Generalized Bienenstock-Cooper-Munro rule for spiking neurons that maximizes information transmission
- PMID: 15795376
- PMCID: PMC555686
- DOI: 10.1073/pnas.0500495102
Generalized Bienenstock-Cooper-Munro rule for spiking neurons that maximizes information transmission
Abstract
Maximization of information transmission by a spiking-neuron model predicts changes of synaptic connections that depend on timing of pre- and postsynaptic spikes and on the postsynaptic membrane potential. Under the assumption of Poisson firing statistics, the synaptic update rule exhibits all of the features of the Bienenstock-Cooper-Munro rule, in particular, regimes of synaptic potentiation and depression separated by a sliding threshold. Moreover, the learning rule is also applicable to the more realistic case of neuron models with refractoriness, and is sensitive to correlations between input spikes, even in the absence of presynaptic rate modulation. The learning rule is found by maximizing the mutual information between presynaptic and postsynaptic spike trains under the constraint that the postsynaptic firing rate stays close to some target firing rate. An interpretation of the synaptic update rule in terms of homeostatic synaptic processes and spike-timing-dependent plasticity is discussed.
Figures
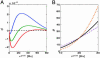
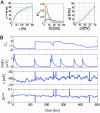
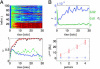
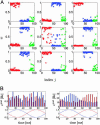
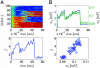
Similar articles
-
A triplet spike-timing-dependent plasticity model generalizes the Bienenstock-Cooper-Munro rule to higher-order spatiotemporal correlations.Proc Natl Acad Sci U S A. 2011 Nov 29;108(48):19383-8. doi: 10.1073/pnas.1105933108. Epub 2011 Nov 11. Proc Natl Acad Sci U S A. 2011. PMID: 22080608 Free PMC article.
-
Synaptic and temporal ensemble interpretation of spike-timing-dependent plasticity.Neural Comput. 2005 Nov;17(11):2316-36. doi: 10.1162/0899766054796879. Neural Comput. 2005. PMID: 16156931
-
Triplets of spikes in a model of spike timing-dependent plasticity.J Neurosci. 2006 Sep 20;26(38):9673-82. doi: 10.1523/JNEUROSCI.1425-06.2006. J Neurosci. 2006. PMID: 16988038 Free PMC article.
-
Spike timing-dependent plasticity: a Hebbian learning rule.Annu Rev Neurosci. 2008;31:25-46. doi: 10.1146/annurev.neuro.31.060407.125639. Annu Rev Neurosci. 2008. PMID: 18275283 Review.
-
Coding properties of spiking neurons: reverse and cross-correlations.Neural Netw. 2001 Jul-Sep;14(6-7):599-610. doi: 10.1016/s0893-6080(01)00053-3. Neural Netw. 2001. PMID: 11665756 Review.
Cited by
-
Spatio-Temporally Efficient Coding Assigns Functions to Hierarchical Structures of the Visual System.Front Comput Neurosci. 2022 May 27;16:890447. doi: 10.3389/fncom.2022.890447. eCollection 2022. Front Comput Neurosci. 2022. PMID: 35694611 Free PMC article.
-
Homeostatic Plasticity and STDP: Keeping a Neuron's Cool in a Fluctuating World.Front Synaptic Neurosci. 2010 Jun 7;2:5. doi: 10.3389/fnsyn.2010.00005. eCollection 2010. Front Synaptic Neurosci. 2010. PMID: 21423491 Free PMC article.
-
Phenomenological models of synaptic plasticity based on spike timing.Biol Cybern. 2008 Jun;98(6):459-78. doi: 10.1007/s00422-008-0233-1. Epub 2008 May 20. Biol Cybern. 2008. PMID: 18491160 Free PMC article. Review.
-
Behavior control in the sensorimotor loop with short-term synaptic dynamics induced by self-regulating neurons.Front Neurorobot. 2014 May 23;8:19. doi: 10.3389/fnbot.2014.00019. eCollection 2014. Front Neurorobot. 2014. PMID: 24904403 Free PMC article.
-
Learning as filtering: Implications for spike-based plasticity.PLoS Comput Biol. 2022 Feb 23;18(2):e1009721. doi: 10.1371/journal.pcbi.1009721. eCollection 2022 Feb. PLoS Comput Biol. 2022. PMID: 35196324 Free PMC article.
References
Publication types
MeSH terms
LinkOut - more resources
Full Text Sources
Other Literature Sources