Everyone knows what is interesting: salient locations which should be fixated
- PMID: 20053088
- PMCID: PMC2915572
- DOI: 10.1167/9.11.25
Everyone knows what is interesting: salient locations which should be fixated
Abstract
Most natural scenes are too complex to be perceived instantaneously in their entirety. Observers therefore have to select parts of them and process these parts sequentially. We study how this selection and prioritization process is performed by humans at two different levels. One is the overt attention mechanism of saccadic eye movements in a free-viewing paradigm. The second is a conscious decision process in which we asked observers which points in a scene they considered the most interesting. We find in a very large participant population (more than one thousand) that observers largely agree on which points they consider interesting. Their selections are also correlated with the eye movement pattern of different subjects. Both are correlated with predictions of a purely bottom-up saliency map model. Thus, bottom-up saliency influences cognitive processes as far removed from the sensory periphery as in the conscious choice of what an observer considers interesting.
Figures
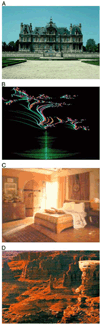
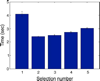
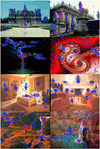
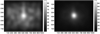
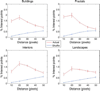
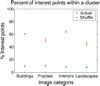
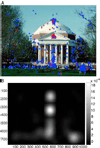
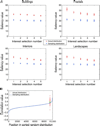
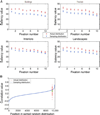
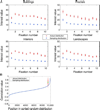
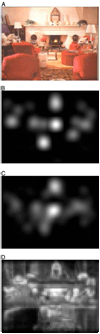
Similar articles
-
What stands out in a scene? A study of human explicit saliency judgment.Vision Res. 2013 Oct 18;91:62-77. doi: 10.1016/j.visres.2013.07.016. Epub 2013 Aug 15. Vision Res. 2013. PMID: 23954536
-
Real-world visual search is dominated by top-down guidance.Vision Res. 2006 Nov;46(24):4118-33. doi: 10.1016/j.visres.2006.08.008. Epub 2006 Sep 26. Vision Res. 2006. PMID: 17005231
-
Fixation and saliency during search of natural scenes: the case of visual agnosia.Neuropsychologia. 2009 Jul;47(8-9):1994-2003. doi: 10.1016/j.neuropsychologia.2009.03.013. Epub 2009 Mar 18. Neuropsychologia. 2009. PMID: 19428433
-
Viewing task influences eye movement control during active scene perception.J Vis. 2009 Mar 13;9(3):6.1-15. doi: 10.1167/9.3.6. J Vis. 2009. PMID: 19757945
-
Visual cognition.Vision Res. 2011 Jul 1;51(13):1538-51. doi: 10.1016/j.visres.2011.01.015. Epub 2011 Feb 15. Vision Res. 2011. PMID: 21329719 Free PMC article. Review.
Cited by
-
The Role of Stimuli-Driven and Goal-Driven Attention in Shopping Decision-Making Behaviors-An EEG and VR Study.Brain Sci. 2023 Jun 8;13(6):928. doi: 10.3390/brainsci13060928. Brain Sci. 2023. PMID: 37371406 Free PMC article.
-
Idiosyncratic viewing patterns of social scenes reflect individual preferences.J Vis. 2022 Dec 1;22(13):10. doi: 10.1167/jov.22.13.10. J Vis. 2022. PMID: 36583910 Free PMC article.
-
Predicting Goal-directed Human Attention Using Inverse Reinforcement Learning.Proc IEEE Comput Soc Conf Comput Vis Pattern Recognit. 2020 Jun;2020:190-199. doi: 10.1109/cvpr42600.2020.00027. Epub 2020 Aug 5. Proc IEEE Comput Soc Conf Comput Vis Pattern Recognit. 2020. PMID: 34163124 Free PMC article.
-
A Neuromorphic Proto-Object Based Dynamic Visual Saliency Model With a Hybrid FPGA Implementation.IEEE Trans Biomed Circuits Syst. 2021 Jun;15(3):580-594. doi: 10.1109/TBCAS.2021.3089622. Epub 2021 Aug 12. IEEE Trans Biomed Circuits Syst. 2021. PMID: 34133287 Free PMC article.
-
Salience-based object prioritization during active viewing of naturalistic scenes in young and older adults.Sci Rep. 2020 Dec 16;10(1):22057. doi: 10.1038/s41598-020-78203-7. Sci Rep. 2020. PMID: 33328485 Free PMC article.
References
-
- Bacon WF, Egeth HE. Overriding stimulus-driven attentional capture. Perception & Psychophysics. 1994;55:485–496. [PubMed] - PubMed
-
- Cerf M, Cleary DR, Peters RJ, Einhäuser W, Koch C. Observers are consistent when rating image conspicuity. Vision Research. 2007;47:3052–3060. [PubMed] - PubMed
-
- Craft E, Schütze H, Niebur E, von der Heydt R. A neural model of figure–ground organization. Journal of Neurophysiology. 2007;97:4310–4326. [PubMed] [Article] - PubMed
-
- Driver J, Baylis G. Edge-assignment and figure–ground segmentation in short-term visual matching. Cognitive Psychology. 1996;31:248–306. [PubMed] - PubMed
-
- Elazary L, Itti L. Interesting objects are visually salient. Journal of Vision. 2008;8(3):3. 1–15, http://journalofvision.org/8/3/3/, doi:10.1167/8.3.3. [PubMed] [Article] - PubMed
Publication types
MeSH terms
Grants and funding
LinkOut - more resources
Full Text Sources
Other Literature Sources