States versus rewards: dissociable neural prediction error signals underlying model-based and model-free reinforcement learning
- PMID: 20510862
- PMCID: PMC2895323
- DOI: 10.1016/j.neuron.2010.04.016
States versus rewards: dissociable neural prediction error signals underlying model-based and model-free reinforcement learning
Abstract
Reinforcement learning (RL) uses sequential experience with situations ("states") and outcomes to assess actions. Whereas model-free RL uses this experience directly, in the form of a reward prediction error (RPE), model-based RL uses it indirectly, building a model of the state transition and outcome structure of the environment, and evaluating actions by searching this model. A state prediction error (SPE) plays a central role, reporting discrepancies between the current model and the observed state transitions. Using functional magnetic resonance imaging in humans solving a probabilistic Markov decision task, we found the neural signature of an SPE in the intraparietal sulcus and lateral prefrontal cortex, in addition to the previously well-characterized RPE in the ventral striatum. This finding supports the existence of two unique forms of learning signal in humans, which may form the basis of distinct computational strategies for guiding behavior.
Copyright 2010 Elsevier Inc. All rights reserved.
Figures
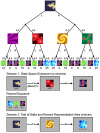
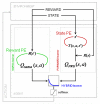
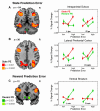
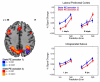
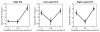
Comment in
- Nature. 2010 Jul 29;466(7306):535
Similar articles
-
The involvement of model-based but not model-free learning signals during observational reward learning in the absence of choice.J Neurophysiol. 2016 Jun 1;115(6):3195-203. doi: 10.1152/jn.00046.2016. Epub 2016 Apr 6. J Neurophysiol. 2016. PMID: 27052578 Free PMC article.
-
Beta Oscillations in Monkey Striatum Encode Reward Prediction Error Signals.J Neurosci. 2023 May 3;43(18):3339-3352. doi: 10.1523/JNEUROSCI.0952-22.2023. Epub 2023 Apr 4. J Neurosci. 2023. PMID: 37015808 Free PMC article.
-
Reinforcement learning signals in the human striatum distinguish learners from nonlearners during reward-based decision making.J Neurosci. 2007 Nov 21;27(47):12860-7. doi: 10.1523/JNEUROSCI.2496-07.2007. J Neurosci. 2007. PMID: 18032658 Free PMC article.
-
The ubiquity of model-based reinforcement learning.Curr Opin Neurobiol. 2012 Dec;22(6):1075-81. doi: 10.1016/j.conb.2012.08.003. Epub 2012 Sep 6. Curr Opin Neurobiol. 2012. PMID: 22959354 Free PMC article. Review.
-
Reward-dependent learning in neuronal networks for planning and decision making.Prog Brain Res. 2000;126:217-29. doi: 10.1016/S0079-6123(00)26016-0. Prog Brain Res. 2000. PMID: 11105649 Review.
Cited by
-
Navigating complex decision spaces: Problems and paradigms in sequential choice.Psychol Bull. 2014 Mar;140(2):466-86. doi: 10.1037/a0033455. Epub 2013 Jul 8. Psychol Bull. 2014. PMID: 23834192 Free PMC article. Review.
-
Integrating cortico-limbic-basal ganglia architectures for learning model-based and model-free navigation strategies.Front Behav Neurosci. 2012 Nov 27;6:79. doi: 10.3389/fnbeh.2012.00079. eCollection 2012. Front Behav Neurosci. 2012. PMID: 23205006 Free PMC article.
-
Fronto-striatal structures related with model-based control as an endophenotype for obsessive-compulsive disorder.Sci Rep. 2021 Jun 7;11(1):11951. doi: 10.1038/s41598-021-91179-2. Sci Rep. 2021. PMID: 34099768 Free PMC article.
-
Posttraumatic Stress Disorder and the Developing Adolescent Brain.Biol Psychiatry. 2021 Jan 15;89(2):144-151. doi: 10.1016/j.biopsych.2020.06.001. Epub 2020 Jun 10. Biol Psychiatry. 2021. PMID: 32709416 Free PMC article. Review.
-
Agency modulates the lateral and medial prefrontal cortex responses in belief-based decision making.PLoS One. 2013 Jun 6;8(6):e65274. doi: 10.1371/journal.pone.0065274. Print 2013. PLoS One. 2013. PMID: 23762332 Free PMC article.
References
Publication types
MeSH terms
Grants and funding
LinkOut - more resources
Full Text Sources
Other Literature Sources