatches in the mechanisms for perception and eye movements lead to suboptimal search. Three exceptions which resulted in partial or no convergence were a case of an organism for which the targets are equally detectable across the retina, an organism with sufficient time to foveate all possible target locations, and a strict two-pathway model with no interconnections and differential pre-filtering based on parvocellular and magnocellular lateral geniculate cell properties. Thus, similar neural mechanisms for perception and eye movement actions during search are optimal and should be expected from the effects of natural selection on an organism with limited time to search for food that is not equi-detectable across its retina and interconnected perception and action neural pathways.
Conflict of interest statement
The authors have declared that no competing interests exist.
Figures
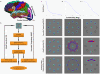
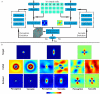
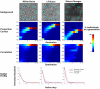
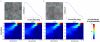
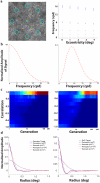
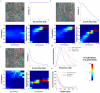
Similar articles
-
Similar neural representations of the target for saccades and perception during search.J Neurosci. 2007 Feb 7;27(6):1266-70. doi: 10.1523/JNEUROSCI.3975-06.2007. J Neurosci. 2007. PMID: 17287501 Free PMC article.
-
Remapping in human visual cortex.J Neurophysiol. 2007 Feb;97(2):1738-55. doi: 10.1152/jn.00189.2006. Epub 2006 Nov 8. J Neurophysiol. 2007. PMID: 17093130 Free PMC article.
-
Virtual evolution for visual search in natural images results in behavioral receptive fields with inhibitory surrounds.Vis Neurosci. 2009 Jan-Feb;26(1):93-108. doi: 10.1017/S0952523809090014. Epub 2009 Mar 12. Vis Neurosci. 2009. PMID: 19278570
-
3-D vision and figure-ground separation by visual cortex.Percept Psychophys. 1994 Jan;55(1):48-121. doi: 10.3758/bf03206880. Percept Psychophys. 1994. PMID: 8036093 Review.
-
Is visual processing in the dorsal stream accessible to consciousness?Proc Biol Sci. 2012 Jun 22;279(1737):2289-98. doi: 10.1098/rspb.2011.2663. Epub 2012 Mar 28. Proc Biol Sci. 2012. PMID: 22456882 Free PMC article. Review.
Cited by
-
Optimal and human eye movements to clustered low value cues to increase decision rewards during search.Vision Res. 2015 Aug;113(Pt B):137-54. doi: 10.1016/j.visres.2015.05.016. Epub 2015 Jun 17. Vision Res. 2015. PMID: 26093154 Free PMC article.
-
Object detection through search with a foveated visual system.PLoS Comput Biol. 2017 Oct 9;13(10):e1005743. doi: 10.1371/journal.pcbi.1005743. eCollection 2017 Oct. PLoS Comput Biol. 2017. PMID: 28991906 Free PMC article.
-
Gambling in the visual periphery: a conjoint-measurement analysis of human ability to judge visual uncertainty.PLoS Comput Biol. 2010 Dec 2;6(12):e1001023. doi: 10.1371/journal.pcbi.1001023. PLoS Comput Biol. 2010. PMID: 21152007 Free PMC article.
-
Stop before you saccade: Looking into an artificial peripheral scotoma.J Vis. 2015;15(5):7. doi: 10.1167/15.5.7. J Vis. 2015. PMID: 26067525 Free PMC article.
-
Hiding the Rabbit: Using a genetic algorithm to investigate shape guidance in visual search.J Vis. 2022 Jan 4;22(1):7. doi: 10.1167/jov.22.1.7. J Vis. 2022. PMID: 35024760 Free PMC article.
References
-
- Ungerleider LG, Mishkin M. Two cortical visual systems. In: Ingle DJ, Goodale MA, Mansfield RW, editors. Analysis of Visual Behavior. Cambridge, Massachusetts: MIT Press; 1982. pp. 549–586.
-
- Sakata H, Taira M, Kusunoki M, Murata A, Tanaka Y. The parietal association cortex in depth perception and visual control of hand action. Trends Neurosci. 1997;20:350–357. - PubMed
-
- Snyder LH, Batista AP, Andersen RA. Coding of intention in the posterior parietal cortex. Nature. 1997;386:167–170. - PubMed
-
- Goodale MA, Milner AD, Jakobson LS, Carey DP. A neurological dissociation between perceiving objects and grasping them. Nature. 1991;349:154–156. - PubMed
-
- Goodale MA, Milner AD. Separate visual pathways for perception and action. Trends Neurosci. 1992;15:20–25. - PubMed
Publication types
MeSH terms
LinkOut - more resources
Full Text Sources