A spatiotemporal atlas of MR intensity, tissue probability and shape of the fetal brain with application to segmentation
- PMID: 20600970
- PMCID: PMC2930902
- DOI: 10.1016/j.neuroimage.2010.06.054
A spatiotemporal atlas of MR intensity, tissue probability and shape of the fetal brain with application to segmentation
Abstract
Modeling and analysis of MR images of the developing human brain is a challenge due to rapid changes in brain morphology and morphometry. We present an approach to the construction of a spatiotemporal atlas of the fetal brain with temporal models of MR intensity, tissue probability and shape changes. This spatiotemporal model is created from a set of reconstructed MR images of fetal subjects with different gestational ages. Groupwise registration of manual segmentations and voxelwise nonlinear modeling allow us to capture the appearance, disappearance and spatial variation of brain structures over time. Applying this model to atlas-based segmentation, we generate age-specific MR templates and tissue probability maps and use them to initialize automatic tissue delineation in new MR images. The choice of model parameters and the final performance are evaluated using clinical MR scans of young fetuses with gestational ages ranging from 20.57 to 24.71 weeks. Experimental results indicate that quadratic temporal models can correctly capture growth-related changes in the fetal brain anatomy and provide improvement in accuracy of atlas-based tissue segmentation.
Copyright 2010 Elsevier Inc. All rights reserved.
Figures
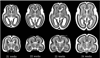
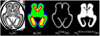
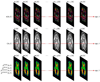
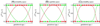
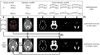
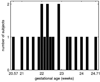
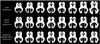
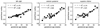
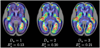
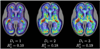
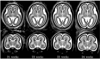
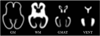
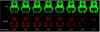
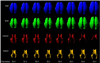
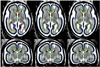
Similar articles
-
A spatio-temporal atlas of the human fetal brain with application to tissue segmentation.Med Image Comput Comput Assist Interv. 2009;12(Pt 1):289-96. doi: 10.1007/978-3-642-04268-3_36. Med Image Comput Comput Assist Interv. 2009. PMID: 20425999 Free PMC article.
-
A normative spatiotemporal MRI atlas of the fetal brain for automatic segmentation and analysis of early brain growth.Sci Rep. 2017 Mar 28;7(1):476. doi: 10.1038/s41598-017-00525-w. Sci Rep. 2017. PMID: 28352082 Free PMC article.
-
Atlas-based segmentation of developing tissues in the human brain with quantitative validation in young fetuses.Hum Brain Mapp. 2010 Sep;31(9):1348-58. doi: 10.1002/hbm.20935. Hum Brain Mapp. 2010. PMID: 20108226 Free PMC article.
-
Fetal brain growth portrayed by a spatiotemporal diffusion tensor MRI atlas computed from in utero images.Neuroimage. 2019 Jan 15;185:593-608. doi: 10.1016/j.neuroimage.2018.08.030. Epub 2018 Aug 30. Neuroimage. 2019. PMID: 30172006 Free PMC article. Review.
-
Atlas learning in fetal brain development.Top Magn Reson Imaging. 2011 Jun;22(3):107-11. doi: 10.1097/RMR.0b013e318267fe94. Top Magn Reson Imaging. 2011. PMID: 23558465 Review.
Cited by
-
Quantifying and modelling tissue maturation in the living human fetal brain.Int J Dev Neurosci. 2014 Feb;32:3-10. doi: 10.1016/j.ijdevneu.2013.06.006. Epub 2013 Jul 3. Int J Dev Neurosci. 2014. PMID: 23831076 Free PMC article. Review.
-
Spatial-temporal atlas of human fetal brain development during the early second trimester.Neuroimage. 2013 Nov 15;82:115-26. doi: 10.1016/j.neuroimage.2013.05.063. Epub 2013 May 31. Neuroimage. 2013. PMID: 23727529 Free PMC article.
-
Construction of 4D high-definition cortical surface atlases of infants: Methods and applications.Med Image Anal. 2015 Oct;25(1):22-36. doi: 10.1016/j.media.2015.04.005. Epub 2015 Apr 17. Med Image Anal. 2015. PMID: 25980388 Free PMC article.
-
A discriminative feature selection approach for shape analysis: Application to fetal brain cortical folding.Med Image Anal. 2017 Jan;35:313-326. doi: 10.1016/j.media.2016.07.005. Epub 2016 Jul 25. Med Image Anal. 2017. PMID: 27498089 Free PMC article.
-
Functional Connectivity of the Human Brain in Utero.Trends Cogn Sci. 2016 Dec;20(12):931-939. doi: 10.1016/j.tics.2016.10.001. Epub 2016 Nov 4. Trends Cogn Sci. 2016. PMID: 27825537 Free PMC article. Review.
References
-
- Ashburner J, Friston KJ. Unified segmentation. Neuroimage. 2005;26(3):839–851. - PubMed
-
- Bajcsy R, Lieberson R, Reivich M. A computerized system for the elastic matching of deformed radiographic images to idealized atlas images. J. Comput. Assist. Tomogr. 1983;7(4):618–625. - PubMed
-
- Battin MR, Maalouf EF, Counsell SJ, Herlihy AH, Rutherford MA, Azzopardi D, Edwards AD. Magnetic resonance imaging of the brain in very preterm infants: visualization of the germinal matrix, early myelination, and cortical folding. Pediatrics. 1998;101(6):957–962. - PubMed
-
- Davis BC, Fletcher PT, Bullitt E, Joshi SC. Population shape regression from random design data; Proc. International Conference on Computer Vision; 2007. pp. 1–7.
-
- Dice LR. Measures of the amount of ecologic association between species. Ecology. 1945;26(3):297–302.
Publication types
MeSH terms
Grants and funding
LinkOut - more resources
Full Text Sources
Other Literature Sources
Medical