Learning latent structure: carving nature at its joints
- PMID: 20227271
- PMCID: PMC2862793
- DOI: 10.1016/j.conb.2010.02.008
Learning latent structure: carving nature at its joints
Abstract
Reinforcement learning (RL) algorithms provide powerful explanations for simple learning and decision-making behaviors and the functions of their underlying neural substrates. Unfortunately, in real-world situations that involve many stimuli and actions, these algorithms learn pitifully slowly, exposing their inferiority in comparison to animal and human learning. Here we suggest that one reason for this discrepancy is that humans and animals take advantage of structure that is inherent in real-world tasks to simplify the learning problem. We survey an emerging literature on 'structure learning'--using experience to infer the structure of a task--and how this can be of service to RL, with an emphasis on structure in perception and action.
(c) 2010 Elsevier Ltd. All rights reserved.
Figures
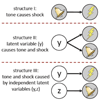
Similar articles
-
Computational advantages of reverberating loops for sensorimotor learning.Neural Comput. 2012 Mar;24(3):611-34. doi: 10.1162/NECO_a_00237. Epub 2011 Nov 17. Neural Comput. 2012. PMID: 22091669
-
Modeling of autonomous problem solving process by dynamic construction of task models in multiple tasks environment.Neural Netw. 2006 Oct;19(8):1169-80. doi: 10.1016/j.neunet.2006.05.037. Epub 2006 Sep 20. Neural Netw. 2006. PMID: 16989982
-
Neural Control of a Tracking Task via Attention-Gated Reinforcement Learning for Brain-Machine Interfaces.IEEE Trans Neural Syst Rehabil Eng. 2015 May;23(3):458-67. doi: 10.1109/TNSRE.2014.2341275. Epub 2014 Jul 24. IEEE Trans Neural Syst Rehabil Eng. 2015. PMID: 25073173
-
Circuit Mechanisms of Sensorimotor Learning.Neuron. 2016 Nov 23;92(4):705-721. doi: 10.1016/j.neuron.2016.10.029. Neuron. 2016. PMID: 27883902 Free PMC article. Review.
-
Goal-directed control and its antipodes.Neural Netw. 2009 Apr;22(3):213-9. doi: 10.1016/j.neunet.2009.03.004. Epub 2009 Mar 24. Neural Netw. 2009. PMID: 19362448 Review.
Cited by
-
Over the river, through the woods: cognitive maps in the hippocampus and orbitofrontal cortex.Nat Rev Neurosci. 2016 Aug;17(8):513-23. doi: 10.1038/nrn.2016.56. Epub 2016 Jun 3. Nat Rev Neurosci. 2016. PMID: 27256552 Free PMC article. Review.
-
Dynamic integration of conceptual information during learning.PLoS One. 2018 Nov 14;13(11):e0207357. doi: 10.1371/journal.pone.0207357. eCollection 2018. PLoS One. 2018. PMID: 30427917 Free PMC article.
-
Statistical context dictates the relationship between feedback-related EEG signals and learning.Elife. 2019 Aug 21;8:e46975. doi: 10.7554/eLife.46975. Elife. 2019. PMID: 31433294 Free PMC article.
-
Deterministic response strategies in a trial-and-error learning task.PLoS Comput Biol. 2018 Nov 29;14(11):e1006621. doi: 10.1371/journal.pcbi.1006621. eCollection 2018 Nov. PLoS Comput Biol. 2018. PMID: 30496285 Free PMC article.
-
Competitive Frontoparietal Interactions Mediate Implicit Inferences.J Neurosci. 2019 Jun 26;39(26):5183-5194. doi: 10.1523/JNEUROSCI.2551-18.2019. Epub 2019 Apr 23. J Neurosci. 2019. PMID: 31015338 Free PMC article.
References
-
- Sutton RS, Barto AG. Reinforcement Learning: An Introduction. MIT Press; 1998.
-
- Schultz W, Dayan P, Montague PR. A neural substrate of prediction and reward. Science. 1997;275(5306):1593. - PubMed
-
- Houk JC, Adams JL, Barto AG. A model of how the basal ganglia generate and use neural signals that predict reinforcement. Models of information processing in the basal ganglia. 1995:249–270.
-
-
Kemp C, Tenenbaum JB. Structured statistical models of inductive reasoning. Psychological review. 2009;116(1):20–58. An elegant demonstration of how structured knowledge influences human reasoning in a large variety of domains.
-
-
- Mach E. The analysis of sensations. 1897
Publication types
MeSH terms
Grants and funding
LinkOut - more resources
Full Text Sources